
1

Bridging empirical-theoretical gap in neural network formal language learning
source link: https://arxiv.org/abs/2402.10013
Go to the source link to view the article. You can view the picture content, updated content and better typesetting reading experience. If the link is broken, please click the button below to view the snapshot at that time.
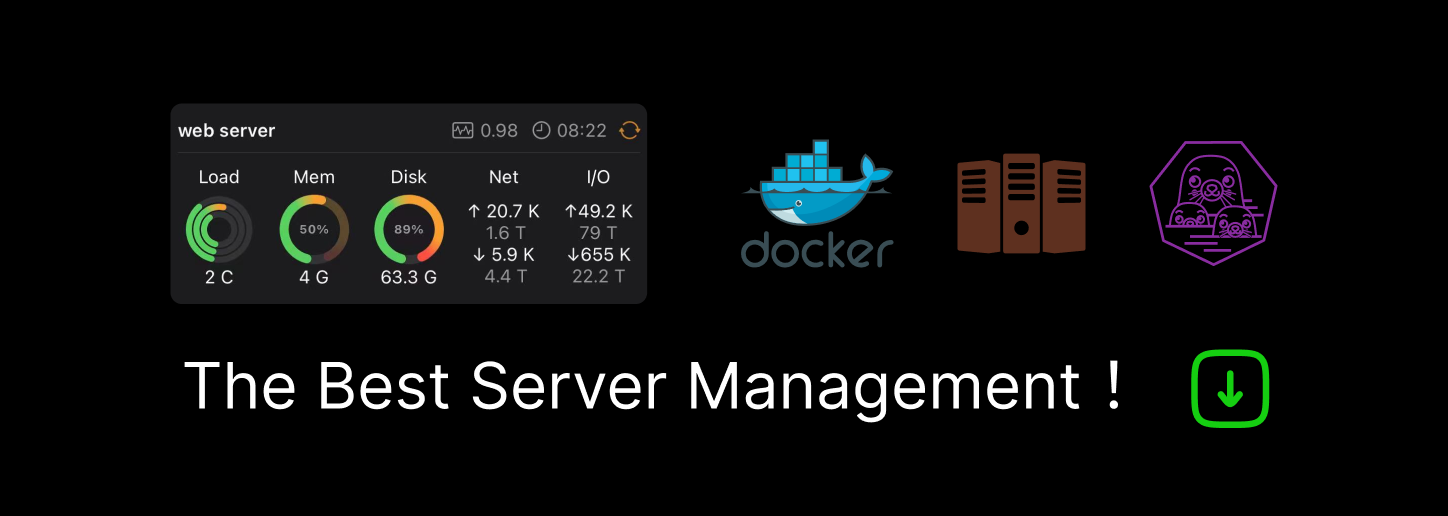
Computer Science > Computation and Language
[Submitted on 15 Feb 2024]
Bridging the Empirical-Theoretical Gap in Neural Network Formal Language Learning Using Minimum Description Length
Neural networks offer good approximation to many tasks but consistently fail to reach perfect generalization, even when theoretical work shows that such perfect solutions can be expressed by certain architectures. Using the task of formal language learning, we focus on one simple formal language and show that the theoretically correct solution is in fact not an optimum of commonly used objectives -- even with regularization techniques that according to common wisdom should lead to simple weights and good generalization (L1, L2) or other meta-heuristics (early-stopping, dropout). However, replacing standard targets with the Minimum Description Length objective (MDL) results in the correct solution being an optimum.
Comments: | 9 pages, 5 figures, 3 appendix pages |
Subjects: | Computation and Language (cs.CL); Formal Languages and Automata Theory (cs.FL) |
Cite as: | arXiv:2402.10013 [cs.CL] |
(or arXiv:2402.10013v1 [cs.CL] for this version) | |
https://doi.org/10.48550/arXiv.2402.10013 |
Recommend
About Joyk
Aggregate valuable and interesting links.
Joyk means Joy of geeK