

推荐系统--FFM模型理论和实践
source link: https://leesen998.github.io/2018/09/15/%E6%8E%A8%E8%8D%90%E7%B3%BB%E7%BB%9F--FFM%E6%A8%A1%E5%9E%8B%E7%90%86%E8%AE%BA%E5%92%8C%E5%AE%9E%E8%B7%B5/
Go to the source link to view the article. You can view the picture content, updated content and better typesetting reading experience. If the link is broken, please click the button below to view the snapshot at that time.
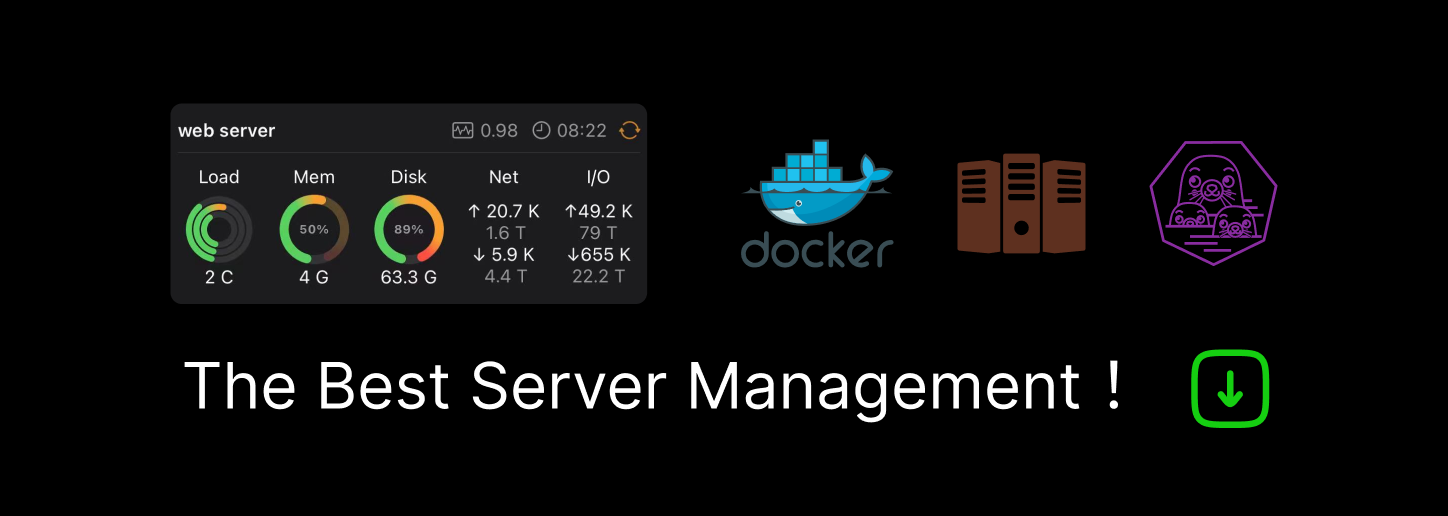
推荐系统--FFM模型理论和实践
推荐系统–FFM模型理论和实践
1、FFM理论
在CTR预估中,经常会遇到one-hot类型的变量,one-hot类型变量会导致严重的数据特征稀疏的情况,为了解决这一问题,在上一讲中,我们介绍了FM算法。这一讲我们介绍一种在FM基础上发展出来的算法-FFM(Field-aware Factorization Machine)。
FFM模型中引入了类别的概念,即field。还是拿上一讲中的数据来讲,先看下图:
在上面的广告点击案例中,“Day=26/11/15”、“Day=1/7/14”、“Day=19/2/15”这三个特征都是代表日期的,可以放到同一个field中。同理,Country也可以放到一个field中。简单来说,同一个categorical特征经过One-Hot编码生成的数值特征都可以放到同一个field,包括用户国籍,广告类型,日期等等。
在FFM中,每一维特征 xi,针对其它特征的每一种field fj,都会学习一个隐向量 v_i,fj。因此,隐向量不仅与特征相关,也与field相关。也就是说,“Day=26/11/15”这个特征与“Country”特征和“Ad_type”特征进行关联的时候使用不同的隐向量,这与“Country”和“Ad_type”的内在差异相符,也是FFM中“field-aware”的由来。
假设样本的 n个特征属于 f个field,那么FFM的二次项有 nf个隐向量。而在FM模型中,每一维特征的隐向量只有一个。FM可以看作FFM的特例,是把所有特征都归属到一个field时的FFM模型。根据FFM的field敏感特性,可以导出其模型方程。
可以看到,如果隐向量的长度为 k,那么FFM的二次参数有 nfk 个,远多于FM模型的 nk个。此外,由于隐向量与field相关,FFM二次项并不能够化简,其预测复杂度是 O(kn^2)。
下面以一个例子简单说明FFM的特征组合方式。输入记录如下:
这条记录可以编码成5个特征,其中“Genre=Comedy”和“Genre=Drama”属于同一个field,“Price”是数值型,不用One-Hot编码转换。为了方便说明FFM的样本格式,我们将所有的特征和对应的field映射成整数编号。
那么,FFM的组合特征有10项,如下图所示。
其中,红色是field编号,蓝色是特征编号。
2、FFM实现细节
这里讲得只是一种FFM的实现方式,并不是唯一的。
损失函数
FFM将问题定义为分类问题,使用的是logistic loss,同时加入了正则项
什么,这是logisitc loss?第一眼看到我是懵逼的,逻辑回归的损失函数我很熟悉啊,不是长这样的啊?其实是我目光太短浅了。逻辑回归其实是有两种表述方式的损失函数的,取决于你将类别定义为0和1还是1和-1。大家可以参考下下面的文章:https://www.cnblogs.com/ljygoodgoodstudydaydayup/p/6340129.html。当我们将类别设定为1和-1的时候,逻辑回归的损失函数就是上面的样子。
随机梯度下降
训练FFM使用的是随机梯度下降方法,即每次只选一条数据进行训练,这里还有必要补一补梯度下降的知识,梯度下降是有三种方式的,截图取自参考文献3:
3、tensorflow实现代码
生成数据
这里我没有找到合适的数据,就自己产生了一点数据,数据涉及20维特征,前十维特征是一个field,后十维是一个field:
def gen_data():
labels = [-1,1]
y = [np.random.choice(labels,1)[0] for _ in range(all_data_size)]
x_field = [i // 10 for i in range(input_x_size)]
x = np.random.randint(0,2,size=(all_data_size,input_x_size))
