

PASS
source link: https://www.robots.ox.ac.uk/~vgg/research/pass/
Go to the source link to view the article. You can view the picture content, updated content and better typesetting reading experience. If the link is broken, please click the button below to view the snapshot at that time.
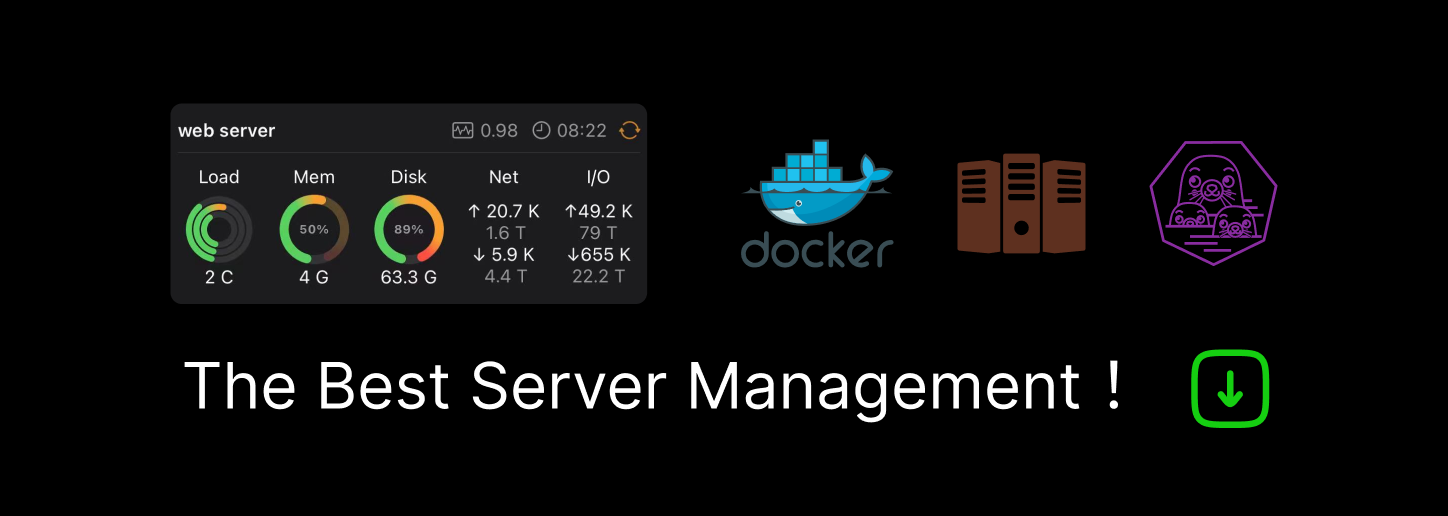
PASS is a large-scale image dataset that does not include any humans and which can be used for high-quality pretraining while significantly reducing privacy concerns.
Humans
Our dataset does not include any identifiable humans. This protects the privacy of the creators of the images.
images
PASS contains 1,440,191 images not containing humans or personally identifiable information.
licence files
The whole dataset only contains CC-BY licensed images with full attribution information.


The dataset is geographically diverse and almost a third of images contain geo-location.
Download
To download PASS, we provide a link to our file on zenodo.
The page includes a link to download the images and the licenses/creator file. For the images, simply download the tars, concatenate and extract it.
Or manually download the files from the list of urls, e.g. like this.
Find pretrained models here.
PASS dataset
Code and Models
Pretrained models and code for running evaluations as in the paper.
License
The PASS dataset is available to download for commercial/research purposes under a Creative Commons Attribution 4.0 International License. The copyright remains with the original owners of the video. A complete version of the license can be found here.
Please contact the authors below if you have any queries regarding the dataset.
Publications
Please cite the following if you make use of the dataset.
@Article{asano21pass,
author = "Yuki M. Asano and Christian Rupprecht and Andrew Zisserman and Andrea Vedaldi",
title = "PASS: An ImageNet replacement for self-supervised pretraining without humans",
journal = "NeurIPS Track on Datasets and Benchmarks",
year = "2021"
}
Acknowledgements
We are thankful to Abhishek Dutta and Ashish Thandavan for their great support. We thank Rajan and his team of annotators at Elancer for their precise work. We are grateful for support from the AWS Machine Learning Research Awards (MLRA), EPSRC Centre for Doctoral Training in Autonomous Intelligent Machines & Systems [EP/L015897/1], the Qualcomm Innovation Fellowship, a Royal Society Research Professorship, and the EPSRC Programme Grant VisualAI EP/T028572/1. C. R. is supported by Innovate UK (project 71653) on behalf of UK Research and Innovation (UKRI) and by the European Research Council (ERC) IDIU-638009.
Recommend
About Joyk
Aggregate valuable and interesting links.
Joyk means Joy of geeK