

了解Pytorch|Get Started with PyTorch - 孤飞
source link: https://www.cnblogs.com/ranxi169/p/16739887.html
Go to the source link to view the article. You can view the picture content, updated content and better typesetting reading experience. If the link is broken, please click the button below to view the snapshot at that time.
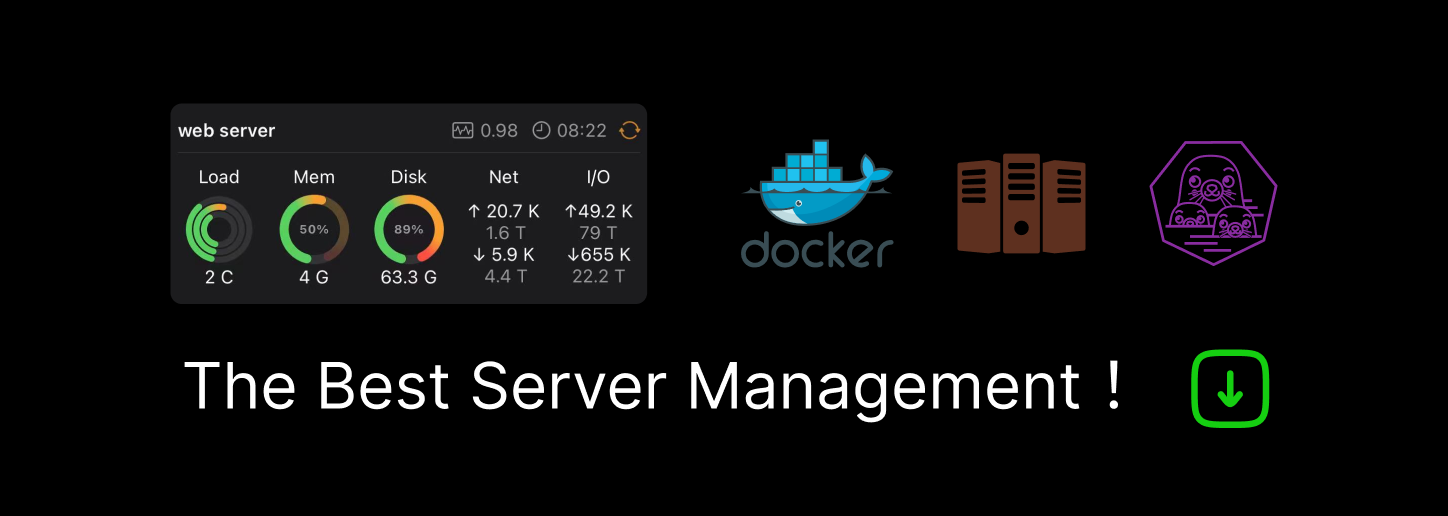
一个开源的机器学习框架,加速了从研究原型到生产部署的路径。!pip install torch -i https://pypi.tuna.tsinghua.edu.cn/simple
1 2
import torch import numpy as np
Basics
就像Tensorflow一样,我们也将继续在PyTorch中玩转Tensors。
从数据(列表)中创建张量
1 2
data = [[1, 2],[3, 4]] tensors = torch.tensor(data)
tensors
tensor([[1, 2],
[3, 4]])
从NumPy创建
1 2
np_array = np.arange(10) tensor_np = torch.from_numpy(np_array)
tensor_np
tensor([0, 1, 2, 3, 4, 5, 6, 7, 8, 9], dtype=torch.int32)
形状、ndim和dtype
这与我们在《Numpy教程--第1天》中看到的相同。
tensor_np.shape
torch.Size([10])
tensor_np.ndim
tensor_np.dtype
torch.int32
张量操作(Tensor_Operations)
1 2 3
ten1 = torch.tensor([1,2,3]) ten2 = torch.tensor([4,5,6]) ten1+ten2
tensor([5, 7, 9])
你可以使用+
或torch.add
来执行张量添加。
torch.sub(ten2,ten1)
tensor([3, 3, 3])
torch.add(ten1,ten2)
tensor([5, 7, 9])
torch.subtract(ten2,ten1)
tensor([3, 3, 3])
你可以使用-
或torch.sub
来执行张量添加。
ten1*10
tensor([10, 20, 30])
深度学习中非常重要的操作--矩阵乘法
Rules of Matrix Multiplication:
- (3,2) * (3,2) = Error
- (4,3) * (3,2) = (4,2)
- (2,2) * (2,5) = (2,5)
torch.matmul(ten1,ten2)
tensor(32)
1 2 3 4
matrix4_3 = torch.tensor([[1,2,3], [4,5,6], [7,8,9], [10,11,12]])
