

使用Lucene.Net实现全文检索
source link: https://www.cnblogs.com/MeteorSeed/archive/2012/12/24/2703716.html
Go to the source link to view the article. You can view the picture content, updated content and better typesetting reading experience. If the link is broken, please click the button below to view the snapshot at that time.
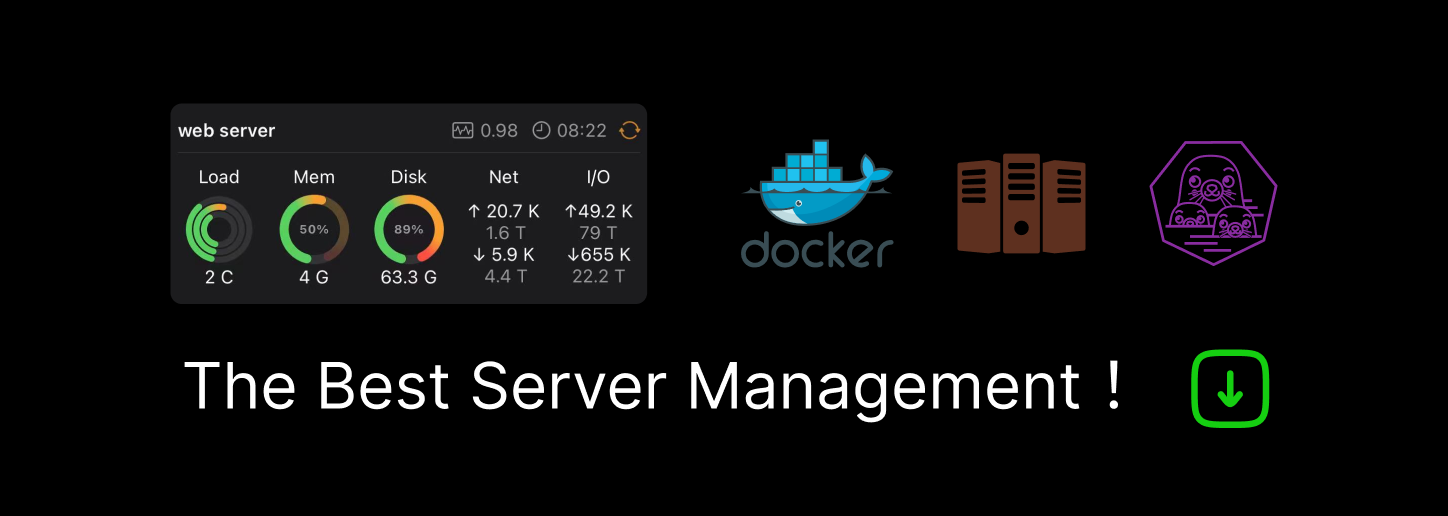
使用Lucene.Net实现全文检索
一 Lucene.Net概述
Lucene.Net是一个C#开发的开源全文索引库,其源码包括“核心”与“外围”两部分。外围部分实现辅助功能,而核心部分包括:
- Lucene.Net.Index 提供索引管理,词组排序。
- Lucene.Net.Search 提供查询相关功能。
- Lucene.Net.Store 支持数据存储管理,主要包括I/O操作。
- Lucene.Net.Util 公共类。
- Lucene.Net.Documents 负责描述索引存储时的文件结构管理。
- Lucene.Net.QueryParsers 提供查询语法。
- Lucene.Net.Analysis 负责分析文本。
全文检索流程如下:
一个简单的全文检索实例:
创建索引:
关键代码形如:
static void createIndex(string title, string content) { LN.Analysis.Analyzer analyzer = new LN.Analysis.Standard.StandardAnalyzer(); LN.Index.IndexWriter iw = new LN.Index.IndexWriter("Index", analyzer, false); LN.Documents.Document document = new LN.Documents.Document(); document.Add(new LN.Documents.Field("title", title, LN.Documents.Field.Store.YES, LN.Documents.Field.Index.TOKENIZED)); document.Add(new LN.Documents.Field("content", content, LN.Documents.Field.Store.YES, LN.Documents.Field.Index.TOKENIZED)); iw.AddDocument(document); iw.Optimize(); iw.Close(); }
关键代码形如:
static List<Item> search(string keyWord) { List<Item> results = new List<Item>(); LN.Analysis.Analyzer analyzer = new LN.Analysis.Standard.StandardAnalyzer(); LN.Search.IndexSearcher searcher = new LN.Search.IndexSearcher("Index"); LN.QueryParsers.MultiFieldQueryParser parser = new LN.QueryParsers.MultiFieldQueryParser(new string[] { "title", "content" }, analyzer); LN.Search.Query query = parser.Parse(keyWord); LN.Search.Hits hits = searcher.Search(query); for (int i = 0; i < hits.Length(); i++) { LN.Documents.Document doc = hits.Doc(i); results.Add(new Item() { Title = doc.Get("title"), Content = doc.Get("content") }); } searcher.Close(); return results; }
二 分词
(一)内置分词器
分词(切词)是实现全文检索的基础,之所以我们能够让机器理解我们的自然语言,是因为有了分词的帮助。分词工作由Analyzer类完成,它负责把文本切成Token序列,Token就是索引中的单词。Lucene.Net在两个地方用到分词:创建文档索引和分析搜索关键字。其过程示意如下:
由此可知,在创建索引和搜索时,必须使用同样的分词器,保证其切出相同的Token才能检索到结果。(Lucene.Net把查询关键字中的单词叫做“Term”,Term和Token的文本是一样的,只是某些属性不一样。)
Lucene.Net实现了一些分词器,其对英文支持较好,但是对中文支持欠佳。
