

如何在langchain中对大模型的输出进行格式化
source link: http://www.flydean.com/007-langchain-output-parthcer/
Go to the source link to view the article. You can view the picture content, updated content and better typesetting reading experience. If the link is broken, please click the button below to view the snapshot at that time.
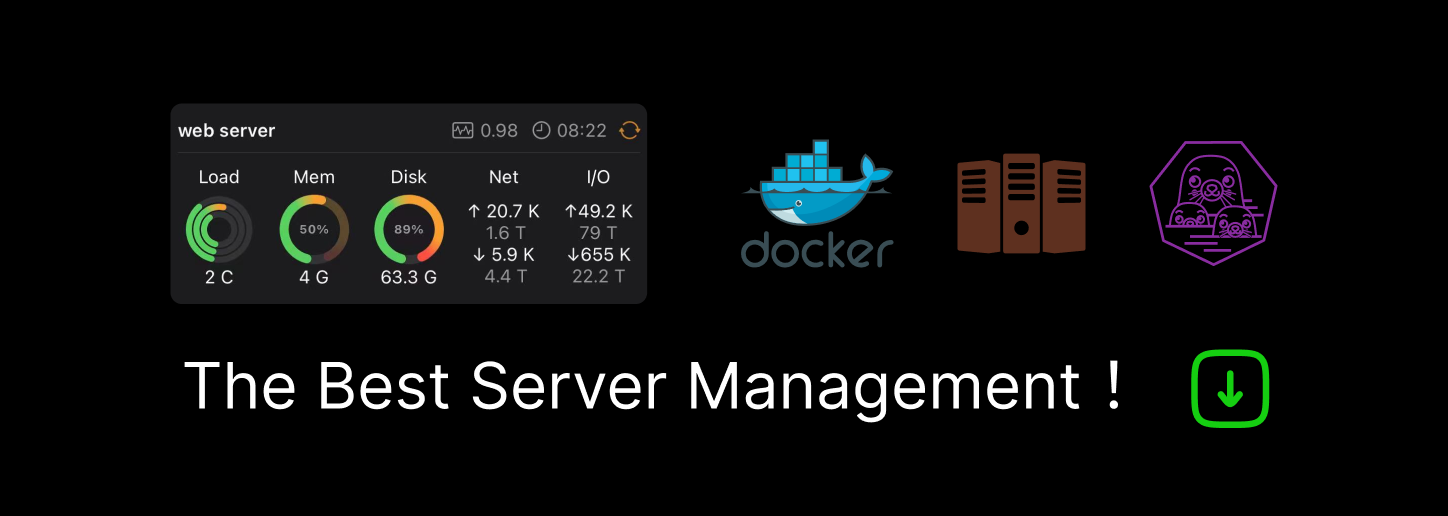
我们知道在大语言模型中, 不管模型的能力有多强大,他的输入和输出基本上都是文本格式的,文本格式的输入输出虽然对人来说非常的友好,但是如果我们想要进行一些结构化处理的话还是会有一点点的不方便。
不用担心,langchain已经为我们想到了这个问题,并且提出了完满的解决方案。
langchain中的output parsers
langchain中所有的output parsers都是继承自BaseOutputParser。这个基础类提供了对LLM大模型输出的格式化方法,是一个优秀的工具类。
我们先来看下他的实现:
class BaseOutputParser(BaseModel, ABC, Generic[T]):
@abstractmethod
def parse(self, text: str) -> T:
"""Parse the output of an LLM call.
A method which takes in a string (assumed output of a language model )
and parses it into some structure.
Args:
text: output of language model
Returns:
structured output
"""
def parse_with_prompt(self, completion: str, prompt: PromptValue) -> Any:
"""Optional method to parse the output of an LLM call with a prompt.
The prompt is largely provided in the event the OutputParser wants
to retry or fix the output in some way, and needs information from
the prompt to do so.
Args:
completion: output of language model
prompt: prompt value
Returns:
structured output
"""
return self.parse(completion)
def get_format_instructions(self) -> str:
"""Instructions on how the LLM output should be formatted."""
raise NotImplementedError
@property
def _type(self) -> str:
"""Return the type key."""
raise NotImplementedError(
f"_type property is not implemented in class {self.__class__.__name__}."
" This is required for serialization."
