

Don’t Let Gen AI Limit Your Team’s Creativity
source link: https://hbr.org/2024/03/dont-let-gen-ai-limit-your-teams-creativity?ab=HP-topics-text-21
Go to the source link to view the article. You can view the picture content, updated content and better typesetting reading experience. If the link is broken, please click the button below to view the snapshot at that time.
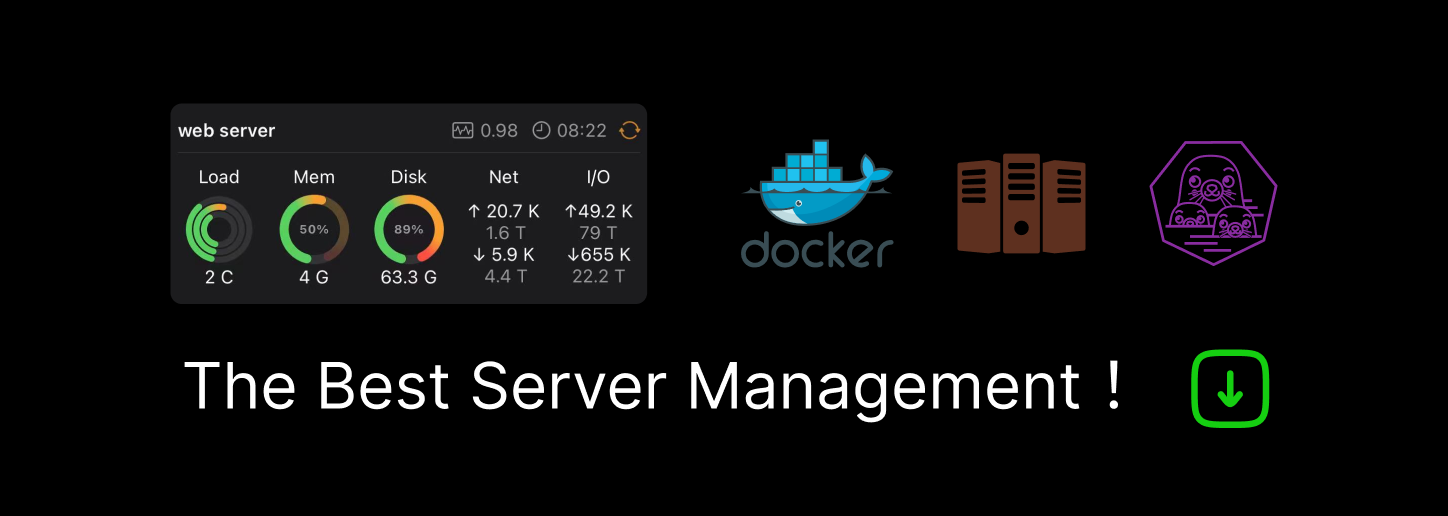
Don’t Let Gen AI Limit Your Team’s Creativity

