

Powering GenAI with Enterprise Retrieval Augmented Generation (RAG)
source link: https://www.gigaspaces.com/blog/genai-enterprise-retrieval-augmented-generation
Go to the source link to view the article. You can view the picture content, updated content and better typesetting reading experience. If the link is broken, please click the button below to view the snapshot at that time.
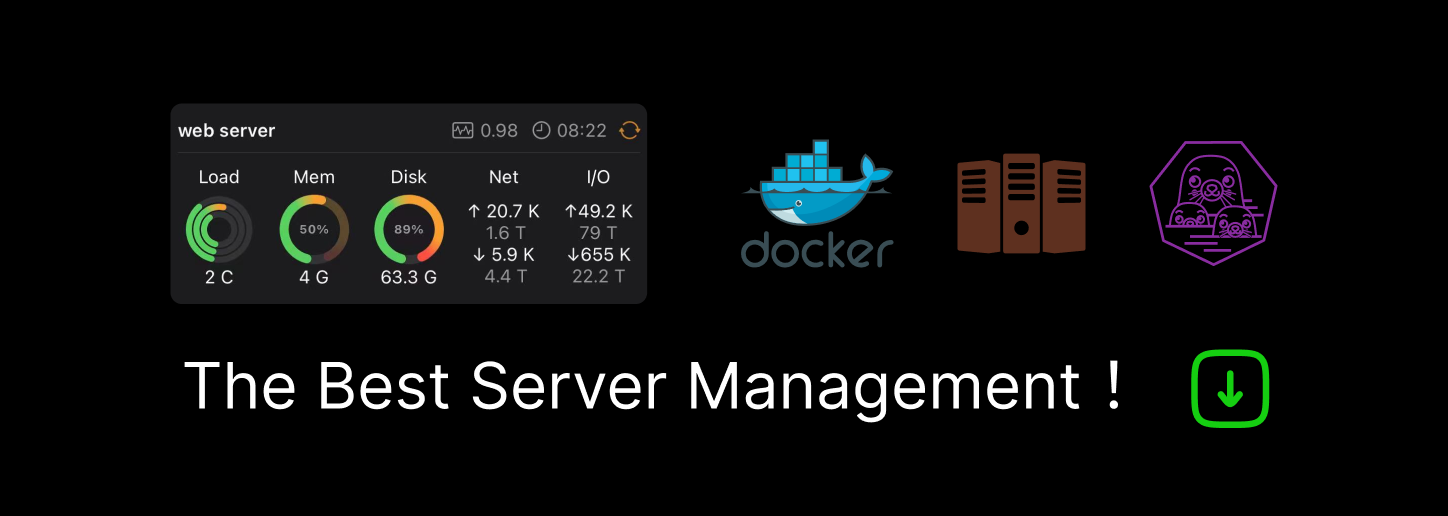
Powering GenAI with Enterprise Retrieval Augmented Generation (RAG)

Businesses are on the lookout for ways to harness the full potential of AI-driven productivity, yet issues with data accuracy, cost and safety continue to be a stumbling block. As Generative AI becomes more accessible with natural language, more employees are using it in many aspects of their work. At the same time, organizations are feverishly looking for enterprise-specific and business oriented use cases that can benefit from Generative AI.
The open-source LLMs upon which GenAI relies are typically trained on publicly available, generic unstructured data and often lack the context of specific enterprise subject matter. Since the training data of most LLMs did not include internal company information, it is unlikely to give accurate answers when queried about topics that rely on enterprise information.
A manager might want to know how an impending storm will affect their warehouses, requiring publicly available weather data and physical stats related to the facilities. A sales rep may need granular data about all retail clients within a certain company size in a specific region, blending company information with geolocation data. LLMs, while adept at generating text based on patterns learned during training, often fall short when faced with queries relating to enterprise information.
LLMs that are not accurate cannot be trusted for business decisions. As recently noted in the 2024 AI & ML Report by Aporia: 89% of respondents report that their Large Language Models (LLMs) exhibit signs of hallucinations.
One way of addressing this problem is training LLMs on internal data sets. However this approach is not optimal, since internal data is changing constantly. Moreover, LLM training is very costly and highly compute intensive. Constantly retraining LLMs therefore is not very feasible.

Illustration of how eRAG enables LLMs to make sense out of structured data, with the help of its unique reasoning engine
Enhancing AI with Retrieval Augmented Generation for Enterprise
Retrieval Augmented Generation (RAG) is an approach that shows much promise in improving the accuracy of GenAI responses so that they can be used in the enterprise to make trusted business decisions. The RAG framework optimizes the output of an LLM, so it references an authoritative knowledge base outside of its training data sources before generating a response. RAG enables LLMs to achieve better accuracy by providing the specific context of a topic being queried, allowing the LLM to generate enriched, detailed answers to inquiries on specific subject matters.
RAG first considers the nuances of the query; then the context in which it is posed, and then the relevance of the available information. An effective prompt service is essential, involving creating concise, clear, and contextually rich prompts to elicit accurate and relevant responses from LLMs. Advanced algorithms, often leveraging the latest in machine learning and deep learning, sift through vast amounts of data to find the most pertinent information. This integration enables the RAG system to pull relevant information from a vast array of external data sources, such as academic databases, online forums and social media platforms.
Optimizing AI for Business Through Enterprise RAG
Typically, RAG frameworks operate on unstructured data. However, most business enterprise data is structured data – stored primarily in relational databases. Enterprise data is a messy business: It is highly complex and often obtuse, consisting of millions of data tables and objects. If delivered to LLMs as is, the LLM would not be able to make any sense of the information. As a result, even the best LLMs today reach accuracy levels of no more than 55%, when querying structured data. This is where enterprise RAG comes in: A RAG framework designed to provide LLMs with the specific context of structured data and enabling LLMs to vastly increase the accuracy of responses to queries relating to enterprise information.
Enterprise RAG aims to make generative AI viable for real-life business environments by addressing the problem of inaccurate or hallucinatory responses, enabling LLMs to query structured data in natural language and achieve human level accuracy. This solution improves productivity by extracting, analyzing, curating and synthesizing internal and external data sources in seconds. In parallel, it is designed to maintain data privacy and data governance with strict authorization controls, especially since custom data sources may contain sensitive information. Enterprise RAG dynamically augments its knowledge base in real-time, incorporating data that can enhance its accuracy, depth, and relevance.
With enterprises scrambling to implement Generative AI across their organizations, new and innovative technologies and approaches are emerging. Ultimately, the goal is to enable employees to interact with enterprise data effortlessly, in natural language. Enterprise RAG is an important step in ensuring that the responses generated can be trusted for business decisions.
Read how GigaSpaces is helping make GenAI viable in the enterprise with enterprise RAG
With more than 10 years’ experience in product leadership roles, Danna Bethlehem currently leads product marketing for GigaSpaces, focusing on GTM for the company's data platform and data hub solutions. Prior to joining GigaSpaces, Danna headed product marketing for authentication and access management solutions in Thales where she contributed to the evolution of the company’s IAM solutions, from MFA, through cloud-based authentication and access management-as-a-service. Over the years, Danna has held product leadership positions in several Israeli startups ranging from financial services to cyber-security.
Recommend
About Joyk
Aggregate valuable and interesting links.
Joyk means Joy of geeK