

在 Mac M1 上运行 pytorch MNIST 模型
source link: https://blog.kelu.org/tech/2023/02/17/pytorch-mnist-tutorial.html
Go to the source link to view the article. You can view the picture content, updated content and better typesetting reading experience. If the link is broken, please click the button below to view the snapshot at that time.
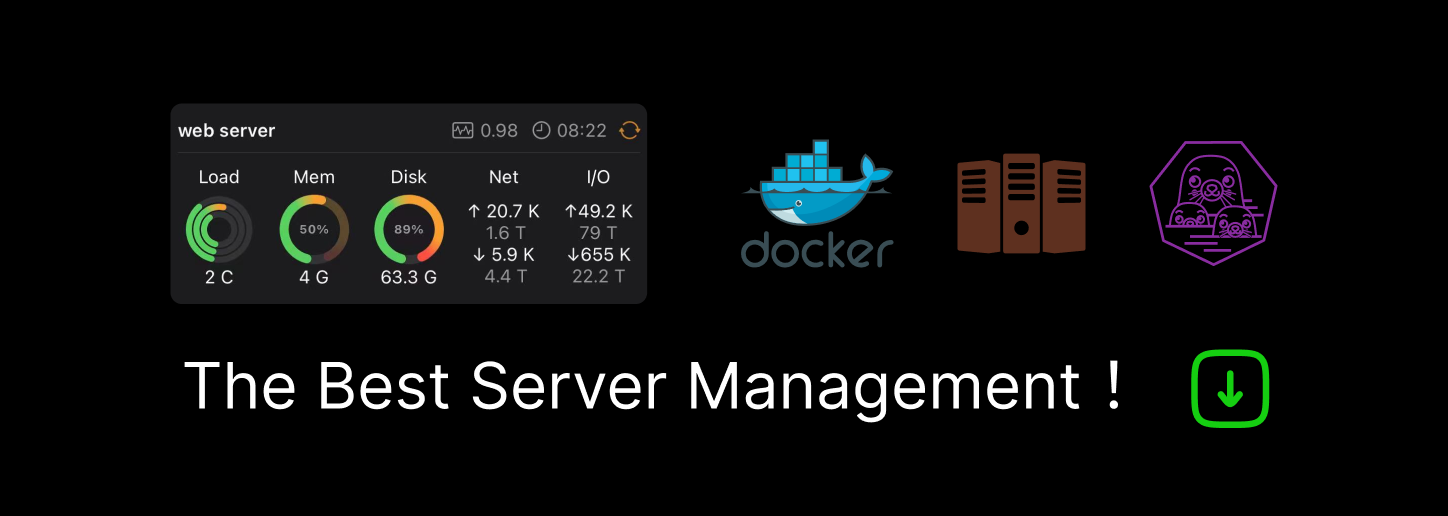
http://yann.lecun.com/exdb/mnist/

MNIST 包括6万张28x28的训练样本,1万张测试样本,很多教程都会对它”下手”几乎成为一个 “典范”,可以说它就是计算机视觉里面的Hello World。所以我们这里也会使用MNIST来进行实战。
前面在介绍卷积神经网络的时候说到过LeNet-5,LeNet-5之所以强大就是因为在当时的环境下将MNIST数据的识别率提高到了99%,这里我们也自己从头搭建一个卷积神经网络,也达到99%的准确率。
下载这个文件 pytorch-m1-gpu-mnist.py,源码我贴到文末。
上传到jupyer:

新建一个运行文件

在jupter种运行文件:
%load pytorch-m1-gpu-mnist.py
%run pytorch-m1-gpu-mnist.py

点击Run:

最后输出大致是这样:
Train Epoch: 5 [0/60000 (0%)] Loss: 0.046214
Train Epoch: 5 [1280/60000 (2%)] Loss: 0.054890
Train Epoch: 5 [2560/60000 (4%)] Loss: 0.126030
Train Epoch: 5 [3840/60000 (6%)] Loss: 0.037496
Train Epoch: 5 [5120/60000 (9%)] Loss: 0.082181
Train Epoch: 5 [6400/60000 (11%)] Loss: 0.037875
Train Epoch: 5 [7680/60000 (13%)] Loss: 0.085949
Train Epoch: 5 [8960/60000 (15%)] Loss: 0.060418
Train Epoch: 5 [10240/60000 (17%)] Loss: 0.118635
Train Epoch: 5 [11520/60000 (19%)] Loss: 0.023937
Train Epoch: 5 [12800/60000 (21%)] Loss: 0.182207
Train Epoch: 5 [14080/60000 (23%)] Loss: 0.076019
Train Epoch: 5 [15360/60000 (26%)] Loss: 0.033017
Train Epoch: 5 [16640/60000 (28%)] Loss: 0.055296
Train Epoch: 5 [17920/60000 (30%)] Loss: 0.028324
Train Epoch: 5 [19200/60000 (32%)] Loss: 0.049647
Train Epoch: 5 [20480/60000 (34%)] Loss: 0.056441
Train Epoch: 5 [21760/60000 (36%)] Loss: 0.079691
Train Epoch: 5 [23040/60000 (38%)] Loss: 0.065786
Train Epoch: 5 [24320/60000 (41%)] Loss: 0.064102
Train Epoch: 5 [25600/60000 (43%)] Loss: 0.165235
Train Epoch: 5 [26880/60000 (45%)] Loss: 0.047473
Train Epoch: 5 [28160/60000 (47%)] Loss: 0.123398
Train Epoch: 5 [29440/60000 (49%)] Loss: 0.044776
Train Epoch: 5 [30720/60000 (51%)] Loss: 0.070954
Train Epoch: 5 [32000/60000 (53%)] Loss: 0.048687
Train Epoch: 5 [33280/60000 (55%)] Loss: 0.129717
Train Epoch: 5 [34560/60000 (58%)] Loss: 0.075629
Train Epoch: 5 [35840/60000 (60%)] Loss: 0.026882
Train Epoch: 5 [37120/60000 (62%)] Loss: 0.035822
Train Epoch: 5 [38400/60000 (64%)] Loss: 0.020158
Train Epoch: 5 [39680/60000 (66%)] Loss: 0.037771
Train Epoch: 5 [40960/60000 (68%)] Loss: 0.024614
Train Epoch: 5 [42240/60000 (70%)] Loss: 0.070286
Train Epoch: 5 [43520/60000 (72%)] Loss: 0.104104
Train Epoch: 5 [44800/60000 (75%)] Loss: 0.021874
Train Epoch: 5 [46080/60000 (77%)] Loss: 0.027039
Train Epoch: 5 [47360/60000 (79%)] Loss: 0.029215
Train Epoch: 5 [48640/60000 (81%)] Loss: 0.033327
Train Epoch: 5 [49920/60000 (83%)] Loss: 0.008433
Train Epoch: 5 [51200/60000 (85%)] Loss: 0.058720
Train Epoch: 5 [52480/60000 (87%)] Loss: 0.039366
Train Epoch: 5 [53760/60000 (90%)] Loss: 0.109450
Train Epoch: 5 [55040/60000 (92%)] Loss: 0.042343
Train Epoch: 5 [56320/60000 (94%)] Loss: 0.077304
Train Epoch: 5 [57600/60000 (96%)] Loss: 0.021365
Train Epoch: 5 [58880/60000 (98%)] Loss: 0.090809
Test set: Average loss: 0.0475, Accuracy: 9853/10000 (99%)
"""
MNIST with PyTorch on Apple Silicon GPU
Script will be linked in the description as a Github Gist.
Install PyTorch nightly with this command:
pip3 install --pre torch torchvision torchaudio --extra-index-url https://download.pytorch.org/whl/nightly/cpu
Code borrowed from PyTorch Examples.
"""
import torch
from torch import nn, optim
import torch.nn.functional as F
import torchvision
from torchvision import datasets, transforms
EPOCHS = 5
class Net(nn.Module):
def __init__(self):
super(Net, self).__init__()
self.conv1 = nn.Conv2d(1, 20, 5, 1)
self.conv2 = nn.Conv2d(20, 50, 5, 1)
self.fc1 = nn.Linear(4*4*50, 500)
self.fc2 = nn.Linear(500, 10)
def forward(self, x):
x = F.relu(self.conv1(x))
x = F.max_pool2d(x, 2, 2)
x = F.relu(self.conv2(x))
x = F.max_pool2d(x, 2, 2)
x = x.view(-1, 4*4*50)
x = F.relu(self.fc1(x))
x = self.fc2(x)
return F.log_softmax(x, dim=1)
def train(model, device, train_loader, optimizer, epoch):
model.train()
for batch_idx, (data, target) in enumerate(train_loader):
data, target = data.to(device), target.to(device)
optimizer.zero_grad()
output = model(data)
loss = F.nll_loss(output, target)
loss.backward()
optimizer.step()
if batch_idx % 10 == 0:
print('Train Epoch: {} [{}/{} ({:.0f}%)]\tLoss: {:.6f}'.format(
epoch, batch_idx * len(data), len(train_loader.dataset),
100. * batch_idx / len(train_loader), loss.item()))
def test(model, device, test_loader):
model.eval()
test_loss = 0
correct = 0
with torch.no_grad():
for data, target in test_loader:
data, target = data.to(device), target.to(device)
output = model(data)
test_loss += F.nll_loss(output, target, reduction='sum').item() # sum up batch loss
pred = output.argmax(dim=1, keepdim=True) # get the index of the max log-probability
correct += pred.eq(target.view_as(pred)).sum().item()
test_loss /= len(test_loader.dataset)
print('\nTest set: Average loss: {:.4f}, Accuracy: {}/{} ({:.0f}%)\n'.format(
test_loss, correct, len(test_loader.dataset),
100. * correct / len(test_loader.dataset)))
def main():
print("PyTorch version:", torch.__version__)
print("Torchvision version:", torchvision.__version__)
device = torch.device("mps")
print("Using Device: ", device)
train_loader = torch.utils.data.DataLoader(
datasets.MNIST('../data', train=True, download=True,
transform=transforms.Compose([
transforms.ToTensor(),
transforms.Normalize((0.1307,), (0.3081,))
])),
batch_size=128, shuffle=True)
test_loader = torch.utils.data.DataLoader(
datasets.MNIST('../data', train=False, transform=transforms.Compose([
transforms.ToTensor(),
transforms.Normalize((0.1307,), (0.3081,))
])),
batch_size=128, shuffle=True)
model = Net().to(device)
optimizer = optim.SGD(model.parameters(), lr=0.01, momentum=0.5)
for epoch in range(1, EPOCHS + 1):
train(model, device, train_loader, optimizer, epoch)
test(model, device, test_loader)
if __name__ == "__main__":
main()
Recommend
About Joyk
Aggregate valuable and interesting links.
Joyk means Joy of geeK