

What’s a convolutional neural network and how is it used for image recognition i...
source link: https://www.algolia.com/blog/ai/whats-a-convolutional-neural-network-and-how-is-it-used-for-image-recognition-in-search/
Go to the source link to view the article. You can view the picture content, updated content and better typesetting reading experience. If the link is broken, please click the button below to view the snapshot at that time.
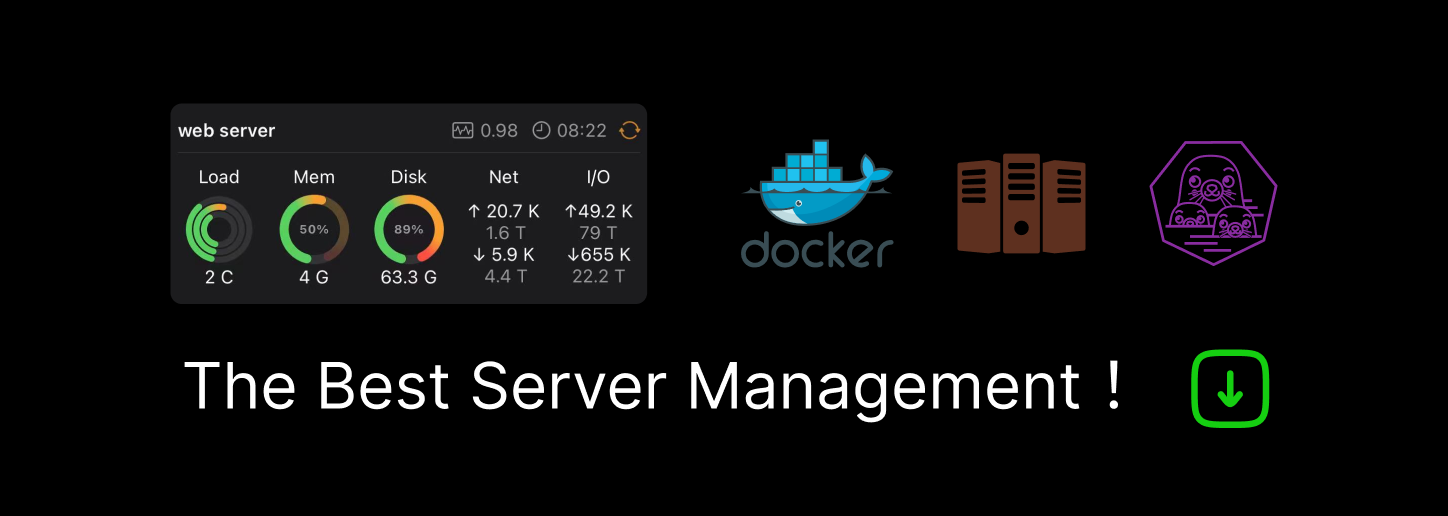
What’s a convolutional neural network and how is it used for image recognition in search?
Mar 1st 2023 ai
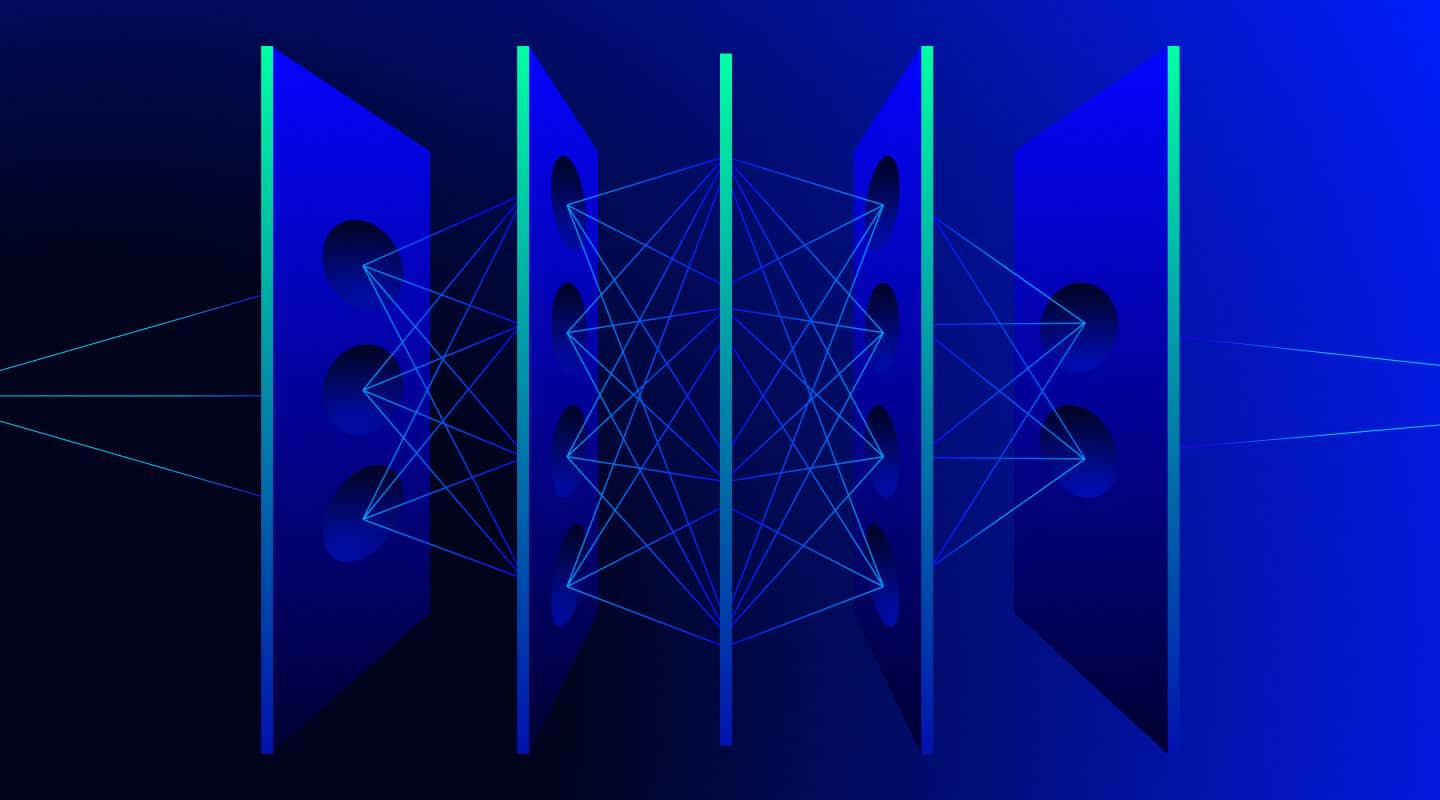
A social media user is shown snapshots of people he may know based on face-recognition technology and asked if he wants to add them as his friends in the app.
A self-driving car moving down a city street uses visual recognition technology for object detection, “seeing” that a pedestrian is about to step off the curb and jaywalk in front of it and decides to respond by slowing down.
A doctor confidently diagnoses her patient’s condition and rules out the presence of malignant cancer cells by being able to use technology to compare thousands of comparable healthcare images of X-rayed ligaments.
A police department generates a clear photo of a suspect that officers can keep on hand. But that’s not all: with generative adversarial networks (GANs), images can then be used to train deep-learning models for facial recognition.
An online retailer suggests people “complete the look” of the jeans they’re considering with tops, jackets, and accessories that other people have chosen to wear with them, as evidenced by social-media image data.
Versatile visual image recognition
These are some of the state-of-the art applications of image-recognition systems, more broadly referred to as computer vision: machines ostensibly “seeing” as people do, perceiving the human environment in the same visual way.
What do all of these image-recognition and -classification applications have in common? They’re expertly handled by a subset of machine learning called a convolutional neural network (CNN, or ConvNet for short).
Definition of a convolutional neural network
A standout in the class of neural networks, a convolutional neural network is a network architecture for deep learning that learns from the data it receives. Among the various types of neural networks, CNNs are the best at identifying images (and videos; plus, they excel with speech and audio signals). In fact, with CNNs, the data input is assumed to be image related.
In its image processing cycle, a convolutional network can assess the image, assign levels of importance to various aspects of it, and differentiate among its visual elements.
The creation of CNN operational structure was inspired by the way neurons are connected in the brain, specifically, the way an animal’s visual cortex is organized. Neurons respond to stimuli only in a certain area — the receptive field. A variety of receptive fields overlap to cover the visual area.