针对内置分词器测试结果如下:
关键代码形如:
private static List<string> cutWords(string words, Analyzer analyzer) { List<string> results = new List<string>(); TokenStream ts = analyzer.ReusableTokenStream("", new StringReader(words)); Token token; while ((token = ts.Next()) != null) { results.Add(token.TermText()); } ts.Close(); return results; }
可见,除了StandardAnalyzer外,其它分词器对中文基本无法处理,需要用户自行解决。
(二)分词过程
分词实际是由以下类型完成:
查看WhitespaceAnalyzer的部分源码如下:
public sealed class WhitespaceAnalyzer:Analyzer { public override TokenStream TokenStream(System.String fieldName, System.IO.TextReader reader) { return new WhitespaceTokenizer(reader); } ... }
由此可见,WhitespaceAnalyzer的工作都是交给WhitespaceTokenizer来完成的,并且没有使用筛选器,这也与之前测试的结果相符。我们可以利用TokenStream的派生类型来实现自定义分词器。 例如修改上述代码来得到一个新的分词器,功能类似WhitespaceAnalyzer,不同的是将大写字母变为小写,其代码形如:
public sealed class NewWhitespaceAnalyzer:Analyzer { public override TokenStream TokenStream(System.String fieldName, System.IO.TextReader reader) { TokenStream ts = new WhitespaceTokenizer(reader); return new LowerCaseFilter(ts); } ... }
(三)中文分词
显然,用户可以自定义分词器,来实现中文分词。但是,大多数用户不熟悉中文分词算法,同时也没有时间和精力来实现自定义分词,毕竟分词并不是我们系统的核心功能。因此,笔者引用了另一个中文分词组件——盘古分词。测试结果如下:
盘古分词使用步骤如下:
Setp 1:添加相关程序集引用
这里需要添加2个程序集,PanGu.dll(盘古分词的核心组件)和PanGu.Lucene.Analyzer.dll(盘古分词的Lucene组件)。
Step 2:添加中文分词库
Step 3:添加并设置配置文件
Step 4:在Lucene.Net使用盘古分词
PanGu.Lucene.Analyzer.dll中定义了Analyzer的派生类型Lucene.Net.Analysis.PanGu.PanGuAnalyzer,与Tokenizer的派生类Lucene.Net.Analysis.PanGu.PanGuTokenizer,语法与Lucene.Net内置分词器相同。
Step 5:维护分词库
使用DictManage.exe管理和维护词库:
三 索引
(一)索引的存储结构
为了方便索引大量文档,Lucene.Net中的一个索引包括多个子索引,叫做Segment(段)。每个Segment包括多个可搜索的文档,叫做Document;每个Document包括多个Field;每个Field又包括多个Term。综上所述,Lucene.Net的索引文件的逻辑结构如下:
索引文件的物理表示如下:
Lucene.Net把一个文档写入索引时,首先生成这个文档的到排索引,然后再把文档的倒排索引合并到段的倒排索引中。
(二)常用类型
- Directory Lucene.Net的Directory类型实现索引的存储。常用类型继承树如下:
- IndexWriter 负责将索引写入Directory。Lucene通过设置缓存来提供写索引的速度,IndexWriter有几个参数来调整缓存的大小,控制Segment的数量,以及写索引的频率:
- 合并因子(mergeFactor) 这个参数决定一个索引块中可以存放多少文档(Document)以及把磁盘上的索引段(Segment)合并成一个大索引段的频率。该参数默认值为10。在默认情况下,缓存中Document数达到10时,所有的文档将写入一个新的Segment。并且,如果Directory的Segment的个数达到10,这10个索引块会被合并成一个新的Segment。对于大量文档来说,这个值大一些会更好。可以通过“SetMergeFactor(int mergeFactor)”方法来设置、
- 最小合并文档数(minMergeDocs)、最大缓存文档数(maxBufferedDocs) 默认值为10,它决定缓存中Document数量达到多少才能将他们写入磁盘。该值越大越消耗内存,I/O操作越少。(本处,笔者也有些糊涂,笔者感觉两者类似,不知道具体区别,若理解有误还请读者赐教。)
