

Why OSI wants to hear your feedback on defining open source AI
source link: https://diginomica.com/why-osi-wants-hear-your-feedback-defining-open-source-ai
Go to the source link to view the article. You can view the picture content, updated content and better typesetting reading experience. If the link is broken, please click the button below to view the snapshot at that time.
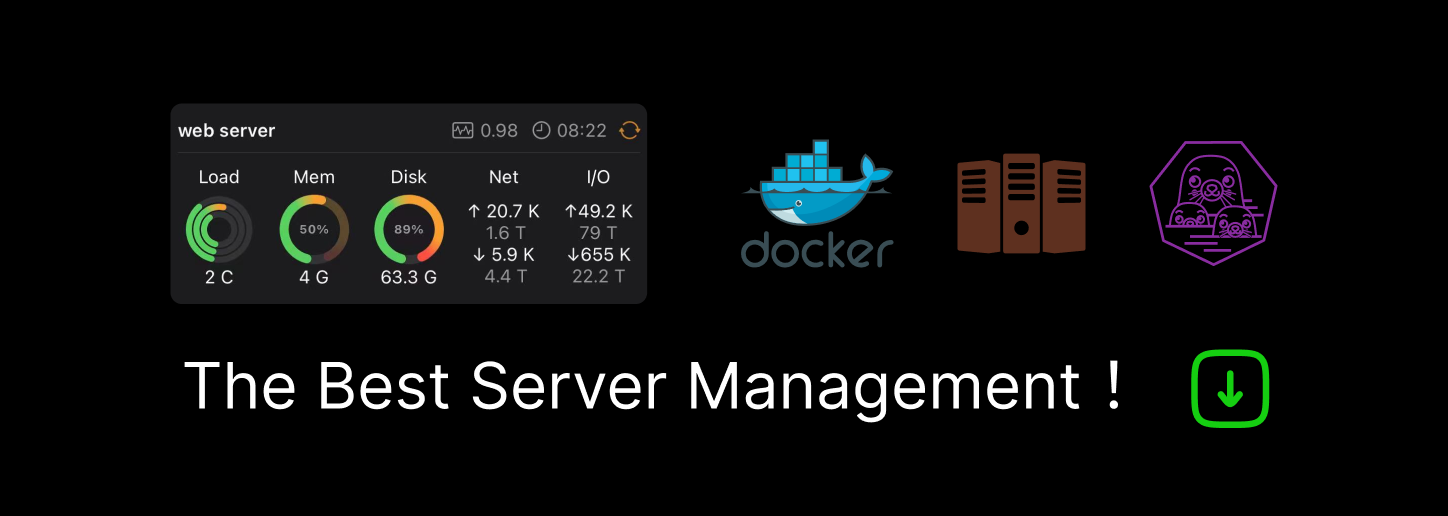
Why OSI wants to hear your feedback on defining open source AI

The Open Source Initiative (OSI) is working to establish a clear and defendable definition of open source AI. This is critical since the word ‘open’ is being tossed around in association with AI, which often strays from being even close to actual open source. There are also legal implications for being truly open source, as diginomica has previously covered.
The OSI has a draft version of the new definition and is soliciting community feedback. Here is the current definition:
To be open source, an AI system needs to make its components available under licenses that individually grant the freedoms to:
- Study how the system works and inspect its components.
- Use the system for any purpose and without having to ask for permission.
- Modify the system to change its recommendations, predictions or decisions to adapt to your needs.
- Share the system with or without modifications, for any purpose.
The definition page also has a preamble about the benefits of AI and notes the definition does not say anything about how to develop and deploy an AI system that is ethical or responsible, although it doesn’t prevent it.
OSI Executive Director Stefano Maffulli says the biggest difference between open source software and AI is that the AI models themselves are not software, they are a completely new type of artefact. There are a lot more moving pieces. He explains:
In our exploration of this topic over the last two years, I’ve learned that with respect to the relationship between AI and open source, we have to stop thinking about the AI world in terms of the individual components — data and data sets, training code and inference code, weights and parameters, model architecture, and the applications on top. Instead, we need to think about AI as a system in order to understand how we want to exercise our freedoms to study, use, modify and share the system as a whole. And that's the way that I've been reframing our conversations about defining open source AI—we need to talk not about the individual components, but rather about the system as a whole.