matrix4_3.shape
torch.Size([4, 3])
1 2 3
matrix3_2 = torch.tensor([[1,2], [3,4], [5,6]])
matrix3_2.shape
torch.Size([3, 2])
result = torch.matmul(matrix4_3,matrix3_2) #=> will result in (4,2)
result
tensor([[ 22, 28],
[ 49, 64],
[ 76, 100],
[103, 136]])
result.shape
torch.Size([4, 2])
你也可以使用torch.mm()
,这是torch.matmul()
的简称。
torch.mm(matrix4_3,matrix3_2)
tensor([[ 22, 28],
[ 49, 64],
[ 76, 100],
[103, 136]])
1 2
#张量的转置 matrix4_3
tensor([[ 1, 2, 3],
[ 4, 5, 6],
[ 7, 8, 9],
[10, 11, 12]])
matrix4_3.T
tensor([[ 1, 4, 7, 10],
[ 2, 5, 8, 11],
[ 3, 6, 9, 12]])
torch.t(matrix4_3)
tensor([[ 1, 4, 7, 10],
[ 2, 5, 8, 11],
[ 3, 6, 9, 12]])
更多张量操作
- Zeros
- Random
1 2
tensorZeroes = torch.zeros((3,3)) tensorZeroes
tensor([[0., 0., 0.],
[0., 0., 0.],
[0., 0., 0.]])
1 2
tensorOnes = torch.ones((3,3)) tensorOnes
tensor([[1., 1., 1.],
[1., 1., 1.],
[1., 1., 1.]])
1 2
tensorRandomN = torch.randn((3,3)) #includes negative tensors tensorRandomN
tensor([[ 1.3255, -0.4937, 1.0488],
[ 1.1797, -0.5422, -0.9703],
[-0.1761, 1.0742, 0.5459]])
1 2
tensorRandom = torch.rand((3,3)) #includes only positive tensors tensorRandom
tensor([[0.2013, 0.9272, 0.7866],
[0.5887, 0.9900, 0.3554],
[0.6128, 0.3316, 0.6635]])
1 2
customFill = torch.full((3,3),5) customFill
tensor([[5, 5, 5],
[5, 5, 5],
[5, 5, 5]])
1 2
initialFill = torch.full((3,3),0.01) initialFill
tensor([[0.0100, 0.0100, 0.0100],
[0.0100, 0.0100, 0.0100],
[0.0100, 0.0100, 0.0100]])