- 最大合并文档数(maxMergeDocs) 默认值为Integer.MAX_VALUE,它决定一个索引段(Segment)中的最大文档(Document)数。该值越大越高效,因为默认值以及很大了所以不用改变。
- 最大域长度(maxFieldLength) 默认值10000,表示截取该域中的前10000个Term,前10000个以外的Term将不被索引和检索。该值可在索引中随时更改,并即时生效(仅对之后的操作生效,一般该值设置为Integer.MAX_VALUE)。
IndexWriter的常用方法包括:
- Flush/Commit Flush方法与Commit方法相同,都是把缓存中的数据提交,可以清除缓存。
- Close 无论是否发生异常都必须调用Close方法,该方法将对文件进行解锁,并完成Flush方法的功能。
- Optimize Optimize方法用于优化索引,执行相当耗时。
- Document 包含了可索引文档的信息。每个Document都有一个编号,但该编号并非永远不变。
- Field 类似实体的某个属性,就像数据库中的一个列,其成员如下:
(可以看到,Index的某些字段我给出的相同的注释,这是因为向下兼容的目的而具有相同的作用。注:高亮显示将用的TermVector。)
常用列选项组合及用法如下:
Index Store TermVector 用法 NOT_ANSLYZED YES NO 文件名、主键 ANSLYZED YES WITH_POSITUION_OFFSETS 标题、摘要 ANSLYZED NO WITH_POSITUION_OFFSETS 很长的全文 NO YES NO 文档类型 NOT_ANSLYZED NO NO 隐藏的关键词(三)创建索引
创建索引流程如下:
1 基本实现
其代码形如:
private static void saveIndex(string dirPath, string uri, string title, string summary) { //判断是创建索引还是追加索引 bool isNew = false; if (!LN.Index.IndexReader.IndexExists(dirPath)) { isNew = true; } LN.Index.IndexWriter iw = new LN.Index.IndexWriter(dirPath, new PanGuAnalyzer(), isNew);//使用PanGuAnalyzer初始化IndexWriter,参数create为true表示创建,为false表示添加。 LN.Documents.Document document = new LN.Documents.Document();//创建Document //添加Field document.Add(new LN.Documents.Field("Uri", uri, LN.Documents.Field.Store.YES, LN.Documents.Field.Index.NO)); document.Add(new LN.Documents.Field("Title", title, LN.Documents.Field.Store.YES, LN.Documents.Field.Index.ANALYZED)); document.Add(new LN.Documents.Field("CreateTime", DateTime.Now.ToString("yyyy-MM-dd"), LN.Documents.Field.Store.YES, LN.Documents.Field.Index.NOT_ANALYZED)); document.Add(new LN.Documents.Field("Summary", summary, LN.Documents.Field.Store.YES, LN.Documents.Field.Index.ANALYZED)); iw.AddDocument(document);//向索引添加文档 iw.Optimize();//优化索引 iw.Close();//关闭索引 }
2 权重Boost
默认情况下,搜索结果以Document.Score作为排序依据,该数值越大排名越靠前。Score与Boost成正比,满分是1,如果某的Document的Score为1,其它文档将根据该文档进行评分,因此不会同时存在多个同时为1的情况。从公式也可看出,Boost不能为0,Boost=0则Score为0。在类型Lucene.Net.Search.Hits这定义了Score(int)方法,能够获取评分。
Boost的默认值为1,通过改变权重我们可以影响查询结果。其代码形如:
“document.SetBoost(2F);”改变Document的权重,将影响所有Field的搜索得分。
“document.GetField("FieldName").SetBoost(2F);”只改变某个Field的权重。
boost的数值存储在Norms中,因此要注意Index的设置,设置NO_NORMS将节省索引空间,但是将不支持权重。
权重的调整建议:
- 标题权重一般比内容高 标题更能够非常准确地描述文档的内容,而且长度比较短,提高权重不会造成严重的影响。
- 不要把包含大量索引的文档的权重设置过高 文档中能索引的词越多,对搜索的影响越大,例如在搜索如“好的”这样常用的词汇时,这篇文章也将位列榜首,但并不是我们需要的。
- 如果能靠设置Field的权重来解决,就不要设置Document的权重 原因与上面的类似,当我们要改变某些关键字的搜索结果时,要尽量减少对其它关键字搜索的影响。
- 考虑降低权重 对于某些没有意义的文档,考虑降低权重来为相对提升其它文档的搜索排位。
(四)合并索引
其代码形如:
private static void mergeIndex(string sourcePath, string targetPath) { LN.Store.Directory sourceDir = LN.Store.FSDirectory.GetDirectory(sourcePath, false); LN.Store.Directory targetDir = LN.Store.FSDirectory.GetDirectory(targetPath, false); LN.Index.IndexWriter iw = new LN.Index.IndexWriter(targetPath, new PanGuAnalyzer(), false); iw.AddIndexes(new LN.Store.Directory[] { sourceDir }); iw.Optimize(); iw.Close(); }
合并索引功能常用于将内存中的Directory合并到硬盘的Directory中。(通常我们使用这种方法来优化索引创建过程。)
(五)删除索引
IndexReader,IndexModifer,IndexWriter都提供了DeleteDocuements、DeleteDocument、DeleteAll方法常来删除索引。因为Document的编号会改变,使用一般不会持久化到数据库中,所以多数情况下会按指定的Term来删除索引。其代码形如:
private static void delIndex(string dirPath, string key) { LN.Index.IndexWriter iw = new LN.Index.IndexWriter(dirPath, new PanGuAnalyzer(), false); iw.DeleteDocuments(new LN.Index.Term("Key", key));
iw.Optimize();//删除文件后并非从磁盘中移除,而是生成一个.del的文件,需要调用Optimize方法来清除。在清除文件前可以使用UndeleteAll方法恢复(笔者未尝试) iw.Close(); }
(需要注意的是,如果Field使用的是Index.NO,则表示不建立索引,当然也无法进行删除。)
(六)更新索引
更新索引时只允许更新整个Docuemnt,无法单独更新Docuemnt中的Field。其代码形如:
private static void updateIndex(string path, string key, LN.Documents.Document doc) { LN.Index.IndexWriter iw = new LN.Index.IndexWriter(path, new PanGuAnalyzer(), false); iw.UpdateDocument(new LN.Index.Term("Key", key), doc); iw.Optimize(); iw.Close(); }
(七)优化索引
通过IndexWriter的Optimize方法优化索引,以加快搜索的速度,该方法提供多个重载,其执行相当耗时,应谨慎使用。优化产生的垃圾文件,在执行Flush/Commit/Close方法后才会被物理删除。Optimize方法及其重载包括:
- Optimize() 合并段,完成后返回。
- Optimize(bool doWait) 与Optimize()相同,但立即返回。
- Optimize(int maxNumSegments) 针对最多maxNumSegments个段进行优化,而并非全部索引。
- Optimize(int maxNumSegments, bool doWait) 与Optimize(int maxNumSegments)相同,但立即返回。
(优化索引实际就是在压缩索引文件,需要大约2倍索引大小的临时空间,且特别耗时。一种好的做法是把内存中的索引合并到应硬盘中。)
四 搜索
(一)基本查询