This also aligns with how many organizations, including the OECD, NIST, the Council on Artificial Intelligence, and regulatory bodies, are approaching the issue. The OSI community is currently focused on building a stable and shared understanding that we need our rights to share, copy, modify, and use an AI system. The next step is to look at a system, such as a machine learning system and ask what kinds of elements or components are needed within that system in order to exercise these four rights.
Democratizing data transparency
Currently, the definition does not consider aspects of transparency into the data used to train AI systems. However, there are some important copyright issues to consider in building better open source AI. Maffulli says:
The conversation about data is interesting in the context of open source because we need to advocate for text and data mining to be excluded from copyright in order to train AI systems. The capability to aggregate large quantities of data needs to be available to everyone, not just a few mega corporations that can agree on cross-licensing deals or have already legally accumulated petabytes of personal data thanks to their terms of service.
The fastest, easiest and most common way of accumulating data has been scraping the internet. That approach comes with a lot of limitations and a lot of challenges. For instance, the internet is not representative of all of society. Many of the text, images and videos online are produced by a subset of people that may include a lot of bias.
The copyright issues around scraping data at scale are also mostly a legal grey area, although, as Chris Middleton points out, data scrapers building AI systems in the UK may soon face regulatory pushback. Content publishers may welcome Google scraping their sites to build a better search experience that paves a digital path to their front door, but less so when new generative AI summaries pose direct competition.
Maffulli argues that the current uncertainty around copyright encourages secrecy that makes it harder to mitigate bias:
If you build a data set by scraping the internet, you are not the copyright holder of the original data. Chances are high that you are using copyrighted data without permission. As a result, you have a very strong incentive to keep secret any data accumulated this way. You may choose not to share it because if you do, you expose yourself to potential legal issues.
And this becomes an even bigger incentive if governments further extend copyright protections to text and data mining and regulate this activity. This would give a strong ‘head start’ advantage to the large corporations like Facebook, Meta, Google, and Microsoft, that have been scraping the internet for a long time or accumulating data under terms and conditions that give them lots of latitude over data not produced by them.
We really need to oppose the widening of copyright to cover text and data mining activities because that would eliminate the ability of researchers in academia and smaller groups to create models that are effective.
Building a safer future
Open source AI could also help reduce the enormous energy requirements of training large language models. There has been a lot of technical innovation for both open and closed AI models. And there is growing interest in training smaller models on more specific data sets and the fine tuning of existing models. Maffulli says this trend underscores the advantage of open source AI systems since models are shared with terms that don't require any special permission to be fine tuned with smaller data sets.
The open source AI movement is facing some pushback from regulators concerned that open source AI might empower hackers, terrorists and geopolitical adversaries. Mafulli believes AI safety discussions need to move beyond the discussions of sci-fi hyped existential risks to address more concrete, realistic risks. In some ways, the hysterical outcry about AI is similar to the vocal faction of doomsayers on the dangers of encryption and GPS in earlier times. He suggests:
I do think it's useful to think about the positive effects for society versus these realistic risks, and the net positive for society is much higher than the limited increased risk. In addressing the more realistic risks of AI, open source is the best defense. Open source gives you the ability to study a system, to understand how it has been built, what kind of bias has been introduced into it, and then modify and tweak it. With open source, experts can double check others' work rather than having individuals or organizations demand, ‘Trust me because I say so.’
In the long run, Maffulli believes that open source AI will accelerate innovation in the same way as traditional open source:
In the ‘80s, when the whole open source movement started, open source contributed to the progression of computer science and helped achieve this fantastic acceleration that computer science as a discipline has had. And I'm expecting that this ‘AI summer’—this new phase of AI where it's finally out of the labs and being deployed—will see a similar acceleration. Already, AI seems to be quite useful and has produced numerous important use cases.
If we get this right, that is, if we can define open source AI and help the widest array of stakeholders—from the legal communities, the researchers in academia, policymakers, small enterprises, large enterprises, and the civil society—understand what open source AI really means, then then we will have not only created a safety net and but also accelerated innovation in the same way that we've seen for open source software.”
My take
In general, the PR hype engine seems to be driving as much innovation in new combinations of the terms ‘open,’ ‘AI,’ and other words as the actual AI developers. Hopefully, a new technical definition of open source AI can provide more clarity for developers, enterprises, and regulators as we move forward.
Recommend
About Joyk
Aggregate valuable and interesting links.
Joyk means Joy of geeK