快速入门Torchvision
安装Torchvision,Torchvision软件包,包括流行的数据集、模型架构和计算机视觉的常见图像转换。
!pip install torchvision --no-deps -i https://pypi.tuna.tsinghua.edu.cn/simple
1 2 3 4
from torch.utils.data import DataLoader from torchvision import datasets from torchvision.transforms import ToTensor from torch import nn
1 2 3 4 5 6 7
# Download training data from open datasets. training_data = datasets.FashionMNIST( root="data", train=True, download=True, transform=ToTensor(), )
1 2 3 4 5 6 7
# Download test data from open datasets. test_data = datasets.FashionMNIST( root="data", train=False, download=True, transform=ToTensor(), )
type(training_data)
torchvision.datasets.mnist.FashionMNIST
Dataloader
在我们的数据集上包裹了一个迭代器,并支持自动批处理、采样、洗牌和多进程数据加载。这里我们定义了一个64的批处理量,即dataloader可迭代的每个元素将返回64个特征和标签的批次。
1 2 3 4 5 6 7 8
import matplotlib.pyplot as plt plt.figure(figsize=(12,10)) for i in range(9): plt.subplot(3,3,i+1) sample_image,sample_label = training_data[i] plt.imshow(sample_image[0]) plt.title(sample_label)
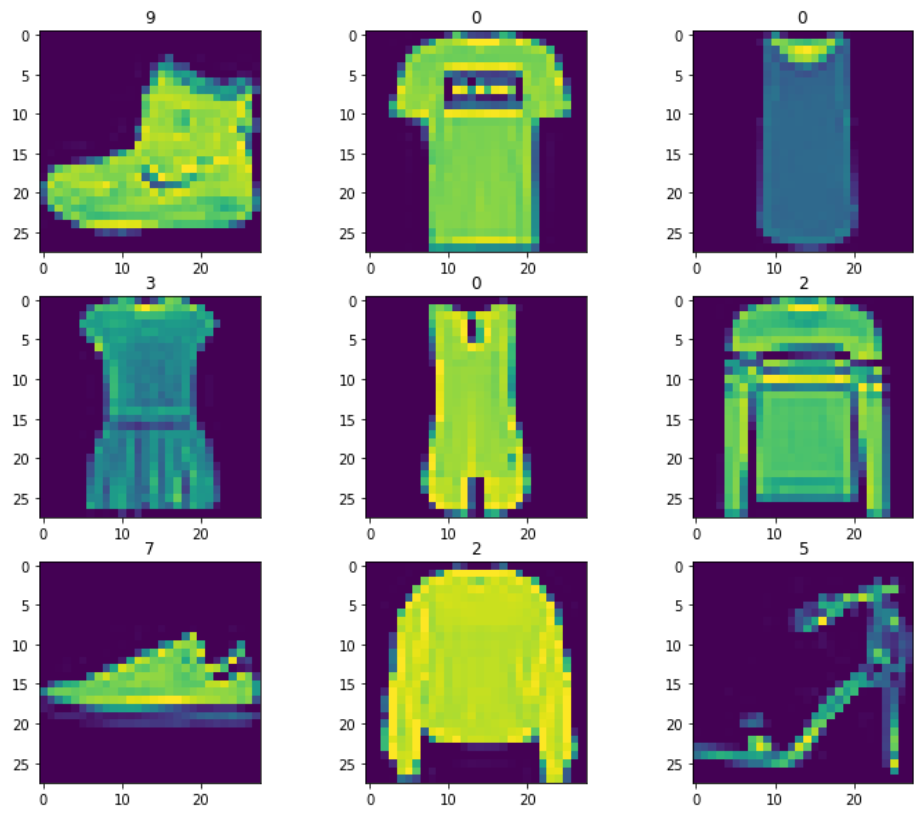
1 2 3 4 5 6 7 8 9
batch_size = 64 training = DataLoader(training_data,batch_size=batch_size) testing = DataLoader(test_data, batch_size=batch_size) for X, y in testing: print(f"Shape of X: {X.shape}") print(f"Shape of y: {y.shape}") break
Shape of X: torch.Size([64, 1, 28, 28])
Shape of y: torch.Size([64])
1 2 3 4
for X,y in training: print(torch.max(X)) print(torch.min(X)) break
tensor(1.)
tensor(0.)