private static List<Item> search(string dirPath, string keywords) { List<Item> results = new List<Item>(); LN.Search.IndexSearcher searcher = new LN.Search.IndexSearcher(dirPath);//初始化IndexSearcher LN.QueryParsers.MultiFieldQueryParser parser = new LN.QueryParsers.MultiFieldQueryParser(new string[] { "Title", "Summary" }, new PanGuAnalyzer());//初始化MultiFieldQueryParser以便同时查询多列 LN.Search.Query query = parser.Parse(keywords);//初始化Query LN.Search.Hits hits = searcher.Search(query);//搜索 //遍历结果集 for (int i = 0; i < hits.Length(); i++) { LN.Documents.Document doc = hits.Doc(i); results.Add(new Item() { Title = doc.Get("Title"), Summary = doc.Get("Summary"), CreateTime = doc.Get("CreateTime"), Uri = doc.Get("Uri") }); } searcher.Close(); return results; }
以上代码显示了一个基本搜索的例子。搜索的基本过程包括:查询请求解析->搜索->获取匹配的结果集->提取所需数据。搜索主要做两件事情:首先,确定那些文档出现在结果集中;然后,为结果集中的文档打分,分高的排在前面。
Lucene.Net采用向量空间搜索模型,在向量空间中越接近的文档越相似。向量空间搜索模型比较复杂(详细内容可以参考维基百科),其大致影响因素包括:
- 与关键字在文档中出现的频率成正比
- 与权重成正比
- 与反转文档频率成正比 该值主要受文档总数和包含关键字的文档数量影响,与文档总数成正比,与包含关键字的文档总数成反比,即索引库中文档越多,包含此关键字的文档越少,反转文档频率越高。
- 与保有率成正比 保有率主要受到关键字在Fleld中出现的次数(词频)和Field的长度(Field包含的词数)影响,与词频成正比,与Field的长度成反比,即从越短的Field中搜索出越多的关键字,我们就认为保有率高。
(二)常用类型
- IndexReader与IndexSearcher IndexReader能够读取索引,IndexSearcher是IndexReader的包装类型,负责搜索。
- Query及其常用派生类 这组类型用于实现各类查询,常用类型的继承树如下:
- QueryPaser及其常用派生类 该组类型用于分析用户的输入,并将其转换为Query实例,常用类型的继承树如下:
- Sort与SortField Sort类型是负责对搜索结果进行排序,可以针对指定的一个或多个域进行排序。SortField用于指定列类型,常用的列类型如下:
- Filter 这组类型实现对搜索的过滤。常用类型的继承树如下:
- TermFregVector TermFregVector包含了指定文档的项和词频信息,当在索引期间存储项向量的时候,才能通过IndexReader检索出TermFregVector。
- Hits Hits用于承载搜索的结果集。
(三)逻辑查询
查询同时包含多个Term的代码形如:
private static List<Item> andTermSearch(string dirPath, string[] keywords) { List<Item> results = new List<Item>(); LN.Search.IndexSearcher searcher = new LN.Search.IndexSearcher(dirPath);//初始化IndexSearcher LN.Search.BooleanQuery bq = new Lucene.Net.Search.BooleanQuery(); foreach (var item in keywords) { LN.Index.Term term = new Lucene.Net.Index.Term("Title", item); LN.Search.TermQuery tq = new Lucene.Net.Search.TermQuery(term); bq.Add(tq, LN.Search.BooleanClause.Occur.MUST); } LN.Search.Hits hits = searcher.Search(bq);//搜索 //遍历结果集 for (int i = 0; i < hits.Length(); i++) { LN.Documents.Document doc = hits.Doc(i); results.Add(new Item() { Title = doc.Get("Title"), Summary = doc.Get("Summary"), CreateTime = doc.Get("CreateTime"), Uri = doc.Get("Uri") }); } searcher.Close(); return results; }
上述代码的核心是“bq.Add(tq, LN.Search.BooleanClause.Occur.MUST);”,Occur的取值及含义如下:
通过改变该值,实现“与”、“或”、“非”操作。“非”操作常常与全匹配查询联合使用,以达到查询不满足某个条件的结果。其代码形如:
LN.Search.MatchAllDocsQuery madq = new Lucene.Net.Search.MatchAllDocsQuery();//匹配所有文档 ... bq.Add(tq, LN.Search.BooleanClause.Occur.MUST_NOT); bq.Add(madq, LN.Search.BooleanClause.Occur.MUST); ...
BooleanQuery的成员SetMinimumNumberShouldMatch(int min),可以设置需要匹配上的条件的最小数量。
(四)复合查询
有2种方式实现复合查询,第一种方式是使用MultiFieldQueryParser,该方式已经在之前的代码中给出,还可以使用BooleanQuery,其代码形如:
private static List<Item> search(string dirPath, string keywords) { List<Item> results = new List<Item>(); LN.Search.IndexSearcher searcher = new LN.Search.IndexSearcher(dirPath);//初始化IndexSearcher LN.Search.BooleanQuery bq = new Lucene.Net.Search.BooleanQuery(); LN.Search.Query qTitle = new Lucene.Net.Search.TermQuery(new Lucene.Net.Index.Term("Title", keywords)); LN.Search.Query qSummary = new Lucene.Net.Search.TermQuery(new Lucene.Net.Index.Term("Summary", keywords)); bq.Add(qTitle, LN.Search.BooleanClause.Occur.SHOULD); bq.Add(qSummary, LN.Search.BooleanClause.Occur.SHOULD); LN.Search.Hits hits = searcher.Search(bq);//搜索 //遍历结果集 for (int i = 0; i < hits.Length(); i++) { LN.Documents.Document doc = hits.Doc(i); results.Add(new Item() { Title = doc.Get("Title"), Summary = doc.Get("Summary"), CreateTime = doc.Get("CreateTime"), Uri = doc.Get("Uri") }); } searcher.Close(); return results; }
也可以使用如下形式:
private static List<Item> search(string dirPath, string keywords) { List<Item> results = new List<Item>(); PanGuAnalyzer analyzer = new PanGuAnalyzer(); LN.Search.IndexSearcher searcher = new LN.Search.IndexSearcher(dirPath);//初始化IndexSearcher LN.Search.BooleanQuery bq = new Lucene.Net.Search.BooleanQuery(); LN.QueryParsers.QueryParser qpTitle = new Lucene.Net.QueryParsers.QueryParser("Title", analyzer); LN.QueryParsers.QueryParser qpSummary = new Lucene.Net.QueryParsers.QueryParser("Summary", analyzer); LN.Search.Query qTitle = qpTitle.Parse(keywords); LN.Search.Query qSummary = qpSummary.Parse(keywords); bq.Add(qTitle, LN.Search.BooleanClause.Occur.SHOULD); bq.Add(qSummary, LN.Search.BooleanClause.Occur.SHOULD); LN.Search.Hits hits = searcher.Search(bq);//搜索 //遍历结果集 for (int i = 0; i < hits.Length(); i++) { LN.Documents.Document doc = hits.Doc(i); results.Add(new Item() { Title = doc.Get("Title"), Summary = doc.Get("Summary"), CreateTime = doc.Get("CreateTime"), Uri = doc.Get("Uri") }); } searcher.Close(); return results; }
