

GNN 教程:DGL框架-大规模分布式训练
source link: https://archwalker.github.io/blog/2019/07/07/GNN-Framework-DGL-DistributedTraining.html
Go to the source link to view the article. You can view the picture content, updated content and better typesetting reading experience. If the link is broken, please click the button below to view the snapshot at that time.
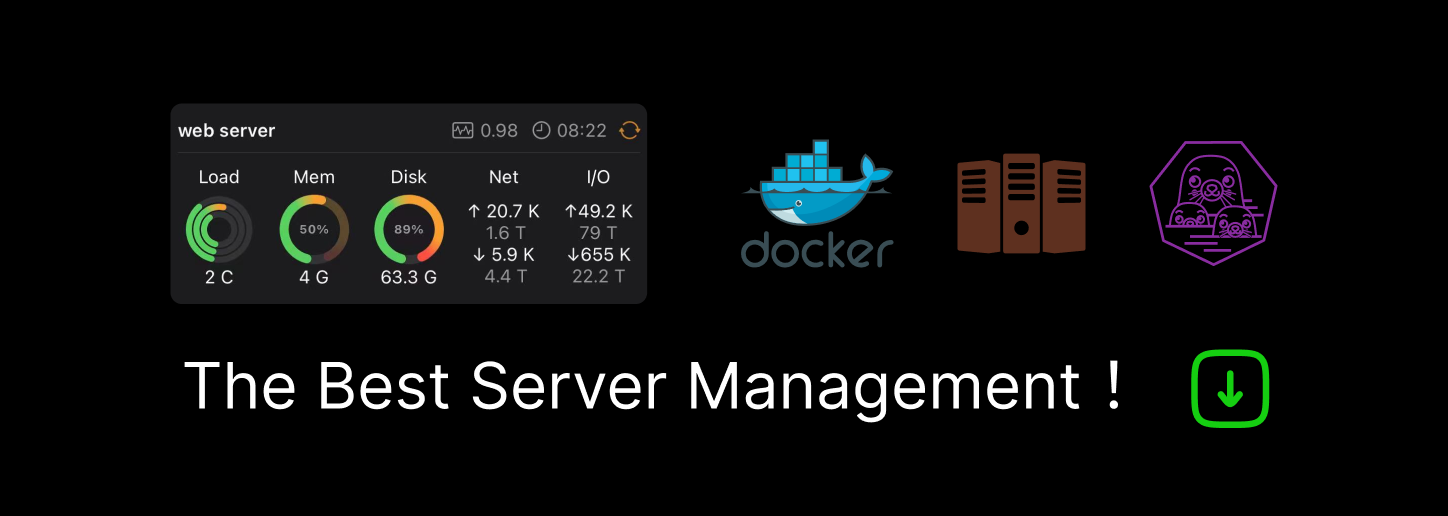
此为原创文章,转载务必保留出处
前面的文章中我们介绍了DGL如何利用采样的技术缩小计算图的规模来通过mini-batch的方式训练模型,当图特别大的时候,非常多的batches需要被计算,因此运算时间又成了问题,一个容易想到解决方案是采用并行计算的技术,很多worker同时采样,计算并且更新梯度。这篇博文重点介绍DGL的并行计算框架。
多进程方案
概括而言,目前DGL(version 0.3)采用的是多进程的并行方案,分布式的方案正在开发中。见下图,DGL的并行计算框架分为两个主要部分:Graph Store
和Sampler
Sampler
被用来从大图中构建许多计算子图(NodeFlow
),DGL能够自动得在多个设备上并行运行多个Sampler
的实例。Graph Store
存储了大图的embedding信息和结构信息,到目前为止,DGL提供了内存共享式的Graph Store,以用来支持多进程,多GPU的并行训练。DGL未来还将提供分布式的Graph Store,以支持超大规模的图训练。
下面来分别介绍它们。
Graph Store
graph store 包含两个部分,server和client,其中server需要作为守护进程(daemon)在训练之前运行起来。比如如下脚本启动了一个graph store server 和 4个worker,并且载入了reddit数据集:
python3 run_store_server.py --dataset reddit --num-workers 4
在训练过程中,这4个worker将会和client交互以取得训练样本。用户需要做的仅仅是编写训练部分的代码。首先需要创建一个client对象连接到对应的server。下面的脚本中用shared_memory
初始化store_type
表明client连接的是一个内存共享式的server。
g = dgl.contrib.graph_store.create_graph_from_store("reddit", store_type="shared_mem")
在采样的博文中,我们已经详细介绍了如何通过采样的技术来减小计算子图的规模。回忆一下,图模型的每一层进行了如下的计算: z(l+1)v=∑u∈N(l)(v)~Auvh(l)uh(l+1)v=σ(z(l+1)vW(l))zv(l+1)=∑u∈N(l)(v)A~uvhu(l)hv(l+1)=σ(zv(l+1)W(l))control-variate sampling用如下的方法近似了z(l+1)vzv(l+1): ^z(l+1)v=|N(v)|∣∣∣^N(l)(v)∣∣∣∑u∈^N(l)(v)~Auv(^h(l)u−¯¯¯h(l)u)+∑u∈N(v)~Auν¯¯¯h(l)u^h(l+1)v=σ(^z(l+1)vW(l))z^v(l+1)=|N(v)||N^(l)(v)|∑u∈N^(l)(v)A~uv(h^u(l)−h¯u(l))+∑u∈N(v)A~uνh¯u(l)h^v(l+1)=σ(z^v(l+1)W(l)) 除了进行这样的近似,作者还采用了预处理的技巧了把采样的层数减少了1。具体来说,GCN的输入是XX的原始embedding,预处理之后GCN的输入是~AXA~X,这种方式使得最早的一层无需进行邻居embedding的融合计算(也就是无需采样),因为左乘以邻接矩阵已经做了这样的计算,因为,需要采样的层数就减少了1。