我们不需要扩展,因为 DataLoader
会处理这个问题。
1 2 3 4 5 6 7 8 9 10 11 12 13 14 15
class NeuralNetwork(nn.Module): def __init__(self): super(NeuralNetwork,self).__init__() self.flatten = nn.Flatten() self.build_model = nn.Sequential( nn.Linear(28*28,512), #28*28 is input shape nn.ReLU(), nn.Linear(512,512), #hidden layer nn.ReLU(), nn.Linear(512,10) #output layer ) def forward(self,x): x = self.flatten(x) dnn = self.build_model(x) return dnn
model = NeuralNetwork()
1 2 3
# compile model - Loss Function and Optimizer loss_fn = nn.CrossEntropyLoss() optimizer = torch.optim.Adam(model.parameters(), lr=1e-5)
1 2 3 4 5 6 7 8 9 10 11 12 13 14 15 16
def train(dataloader, model, loss_fn, optimizer): size = len(dataloader.dataset) model.train() for batch, (X, y) in enumerate(dataloader): # Compute prediction error pred = model(X) loss = loss_fn(pred, y) # Backpropagation optimizer.zero_grad() loss.backward() optimizer.step() if batch % 100 == 0: loss, current = loss.item(), batch * len(X) print(f"loss: {loss:>7f} [{current:>5d}/{size:>5d}]")
1 2 3 4 5 6 7 8 9 10 11 12 13
def test(dataloader, model, loss_fn): size = len(dataloader.dataset) num_batches = len(dataloader) model.eval() test_loss, correct = 0, 0 with torch.no_grad(): for X, y in dataloader: pred = model(X) test_loss += loss_fn(pred, y).item() correct += (pred.argmax(1) == y).type(torch.float).sum().item() test_loss /= num_batches correct /= size print(f"Test Error: \n Accuracy: {(100*correct):>0.1f}%, Avg loss: {test_loss:>8f} \n")
1 2 3 4 5
for epoch in range(5): print(f"Epochs {epoch+1}") train(training, model, loss_fn, optimizer) test(testing, model, loss_fn) print("Done!")
Epochs 1
loss: 0.473322 [ 0/60000]
loss: 0.569312 [ 6400/60000]
loss: 0.383823 [12800/60000]
loss: 0.613123 [19200/60000]
loss: 0.511312 [25600/60000]
loss: 0.534981 [32000/60000]
loss: 0.519904 [38400/60000]
loss: 0.663009 [44800/60000]
loss: 0.595559 [51200/60000]
loss: 0.510713 [57600/60000]
Test Error:
Accuracy: 81.6%, Avg loss: 0.523760
Epochs 2
loss: 0.441475 [ 0/60000]
loss: 0.541651 [ 6400/60000]
loss: 0.362368 [12800/60000]
loss: 0.587903 [19200/60000]
loss: 0.489257 [25600/60000]
loss: 0.512706 [32000/60000]
loss: 0.496316 [38400/60000]
loss: 0.658995 [44800/60000]
loss: 0.588307 [51200/60000]
loss: 0.486178 [57600/60000]
Test Error:
Accuracy: 82.2%, Avg loss: 0.507999
Epochs 3
loss: 0.414868 [ 0/60000]
loss: 0.520754 [ 6400/60000]
loss: 0.345219 [12800/60000]
loss: 0.567657 [19200/60000]
loss: 0.470389 [25600/60000]
loss: 0.493463 [32000/60000]
loss: 0.477664 [38400/60000]
loss: 0.654533 [44800/60000]
loss: 0.580627 [51200/60000]
loss: 0.466487 [57600/60000]
Test Error:
Accuracy: 82.7%, Avg loss: 0.495437
Epochs 4
loss: 0.391931 [ 0/60000]
loss: 0.504477 [ 6400/60000]
loss: 0.331017 [12800/60000]
loss: 0.550430 [19200/60000]
loss: 0.453982 [25600/60000]
loss: 0.477417 [32000/60000]
loss: 0.462027 [38400/60000]
loss: 0.649069 [44800/60000]
loss: 0.573334 [51200/60000]
loss: 0.450685 [57600/60000]
Test Error:
Accuracy: 83.0%, Avg loss: 0.485073
Epochs 5
loss: 0.372204 [ 0/60000]
loss: 0.491510 [ 6400/60000]
loss: 0.318891 [12800/60000]
loss: 0.536430 [19200/60000]
loss: 0.440059 [25600/60000]
loss: 0.463519 [32000/60000]
loss: 0.449640 [38400/60000]
loss: 0.642708 [44800/60000]
loss: 0.565997 [51200/60000]
loss: 0.438368 [57600/60000]
Test Error:
Accuracy: 83.2%, Avg loss: 0.476352
Done!
我们将在下一个笔记本序列中探讨更多关于神经网络的问题。
Notebook:了解PytorchGet Started with PyTorch | Kaggle
原文作者:孤飞-博客园
我的个人博客:https://blog.onefly.top
Recommend
About Joyk
Aggregate valuable and interesting links.
Joyk means Joy of geeK