)
def dict(self, **kwargs: Any) -> Dict:
"""Return dictionary representation of output parser."""
output_parser_dict = super().dict()
output_parser_dict["_type"] = self._type
return output_parser_dict
BaseOutputParser 是一个基础的类,可能被其他特定的输出解析器继承,以实现特定语言模型的输出解析。
这个类使用了Python的ABC模块,表明它是一个抽象基类(Abstract Base Class),不能被直接实例化,而是需要子类继承并实现抽象方法。
Generic[T] 表示这个类是一个泛型类,其中T 是一个类型变量,它表示解析后的输出数据的类型。
@abstractmethod 装饰器标记了 parse 方法,说明它是一个抽象方法,必须在子类中实现。parse 方法接受一个字符串参数 text,通常是语言模型的输出文本,然后将其解析成特定的数据结构,并返回。
parse_with_prompt 方法也是一个抽象方法,接受两个参数,completion 是语言模型的输出,prompt 是与输出相关的提示信息。这个方法是可选的,可以用于在需要时解析输出,可能根据提示信息来调整输出。
get_format_instructions 方法返回关于如何格式化语言模型输出的说明。这个方法可以用于提供解析后数据的格式化信息。
_type 是一个属性,可能用于标识这个解析器的类型,用于后续的序列化或其他操作。
dict 方法返回一个包含输出解析器信息的字典,这个字典可以用于序列化或其他操作。
其中子类必须要实现的方法就是parse。其他的都做为辅助作用。
langchain中有哪些Output Parser
那么langchain中有哪些Output Parser的具体实现呢?具体对应我们应用中的什么场景呢?
接下来我们将会一一道来。
List parser
ListOutputParser的作用就是把LLM的输出转成一个list。ListOutputParser也是一个基类,我们具体使用的是他的子类:CommaSeparatedListOutputParser。
看一下他的parse方法:
def parse(self, text: str) -> List[str]:
"""Parse the output of an LLM call."""
return text.strip().split(", ")
还有一个get_format_instructions:
def get_format_instructions(self) -> str:
return (
"Your response should be a list of comma separated values, "
"eg: `foo, bar, baz`"
)
get_format_instructions是告诉LLM以什么样的格式进行数据的返回。
就是把LLM的输出用逗号进行分割。
下面是一个基本的使用例子:
output_parser = CommaSeparatedListOutputParser()
format_instructions = output_parser.get_format_instructions()
prompt = PromptTemplate(
template="列出几种{subject}.\n{format_instructions}",
input_variables=["subject"],
partial_variables={"format_instructions": format_instructions}
)
_input = prompt.format(subject="水果")
output = model(_input)
print(output)
print(output_parser.parse(output))
我们可以得到下面的输出:
Apple, Orange, Banana, Grape, Watermelon, Strawberry, Pineapple, Peach, Mango, Cherry
['Apple', 'Orange', 'Banana', 'Grape', 'Watermelon', 'Strawberry', 'Pineapple', 'Peach', 'Mango', 'Cherry']
看到这里,大家可能有疑问了, 为什么我们问的是中文,返回的却是因为呢?
这是因为output_parser.get_format_instructions就是用英文描述的,所以LLM会自然的用英文来回答。
别急,我们可以稍微修改下运行代码,如下:
output_parser = CommaSeparatedListOutputParser()
format_instructions = output_parser.get_format_instructions()
prompt = PromptTemplate(
template="列出几种{subject}.\n{format_instructions}",
input_variables=["subject"],
partial_variables={"format_instructions": format_instructions + "用中文回答"}
)
_input = prompt.format(subject="水果")
output = model(_input)
print(output)
print(output_parser.parse(output))
我们在format_instructions之后,提示LLM需要用中文来回答问题。这样我们就可以得到下面的结果:
苹果,橘子,香蕉,梨,葡萄,芒果,柠檬,桃
['苹果,橘子,香蕉,梨,葡萄,芒果,柠檬,桃']
是不是很棒?
Datetime parser
DatetimeOutputParser用来将LLM的输出进行时间的格式化。
class DatetimeOutputParser(BaseOutputParser[datetime]):
format: str = "%Y-%m-%dT%H:%M:%S.%fZ"
def get_format_instructions(self) -> str:
examples = comma_list(_generate_random_datetime_strings(self.format))
return f"""Write a datetime string that matches the
following pattern: "{self.format}". Examples: {examples}"""
def parse(self, response: str) -> datetime:
try:
return datetime.strptime(response.strip(), self.format)
except ValueError as e:
raise OutputParserException(
f"Could not parse datetime string: {response}"
) from e
@property
def _type(self) -> str:
return "datetime"
在get_format_instructions中,他告诉LLM返回的结果是一个日期的字符串。
然后在parse方法中对这个LLM的输出进行格式化,最后返回datetime。
我们看下具体的应用:
output_parser = DatetimeOutputParser()
template = """回答下面问题:
{question}
{format_instructions}"""
prompt = PromptTemplate.from_template(
template,
partial_variables={"format_instructions": output_parser.get_format_instructions()},
)
chain = LLMChain(prompt=prompt, llm=model)
output = chain.run("中华人民共和国是什么时候成立的?")
print(output)
print(output_parser.parse(output))
1949-10-01T00:00:00.000000Z
1949-10-01 00:00:00
回答的还不错,给他点个赞。
Enum parser
如果你有枚举的类型,那么可以尝试使用EnumOutputParser.