Seeing-eye PCs?
How does an eyeless machine come to expertly master pattern recognition, interpreting images in a convolution operation? You guessed it: with the help of artificial intelligence.
A convolutional neural network architecture comprises a model, a series of statistical functions that calculates and recalculates the pixelated vector of numbers until the image is recognized and classified. It can “see” thanks to the utilization of numbers (weights), statistics, and the processing of data through nodes (neurons or inputs), which have weights and thresholds associated with them.
The first step in this image recognition technology: converting the image’s pixel values into numerical values called vectors, which allows for interpretation of the image and extraction of patterns. When that’s achieved, the data can be fed in.
A deep-learning CNN has several types of layers of nodes, each of which learns to detect different features of an image. In each layer, a filter (a kernel or feature detector) is applied, moving across the receptive fields of the image, checking whether certain features are there and activating certain features.
All the nodes in one layer are connected to every activation unit or node in the next layer. A node is activated — its data is passed along to the connecting node — if its output is higher than the assigned threshold.
The nodes know
In the initial processing layers, the focus is on deciphering straightforward features in the image, such as its colors and edges of elements. With each successive layer iteration, the filter activity delves into more complexity, recognizing elements that represent the input.
The partially recognized image created in each layer is pushed along as the input for the next layer. With each layer, the CNN identifies larger segments of the image.
After each scan, a dot product is calculated. Output from the series of dots is known as a feature map.
With multiple scans, the entire image is processed, and the algorithm identifies what’s in the image.
This refinement process can be repeated for dozens, hundreds, or even thousands of layers, making the image progressively better and more detailed.
That feat, in and of itself, is impressive. But there’s more.
As potentially millions of images are processed by the CNN, the model takes note, calibrates, and realigns its weights. Eventually, it gets so visually confident about what it’s seeing that it can recognize almost any image. And across the world of CNNs, all that perfecting of deep-learning processing skills means the field of computer vision has been improving by leaps and bounds.
Multiple layers
The key to a CNN’s identifying an image is increasing levels of complexity from one layer to the next. Different CNN experts cite different numbers of types of CNN layers (some of which are hidden layers). Regardless of these inconsistencies, the outcome is the same: accurate interpretation of the image.
In addition to the baseline input layer and output layer, the building-block layers include:
The convolutional layer
This first layer is where most of the computations are made. A second convolutional layer for additional categorization after the initial one may be included to facilitate extraction of high-level features from the image.
The pooling layer
This layer reduces complexity/dimensionality in the visual representation — the number of parameters in the input — so some information is lost. This downsampling layer improves efficiency and limits the risk of overfitting.
There are two types of pooling operation:
- Max pooling: As the filter scans the input image, it selects the pixel with the maximum value for passing along to the output array.
- Average pooling: As the filter scans, it calculates the average value within the receptive field to pass along to the output array. This method is used less often than max pooling.
The fully connected layer
This is the layer in which, based on the extracted features, the image is classified. This last layer is “fully connected” (FC) because its nodes are connected with nodes or activation units in another layer.
CNNs are superior
When it comes to visual perception, why are CNNs better than regular neural networks (NNs)?
Regular neural networks (NNs) can’t scale. They don’t incorporate the computational power and resources that a CNN does. NNs may attempt to learn excessive amounts of detail in the training data (known as overfitting). If you feed millions of photos into a computer and ask it to consider every detail as important in its image recognition work, including what amounts to visual “noise,” this can distort image classification.
A CNN architecture is better for images because it utilizes a method called parameter sharing, which reduces the computational intensity compared with an NN. In each of its layers, each node is connected to another node. As the filters progress across the image in a given layer, the associated weights stay fixed.
How CNNs have improved image recognition in search
Thanks to CNNs accurately processing visual information, classifying images, and improving computer vision, the field of visual search has been exploding. This visual-processing phenomenon is particularly evident in ecommerce, where sites can now offer users the advantages and pleasures of visual shopping.
At Algolia, we help companies make it easy for real-world people to use image search to find exactly the item they want, plus encourage upselling with features such as “Complete the look,” and more.
Want to enhance your site search results with our CNN-aided image search technology? Contact us and we’ll help you see — and pursue — all the possibilities.
Recommend
About Joyk
Aggregate valuable and interesting links.
Joyk means Joy of geeK