

Deep Learning Opacity In Scientific Discovery
source link: https://arxiv.org/abs/2206.00520
Go to the source link to view the article. You can view the picture content, updated content and better typesetting reading experience. If the link is broken, please click the button below to view the snapshot at that time.
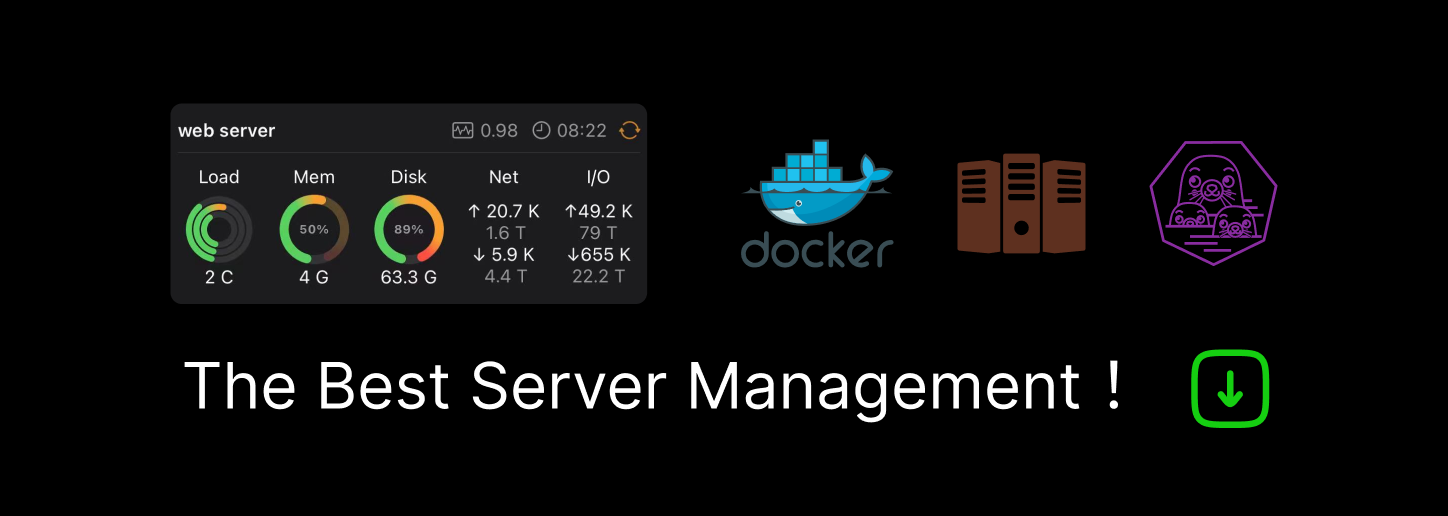
[Submitted on 1 Jun 2022]
Deep Learning Opacity in Scientific Discovery
Philosophers have recently focused on critical, epistemological challenges that arise from the opacity of deep neural networks. One might conclude from this literature that doing good science with opaque models is exceptionally challenging, if not impossible. Yet, this is hard to square with the recent boom in optimism for AI in science alongside a flood of recent scientific breakthroughs driven by AI methods. In this paper, I argue that the disconnect between philosophical pessimism and scientific optimism is driven by a failure to examine how AI is actually used in science. I show that, in order to understand the epistemic justification for AI-powered breakthroughs, philosophers must examine the role played by deep learning as part of a wider process of discovery. The philosophical distinction between the 'context of discovery' and the 'context of justification' is helpful in this regard. I demonstrate the importance of attending to this distinction with two cases drawn from the scientific literature, and show that epistemic opacity need not diminish AI's capacity to lead scientists to significant and justifiable breakthroughs.
Comments: | 12 pages, 1 figure |
Subjects: | Artificial Intelligence (cs.AI); Computers and Society (cs.CY); Machine Learning (cs.LG); Physics and Society (physics.soc-ph) |
Cite as: | arXiv:2206.00520 [cs.AI] |
(or arXiv:2206.00520v1 [cs.AI] for this version) | |
https://doi.org/10.48550/arXiv.2206.00520 |
Recommend
About Joyk
Aggregate valuable and interesting links.
Joyk means Joy of geeK