

折腾一晚上的事情,明白了一个道理
source link: https://www.cnblogs.com/30go/p/16654852.html
Go to the source link to view the article. You can view the picture content, updated content and better typesetting reading experience. If the link is broken, please click the button below to view the snapshot at that time.
折腾一晚上的事情,明白了一个道理
感悟:有时候很简单的笨办法,比那些高大上的技术要实用的多。
有一个数据同步,大约4亿条记录,没有分区。现在要按照天,小时分区写入到iceberg的分区表中。
源数据中本身就是很多几十k大小的非常多的小文件。
于是在读取时,总想着要shuffle,合并小文件,于是是这样的:
hive_df = spark.table("xx.hive_test")
hive_df.repartition(1).write.format('iceberg').mode('overwrite').save('iceberg_dw.iceberg_test')
但是iceberg表写入分区表时,需要根据分区本地排序,或者全局排序,于是写成这样:
hive_df = spark.table("xx.hive_test")
hive_df.repartition(1).sortWithinPartitions("pk_day","pk_hour").write.format('iceberg').mode('overwrite').save('iceberg_dw.iceberg_test')
但是执行起来后,分区排序合并都没有问题,但是由于数据量巨大,都卡在最后的1个partition任务那。于是又改成了多个重分区。
hive_df = spark.table("xx.hive_test")
hive_df.repartition(20).sortWithinPartitions("pk_day","pk_hour").write.format('iceberg').mode('overwrite').save('iceberg_dw.iceberg_test')
但是依然很慢,于是加大了分区
hive_df = spark.table("xx.hive_test")
hive_df.repartition(100).sortWithinPartitions("pk_day","pk_hour").write.format('iceberg').mode('overwrite').save('iceberg_dw.iceberg_test')
折腾了大半夜,通过增大excuter个数,内存大小还是不行。
早上醒来,忽然想到了大数据的分而治之的想法,以前跑数据的时候也干过,将源头数据分几段来读取写入,多跑几个任务就可以。
于是修改为以下代码,通过传入年月日,时分秒,再转换为对应的时间戳取查询源头的记录,将单个小时的合并为1个分区写入目标表。
pySpark程序命名为hive2iceberg.py
from pyspark.sql import SparkSession
import sys,datetime,time,pytz
day_hour = sys.argv[1] + ' ' + sys.argv[2] # 2022-09-02 09:00:00
utc_timestamp = time.mktime(datetime.datetime.strptime(day_hour, '%Y-%m-%d %H:%M:%S').replace(tzinfo=pytz.utc).timetuple())
dt = int(utc_timestamp) * 1000
pk_day = dt - dt % 86400000
dayBeforeYesterday = (dt - dt % 86400000) - 86400000
pk_hour = dt - dt % 3600000
print("=====================================================================================================================")
print(">>>>>>>>>>>>>>>>日期小时:" + str(day_hour) + " pk_day:" + str(pk_day), "dayBeforeYesterday:" + str(dayBeforeYesterday) + " hour:" + str(pk_hour))
print("=====================================================================================================================")
pyfilename= "hive2iceberg"
spark = SparkSession \
.builder \
.config("spark.sql.shuffle.partitions", "1000") \
.config("spark.driver.maxResultSize", "6g") \
.config("spark.debug.maxToStringFields", "1000") \
.config("spark.sql.iceberg.handle-timestamp-without-timezone", "true") \
.config("spark.sql.session.timeZone", "UTC") \
.config("spark.sql.crossJoin.enabled","true") \
.config("spark.sql.catalog.iceberg", "org.apache.iceberg.spark.SparkCatalog") \
.config("iceberg.engine.hive.enabled","true") \
.config("hive.exec.dynamic.partition", "true") \