对于一个大图来说,~AA~和XX都可能很大。两个矩阵的乘法就要通过分布式计算的方式完成,即每一个trainer(worker)负责计算一部分,然后聚合起来。DGL提供了update_all
来进行这种计算:
g.update_all(fn.copy_src(src='features', out='m'),
fn.sum(msg='m', out='preprocess'),
lambda node : {'preprocess': node.data['preprocess'] * node.data['norm']})
初看这段代码和矩阵计算没有任何关系啊,其实这段代码要从语义上理解,在语义上~AXA~X表示邻接矩阵和特征矩阵的乘法,即对于每个节点的特征跟新为邻居特征的和。那么再看上面这段代码就容易了,copy_src
将节点特征取出来,并发送出去, sum
接受到来自邻居的特征并求和,求和结果再发给节点,最后节点自身进行一下renormalize。
update_all
在graph store中是分布式进行的,每个trainer都会分派到一部分节点进行更新。
节点和边的数据现在全部存储在graph store中,因此访问他们不再像以前那样用 g.ndata/g.edata
那样简单,因为这两个方法会读取整个节点和边的数据,而这些数据在graph store中并不存在(他们可能是分开存储的),因此用户只能通过g.nodes[node_ids].data[embed_name]
来访问特定节点的Embedding数据。(注意:这种读数据的方式是通用的,并不是graph store特有的,g.ndata
即是g.nodes[:].data
的缩写)。
为了高效地初始化节点和边tensor,DGL提供了init_ndata
和init_edata
这两种方法。这两种方法都会讲初始化的命令发送到graph store server上,由server来代理初始化工作,下面展示了一个例子:
for i in range(n_layers):
g.init_ndata('h_{}'.format(i), (features.shape[0], args.n_hidden), 'float32')
g.init_ndata('agg_h_{}'.format(i), (features.shape[0], args.n_hidden), 'float32')
其中h_i
存储i
层节点Embedding,agg_h_i
存储i
节点邻居Embedding的聚集后的结果。
初始化节点数据之后,我们可以通过control-variate sampling的方法来训练GCN),这个方法在之前的博文中介绍过
for nf in NeighborSampler(g, batch_size, num_neighbors,
neighbor_type='in', num_hops=L-1,
seed_nodes=labeled_nodes):
for i in range(nf.num_blocks):
# aggregate history on the original graph
g.pull(nf.layer_parent_nid(i+1),
fn.copy_src(src='h_{}'.format(i), out='m'),
lambda node: {'agg_h_{}'.format(i): node.data['m'].mean(axis=1)})