EnumOutputParser的构造函数需要传入一个Enum,我们主要看下他的两个方法:
@property
def _valid_values(self) -> List[str]:
return [e.value for e in self.enum]
def parse(self, response: str) -> Any:
try:
return self.enum(response.strip())
except ValueError:
raise OutputParserException(
f"Response '{response}' is not one of the "
f"expected values: {self._valid_values}"
)
def get_format_instructions(self) -> str:
return f"Select one of the following options: {', '.join(self._valid_values)}"
parse方法接收一个字符串 response,尝试将其解析为枚举类型的一个成员。如果解析成功,它会返回该枚举成员;如果解析失败,它会抛出一个 OutputParserException 异常,异常信息中包含了所有有效值的列表。
get_format_instructions告诉LLM需要从Enum的有效value中选择一个输出。这样parse才能接受到正确的输入值。
具体使用的例子可以参考前面两个parser的用法。篇幅起见,这里就不列了。
Pydantic (JSON) parser
JSON可能是我们在日常代码中最常用的数据结构了,这个数据结构很重要。
在langchain中,提供的JSON parser叫做:PydanticOutputParser。
既然要进行JSON转换,必须得先定义一个JSON的类型对象,然后告诉LLM将文本输出转换成JSON格式,最后调用parse方法把json字符串转换成JSON对象。
我们来看一个例子:
class Student(BaseModel):
name: str = Field(description="学生的姓名")
age: str = Field(description="学生的年龄")
student_query = "告诉我一个学生的信息"
parser = PydanticOutputParser(pydantic_object=Student)
prompt = PromptTemplate(
template="回答下面问题.\n{format_instructions}\n{query}\n",
input_variables=["query"],
partial_variables={"format_instructions": parser.get_format_instructions()+"用中文回答"},
)
_input = prompt.format_prompt(query=student_query)
output = model(_input.to_string())
print(output)
print(parser.parse(output))
这里我们定义了一个Student的结构体,然后让LLM给我一个学生的信息,并用json的格式进行返回。
之后我们使用parser.parse来解析这个json,生成最后的Student信息。
我们可以得到下面的输出:
示例输出:{"name": "张三", "age": "18"}
name='张三' age='18'
Structured output parser
虽然PydanticOutputParser非常强大, 但是有时候我们只是需要一些简单的结构输出,那么可以考虑StructuredOutputParser.
我们看一个具体的例子:
response_schemas = [
ResponseSchema(name="name", description="学生的姓名"),
ResponseSchema(name="age", description="学生的年龄")
]
output_parser = StructuredOutputParser.from_response_schemas(response_schemas)
format_instructions = output_parser.get_format_instructions()
prompt = PromptTemplate(
template="回答下面问题.\n{format_instructions}\n{question}",
input_variables=["question"],
partial_variables={"format_instructions": format_instructions}
)
_input = prompt.format_prompt(question="给我一个女孩的名字?")
output = model(_input.to_string())
print(output)
print(output_parser.parse(output))
这个例子是上面的PydanticOutputParser的改写,但是更加简单。
我们可以得到下面的结果:
` ` `json
{
"name": "Jane",
"age": "18"
}
` ` `
{'name': 'Jane', 'age': '18'}
output返回的是一个markdown格式的json字符串,然后通过output_parser.parse得到最后的json。
其他的一些parser
除了json,xml格式也是比较常用的格式,langchain中提供的XML parser叫做XMLOutputParser。
另外,如果我们在使用parser的过程中出现了格式问题,langchain还贴心的提供了一个OutputFixingParser。也就是说当第一个parser报错的时候,或者说不能解析LLM输出的时候,就会换成OutputFixingParser来尝试修正格式问题:
from langchain.output_parsers import OutputFixingParser
new_parser = OutputFixingParser.from_llm(parser=parser, llm=ChatOpenAI())
new_parser.parse(misformatted)
如果错误不是因为格式引起的,那么langchain还提供了一个RetryOutputParser,来尝试重试:
from langchain.output_parsers import RetryWithErrorOutputParser
retry_parser = RetryWithErrorOutputParser.from_llm(
parser=parser, llm=OpenAI(temperature=0)
)
retry_parser.parse_with_prompt(bad_response, prompt_value)
这几个parser都非常有用,大家可以自行尝试。
虽然langchain中的有些parser我们可以自行借助python语言的各种工具来实现。但是有一些parser实际上是要结合LLM一起来使用的,比如OutputFixingParser和RetryOutputParser。
所以大家还是尽可能的使用langchain提供的parser为好。毕竟轮子都给你造好了,还要啥自行车。
Recommend
About Joyk
Aggregate valuable and interesting links.
Joyk means Joy of geeK