.config("hive.exec.dynamic.partition.mode", "nonstrict") \
.config("hive.exec.max.dynamic.partitions", "5000") \
.appName(pyfilename) \
.enableHiveSupport() \
.getOrCreate()
df = spark.table("xx.hive_test").where("pk_day = %d and pk_hour = %d " % (pk_day,pk_hour))
df.repartition(1).sortWithinPartitions("pk_day","pk_hour").write.format('iceberg').mode('overwrite').save('iceberg_dw.iceberg_test')
然后又准备了一段shell脚本run_state_hive2iceberg_fixdata.sh如下:
#!/bin/sh
if [ $# = 1 ]
then
start_dt=$1
end_dt=$1
elif [ $# = 2 ]
then
start_dt=$1
end_dt=$2
elif [ $# = 0 ]
then
start_dt=`date -d '-1 hour' +%Y%m%d-%H`
end_dt=`date -d '-1 hour' +%Y%m%d-%H`
fi
date_format_1=${start_dt:0:4}-${start_dt:4:2}-${start_dt:6:2}' '${start_dt:9:2}:00:00
date_format_2=${end_dt:0:4}-${end_dt:4:2}-${end_dt:6:2}' '${end_dt:9:2}:00:00
start_sec=`date -d "$date_format_1" +%s`
end_sec=`date -d "$date_format_2" +%s`
for ((i=$start_sec;i<=$end_sec;i+=3600))
do
file_day=`date -d @$i +%Y%m%d`
data_day=`date -d @$i +%Y-%m-%d`
data_hour=`date -d @$i +"%H:00:00"`
echo ${data_day} ${data_hour}
spark-submit \
--conf spark.dynamicAllocation.enabled=false \
--name hive2iceberg \
--master yarn \
--deploy-mode cluster \
--queue prod \
--driver-memory 2G \
--num-executors 5 \
--executor-memory 5G \
--executor-cores 2 \
--archives hdfs://ns1/user/hadoop/mypy3spark_env/py3spark.tar.gz#py3spark \
--conf "spark.pyspark.python=./py3spark/py3spark/bin/python" \
--conf "spark.pyspark.driver.python=./py3spark/py3spark/bin/python" \
/home/hadoop/hive2iceberg.py ${data_day} ${data_hour}
status=$?
if [ $status = 0 ]; then
echo "STATUS=SUCCESS"
else
echo "STATUS=FAIL"
fi
查询源表里面的最大时间和最小时间,然后将时间切割成多个数据段,于是得到下面的执行脚本,因为上面的shell是循环按年月日小时跑的,所以放在后台执行即可。
nohup ./run_state_hive2iceberg_fixdata.sh 2021050504 2021070100 > 2021070100.log 2>&1 &
nohup ./run_state_hive2iceberg_fixdata.sh 2021070100 2021090100 > 2021090100.log 2>&1 &
nohup ./run_state_hive2iceberg_fixdata.sh 2021090100 2021110100 > 2021110100.log 2>&1 &
nohup ./run_state_hive2iceberg_fixdata.sh 2021110100 2022010100 > 2022010100.log 2>&1 &
nohup ./run_state_hive2iceberg_fixdata.sh 2022010100 2022040100 > 2022040100.log 2>&1 &
nohup ./run_state_hive2iceberg_fixdata.sh 2022040100 2022060100 > 2022060100.log 2>&1 &
nohup ./run_state_hive2iceberg_fixdata.sh 2022060100 2022080100 > 2022080100.log 2>&1 &
nohup ./run_state_hive2iceberg_fixdata.sh 2022080100 2022090220 > 2022090220.log 2>&1 &
自己大概计算了下,一个小时的数据量很小,从hive读取到写入iceberg也就1分钟左右,所以一个小时的时间可以跑数据的大概60个小时。也就是一天可以跑数据的1440个小时,也就是2个月。
那么从2021年5月-2022年9月,大概17个月,我分成了8个任务,每个任务跑2个月,大概全部跑完,也就一天的时间。
而且实际计算了下,使用的总算力比跑一个大任务还少不少。
现在想想有时候真的越笨的方法,越是简单轻松。
好了,任务挂到后台,可以补觉去了。
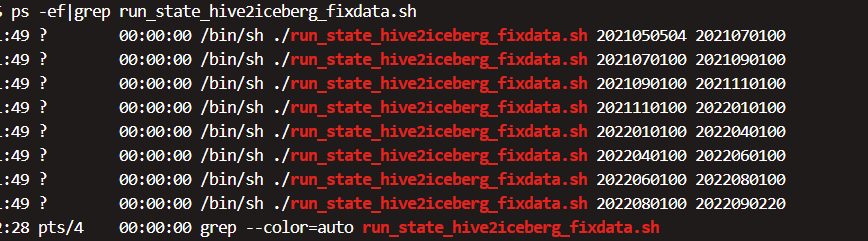
Recommend
About Joyk
Aggregate valuable and interesting links.
Joyk means Joy of geeK