(五)跨度查询
对以下内容分词“I come from Beijing.”应用WhitespaceAnalyzer的结果为:“I\come\from\Beijing.”,其跨度如下:
跨度查询的代码形如:
private static List<Item> search(string dirPath, string keyword1,string keyword2) { List<Item> results = new List<Item>(); Analyzer analyzer = new WhitespaceAnalyzer(); LN.Search.IndexSearcher searcher = new LN.Search.IndexSearcher(dirPath);//初始化IndexSearcher LN.Search.Spans.SpanNearQuery snp = new Lucene.Net.Search.Spans.SpanNearQuery(new Lucene.Net.Search.Spans.SpanQuery[]{ new LN.Search.Spans.SpanTermQuery(new Lucene.Net.Index.Term("Summary", keyword1)),//第一个关键字 new LN.Search.Spans.SpanTermQuery(new Lucene.Net.Index.Term("Summary", keyword2))},//第二个关键字 1,//1个跨度以内 true);//有序 LN.Search.Hits hits = searcher.Search(snp);//搜索 //遍历结果集 for (int i = 0; i < hits.Length(); i++) { LN.Documents.Document doc = hits.Doc(i); results.Add(new Item() { Title = doc.Get("Title"), Summary = doc.Get("Summary"), CreateTime = doc.Get("CreateTime"), Uri = doc.Get("Uri") }); } searcher.Close(); return results; }
更具上述代码,如果传入“I”,“Beijing.”,则无法查询到,因为我在上例中将最大跨度设置为1,而实际跨度为2。可见,利用跨度查询,可以帮助筛选部分查询结果,时候查询那些关系紧密的关键字。
从之前的类图可以看到,跨度查询还有几个常用派生类型,其功能如下:
- SpanFirstQuery 限定只查询前面几个词。
- SpanRegexQuery 支持正则表达式。
- SpanNotQuery 包含必须满足的SpanQuery和必须排除的SpanQuery,例如查找包含“First”,但不包含“The”在前面的内容。
- SpanOrQuery 并操作。
(六)通配符查找
WildcardQuery支持通配符搜索,其中“*”表示多个字符,“?”表示1个字符。其代码形如:WildcardQuery query = new WildcardQuery(new Lucene.Net.Index.Term("Summary", keyword));//keyword="?o*"。
(七)排序
Lucene.Net主要有两种方式来控制排序结果,包括使用Sort对象定制排序和影响相关度计算结果。
1 按单列排序
代码形如:
Sort sort = new Sort(); SortField sf = new SortField("CreateTime", SortField.STRING, true);//true表示逆序 sort.SetSort(sf); Search.Hits hits = searcher.Search(query, sort);//搜索
2 按多列排序
代码形如:
Sort sort = new Sort(); SortField sf1 = new SortField("CreateTime", SortField.STRING, true); SortField sf2 = new SortField("Title", SortField.SCORE, true); sort.SetSort(new SortField[] { sf1, sf2 }); Search.Hits hits = searcher.Search(query, sort);//搜索
3 自定义排序
自定义排序功能,需要定义FieldComparatorSource的派生类型,还需要定义自己的比较器,要求其继承FieldComparator。然后重写FieldComparatorSource的NewComparator方法,并在其中返回自己定义的比较器实例。具体实例,可以参照Lucene.Net源码中的实现。
4 使用查询函数排序
查询函数将索引中的字符通过某个方法转变为数值,并作为评分来影响查询结果。