No one doubts ChatGPT’s ability to generate lots of ideas. But are those ideas any good? In a recent real-world experiment, teams engaged in a creative problem-solving task saw modest gains from AI assistance for the most part—and some underperformed. Don’t blame the technology, says Kian Gohar, CEO of the leadership-development firm GeoLab and one of the study’s authors. Common misconceptions about generative AI, problem-solving, and the creative process are causing workers and their managers to use the tools improperly, sometimes leaving them worse off than if they’d proceeded without AI input.
Gohar and his coresearcher, Jeremy Utley of Stanford University, partnered with four companies: two in Europe and two in the United States. Up to 60 employees in each firm were asked to work in small teams on a business problem their company faced—how to develop internal training resources, for example, or how to scale up B2B sales of a particular product. At each of the firms, some of the teams (those in the control group) approached the problem without any help from AI, while others (those in the experimental group) were given an open-source version of ChatGPT. All the teams watched a short presentation about the problem they were tasked with and had information sheets spelling out relevant details.
The teams had 90 minutes to generate potential solutions, following a structure prescribed by the researchers. Employees first worked individually and then shared their ideas with their teammates during a brainstorming session. Teams in the experimental groups could use ChatGPT during both ideation phases, and they were encouraged to train the tool on the problem by inputting material from the information sheets. At the end of the exercise each team submitted its ideas.
The “owner” of each problem—the person in each organization responsible for implementing the eventual solution—judged the ideas, assigning grades from A (“highly compelling”) to D (“not worth pursuing”) without knowing which had emerged from human-machine collaborations. The results upended the researchers’ expectations, Gohar says. He and his colleagues had assumed that teams leveraging ChatGPT would generate vastly more and better ideas than the others. But those teams produced, on average, just 8% more ideas than teams in the control group did. They got 7% fewer D’s, but they also got 8% more B’s (“interesting but needs development”) and roughly the same share of C’s (“needs significant development”). Most surprising, they got 2% fewer A’s. “Generative AI helped workers avoid awful ideas, but it also led to more average ideas,” Gohar says. Surveys conducted before and after the exercise showed that the teams using AI gained far more confidence in their problem-solving abilities than the others did—a difference of 21%. But the grades they received suggest that much of that confidence was misplaced.
Of course, the potential for gen AI in problem-solving is real, Gohar says. Here are a few steps for maximizing it.
Be precise about the problem you want to solve.
The large language models that undergird generative AI chatbots are designed to give “average” answers; their algorithms have been trained to identify the highest probability of sequential words. If one types, “I bark like a…” and asks the bot to complete the thought, it will almost certainly offer the word “dog.” But if teams are seeking out-of-the-box solutions, average answers will be of little use.
So managers should teach their teams to craft highly specific problem statements, including as much detail as possible, before engaging with the tool. For example, instead of asking, “How can we improve customer satisfaction?” teams could say, “Our customer journey involves the following steps….What changes to our onboarding step will improve retention by 10%?” Gohar comments, “People expect AI to be an oracle: Plug it in, and it will give you your solution.” Teams that took that approach—simply stating the problem in broad terms and asking ChatGPT to solve it—got lackluster results.
Make time for individual brainstorming without the bot.
Before they interact with the AI, give team members some time—15 minutes to a half hour, say—to individually come up with ideas. That will help ensure that they approach the team meeting and the deployment of AI unaffected by groupthink or by what the tool suggests. This step is crucial to gathering diverse and creative ideas and maximizes the number of unique ideas brought to the group for discussion.
Rigorously train the AI.
Gen AI systems lack the contextual understanding that people gain over months or years of working in their organizations and industries. Before integrating ChatGPT or a similar tool into the ideation process, you need to help it catch up. Input as much data related to your specific problem as you can. That might include a customer group’s way of thinking, previous successes and failed initiatives, and industry benchmarks.
Approach AI as an ongoing conversation partner, not an oracle.
The teams in the study developed better ideas when they went back and forth with ChatGPT multiple times. “Most problem-solving requires a conversation,” Gohar says. “You would have a discussion with your colleagues to come up with a better solution to a problem, and that holds when one of those colleagues is ChatGPT.”
Many of the teams in the experiment simply accepted the first suggestion ChatGPT offered up. Gohar attributes this to the Einstellung effect: a cognitive bias whereby people gravitate to early, familiar solutions rather than explore possibilities more expansively. That probably contributed to the high rate of B-grade ideas generated by the AI-assisted teams. No matter how good the tool’s initial suggestion might seem, teams should always follow up with more, and more-specific, questions, Gohar says. Doing so lets the model refine its responses and gives users more solutions to ultimately choose from. “The teams that got A’s were those that had interactive conversations with the bot,” Gohar emphasizes.
Have someone outside the team facilitate the final decision.
When the team comes together to share possible solutions, designate one member to consolidate the suggestions. Then ask the AI to analyze them for alignment with your objectives, offer critiques, challenge assumptions, and suggest more alternatives. This step also serves as a training mechanism and will improve the model’s future performance. It can be useful, Gohar says, to enlist an external facilitator—someone with no dog in the hunt, who ideally is well-versed in AI ideation—to guide the process, help prioritize ideas, and plan next steps.
“Brainstorming with generative AI requires rethinking your ideation workflow and learning new skills,” Gohar concludes. “But if you approach it as a structured, ongoing conversation, you can access a staggering capacity to develop better and more-creative ideas faster.”
About the research: “Evaluating the Practical Impact of Generative AI on Ideation and Team Problem Solving,” by Kian Gohar and Jeremy Utley (working paper)
“The More You Question Generative AI, the Better Its Answers Will Be”
Joe Riesberg is a senior vice president and the chief information officer at Iowa-based EMC Insurance, one of the organizations that participated in the research. He recently spoke with HBR about the experiment and what he learned about best practices for using generative AI. Edited excerpts of the conversation follow.

Why did you join the experiment?
When ChatGPT came out, in November 2022, it was clear that the technology would have huge implications for our business. We immediately began studying it, and by early 2023 we had pinpointed five projects and use cases in which we thought it could improve our performance. So when the research team approached us, we saw it as an opportunity to solve a real business challenge.
What was the challenge?
Our agents’ relationships with customers are crucial, and we wanted to brainstorm ways to further improve them. We fed ChatGPT a few key documents about our company and its services. Then we asked our agents to find the most creative answer to this question: “How might we develop new ways to optimize interactions to enhance agent relationships and ultimately deliver superior customer service?”
Were you surprised by the results?
I was! When I left work that day, I thought that the quality, quantity, and depth of the answers ChatGPT had given my employees on the experimental team were really powerful—surely better than the human-only answers. Turns out I was wrong.
How so?
My team came up with four or five ideas, fed them into the AI system, and asked it to improve on them. Once the system had generated a few responses, they accepted them without asking it for more-nuanced or -creative ones; they were so sure it had given us the best results right off the bat. But the system had generated what it thought would be the “right” solutions—the most logical ones available with the information it had—whereas my colleagues had been tasked with finding the most-creative ones. Too often the AI-assisted teams defaulted to just pasting ChatGPT’s sensible but generic answers into a Word document.
What lessons did you take away from the experiment?
As Midwesterners, we at EMC are sometimes a bit too nice. It doesn’t always come naturally to say to a coworker, “That wasn’t a very creative answer. Give me better ones.” But working with ChatGPT requires direct, unsparing feedback. The more you question generative AI, the better its answers will be. Drawing on it after the initial round of brainstorming can help get people over creative hurdles. And if they’re able to repeatedly challenge the AI to improve its suggestions, they’ll come up with incredible material. It can be hard to learn how to tease out those answers, though; people need time and practice. And the immediate efficiencies from the technology probably won’t be as impressive as people hope. But the improvements from iterating with it—speed, productivity, creativity—will be tremendous in the long term.
Recommend
About Joyk
Aggregate valuable and interesting links.
Joyk means Joy of geeK