# We need to copy data in the NodeFlow to the right context.
nf.copy_from_parent(ctx=right_context)
nf.apply_layer(0, lambda node : {'h' : layer(node.data['preprocess'])})
h = nf.layers[0].data['h']
for i in range(nf.num_blocks):
prev_h = nf.layers[i].data['h_{}'.format(i)]
# compute delta_h, the difference of the current activation and the history
nf.layers[i].data['delta_h'] = h - prev_h
# refresh the old history
nf.layers[i].data['h_{}'.format(i)] = h.detach()
# aggregate the delta_h
nf.block_compute(i,
fn.copy_src(src='delta_h', out='m'),
lambda node: {'delta_h': node.data['m'].mean(axis=1)})
delta_h = nf.layers[i + 1].data['delta_h']
agg_h = nf.layers[i + 1].data['agg_h_{}'.format(i)]
# control variate estimator
nf.layers[i + 1].data['h'] = delta_h + agg_h
nf.apply_layer(i + 1, lambda node : {'h' : layer(node.data['h'])})
h = nf.layers[i + 1].data['h']
# update history
nf.copy_to_parent()
和原来代码稍有不同的是,这里right_context
表示数据在哪个设备上,通过将数据调度到正确的设备上,我们就可以完成多设备的分布式训练。
Distributed Sampler
因为我们有多个设备可以进行并行计算(比如说多GPU,多CPU),那么需要不断地给每个设备提供nodeflow
(计算子图实例)。DGL采用的做法是分出一部分设备专门负责采样,将采样作为服务提供给计算设备,计算设备只负责在采样后的子图上进行计算。DGL支持同时在多个设备上运行多个采样程序,每个采样程序都可以将采样结果发到计算设备上。
一个分布式采样的示例可以这样写,首先,在训练之前用户需要创建一个分布式SamplerReceiver
对象:
sampler = dgl.contrib.sampling.SamplerReceiver(graph, ip_addr, num_sampler)
SamplerReceiver
类用来从其他设备上接收采样出来的子图,这个API的三个参数分别为parent_graph
, ip_address
, 和number_of_samplers
然后,用户只需要在单机版的训练代码中改变一行:
for nf in sampler:
for i in range(nf.num_blocks):
# aggregate history on the original graph
g.pull(nf.layer_parent_nid(i+1),
fn.copy_src(src='h_{}'.format(i), out='m'),
lambda node: {'agg_h_{}'.format(i): node.data['m'].mean(axis=1)})
...
其中,代码for nf in sampler
用来代替原单机采样代码:
for nf in NeighborSampler(g, batch_size, num_neighbors,
neighbor_type='in', num_hops=L-1,
seed_nodes=labeled_nodes):
其他所有的部分都可以保持不变。
因此,额外的开发工作主要是要编写运行在采样设备上的采样逻辑。对于邻居采样来说,开发者只需要拷贝单机采样的代码就可以了:
sender = dgl.contrib.sampling.SamplerSender(trainer_address)
...
for n in num_epoch:
for nf in dgl.contrib.sampling.NeighborSampler(graph, batch_size, num_neighbors,
neighbor_type='in',
shuffle=shuffle,
num_workers=num_workers,
num_hops=num_hops,
add_self_loop=add_self_loop,
seed_nodes=seed_nodes):
sender.send(nf, trainer_id)
# tell trainer I have finished current epoch
sender.signal(trainer_id)
本篇博文重点介绍了DGL的并行计算框架,其主要由采样层-计算层-存储层三层构建而来,采样和计算分布在不同的机器上,可以并行执行。通过这种方式,在存储充足的情况下,DGL可以处理数以亿计节点和边的大图。
Reference
Recommend
About Joyk
Aggregate valuable and interesting links.
Joyk means Joy of geeK