FieldScoreQuery fsq = new FieldScoreQuery("Uri", FieldScoreQuery.Type.INT);//将Uri列解释为Int CustomScoreQuery csq = new CustomScoreQuery(query, fsq);//合并最初的查询分值与当前分值 TopDocs td = searcher.Search(csq, 10);//搜索 //遍历结果集 for (int i = 0; i < td.totalHits; i++) { LN.Documents.Document doc = searcher.Doc(td.scoreDocs[i].doc); results.Add(new Item() { Title = doc.Get("Title"), Summary = doc.Get("Summary"), CreateTime = doc.Get("CreateTime"), Uri = doc.Get("Uri") }); }
可以定义自己的评分方法,具体用法可以参考FieldScoreQuery的源码。
5 设置权重
权重已在上文给出,不在赘述。
(八)过滤
使用Filter及其派生类完成对结果集的过滤,也可以定义自己的过滤器。使用过滤的代码形如:
RangeFilter filter = new RangeFilter("CreateTime", "19990101", "29991010", true, true); Search.Hits hits = searcher.Search(query, filter,sort);//搜索
何时可以使用过滤:
- 根据不同的安全权限显示搜索结果,即查询某个范围内的数据时可以借助过滤器。
- 希望缓存结果时,可以使用支持缓存的过滤器。
五 实践中的问题
本节之前的实例代码,仅针对Lucene.Net的使用进行了梳理,之前实例中的代码并不标准,在实际使用时还需要注意很多问题。本节将对这些问题进行探讨,以帮助读者开发高性能程序。
(一)缓存
Lucene.Net支持对查询(FieldCache)和排序结果(CachingWrapperFilter)进行缓存。每个IndexSearcher或者IndexReader都有自己的缓存,缓存的生命周期与IndexSearcher或者IndexReader的实例相同。CachingWrapperFilter针对每个Filter有一个缓存,除此之外还有其他支持缓存的筛选器。为了使缓存利用率最高,推荐使用单例模式来维护一个IndexSearcher实例。
(二)锁
Lucene.Net借助锁来应对并发问题。其索引访问原则如下:
- 在同一时刻,可以执行多个读操作(检索)。
- 在同一时刻,只能执行一个写操作(创建、优化、修改、删除)。
- 在执行写操作的同时可以同时执行多个读操作。
可见,在执行写操作时,索引文件会被加锁,以防止其他线程在同一时刻修改索引。加锁实际上是在索引目录下,产生一个锁文件,Lucene.Net一共有两种锁文件——commit.lock、write.lock。查询网上的一些资料发现两者的主要区别:
commit.lock主要与segment合并和读取的操作相关。例如,其出现在IndexWriter的初始化时,当segment的信息被读取完毕,它就会被释放。另外,当调用IndexWriter的AddIndexs()或MergeSegment()方法时,也会生成这个锁。
writer.lock出现在向索引添加文档时,或是将文档从索引中删除时。writer.lock会在IndexWriter被初始化时创建,然后会在调用IndexWriter的Close()方法时被释放。另外,会在IndexReader使用Delete方法删除文档时创建,并在调用IndexReader的Close()方法时被释放。
为了能够在出现异常时,得体得处理,最好报Close()放到finally快中。
注意,使用IndexModifier可以简化开发,IndexModifier对象封装了IndexWriter和IndexReader的常用操作,其内部实现了多线程同步锁定。使用 IndexModifier可避免同时使用 IndexWriter和IndexReader时需要在多个对象之间进行同步的麻烦。
(三)使用内存目录以及多线程查询器提高查询速度
对内存操作要比硬盘快的多,因此可以利用RAMDirectory来提高查询速度。设计思路为:在RAMDirectory中创建索引,查询时同时查询RAMDirectory与FSDirectory中的索引,并在合适的时候将RAMDirectory中的索引写入FSDirectory。
查询时使用ParallelMultiSearcher加快搜索速度。ParallelMultiSearcher为多线程版本的搜索器,查询内存与硬盘上的索引。
(四)总是设置权重
一般情况下,最好不要使用默认权重,原因很简单,标题中的关键字或者论文关键字栏目中的关键字,具有更高的价值,为了提高命中率,我们应该在创建索引时就有意地提高这些类型的权重。
主要参考文献:
《盘古分词使用手册》
《使用C#开发搜索引擎》
Recommend
About Joyk
Aggregate valuable and interesting links.
Joyk means Joy of geeK