return x,y,x_field
定义权重项
在ffm中,有三个权重项,首先是bias,然后是一维特征的权重,最后是交叉特征的权重:
def createTwoDimensionWeight(input_x_size,field_size,vector_dimension):
weights = tf.truncated_normal([input_x_size,field_size,vector_dimension])
tf_weights = tf.Variable(weights)
return tf_weights
def createOneDimensionWeight(input_x_size):
weights = tf.truncated_normal([input_x_size])
tf_weights = tf.Variable(weights)
return tf_weights
def createZeroDimensionWeight():
weights = tf.truncated_normal([1])
tf_weights = tf.Variable(weights)
return tf_weights
计算估计值
估计值的计算这里不能项FM一样先将公式化简再来做,对于交叉特征,只能写两重循环,所以对于特别多的特征的情况下,真的计算要爆炸呀!
def inference(input_x,input_x_field,zeroWeights,oneDimWeights,thirdWeight):
"""计算回归模型输出的值"""
secondValue = tf.reduce_sum(tf.multiply(oneDimWeights,input_x,name='secondValue'))
firstTwoValue = tf.add(zeroWeights, secondValue, name="firstTwoValue")
thirdValue = tf.Variable(0.0,dtype=tf.float32)
input_shape = input_x_size
for i in range(input_shape):
featureIndex1 = I
fieldIndex1 = int(input_x_field[I])
for j in range(i+1,input_shape):
featureIndex2 = j
fieldIndex2 = int(input_x_field[j])
vectorLeft = tf.convert_to_tensor([[featureIndex1,fieldIndex2,i] for i in range(vector_dimension)])
weightLeft = tf.gather_nd(thirdWeight,vectorLeft)
weightLeftAfterCut = tf.squeeze(weightLeft)
vectorRight = tf.convert_to_tensor([[featureIndex2,fieldIndex1,i] for i in range(vector_dimension)])
weightRight = tf.gather_nd(thirdWeight,vectorRight)
weightRightAfterCut = tf.squeeze(weightRight)
tempValue = tf.reduce_sum(tf.multiply(weightLeftAfterCut,weightRightAfterCut))
indices2 = [I]
indices3 = [j]
xi = tf.squeeze(tf.gather_nd(input_x, indices2))
xj = tf.squeeze(tf.gather_nd(input_x, indices3))
product = tf.reduce_sum(tf.multiply(xi, xj))
secondItemVal = tf.multiply(tempValue, product)
tf.assign(thirdValue, tf.add(thirdValue, secondItemVal))
return tf.add(firstTwoValue,thirdValue)
定义损失函数
损失函数我们就用逻辑回归损失函数来算,同时加入正则项:
lambda_w = tf.constant(0.001, name='lambda_w')
lambda_v = tf.constant(0.001, name='lambda_v')
zeroWeights = createZeroDimensionWeight()
oneDimWeights = createOneDimensionWeight(input_x_size)
thirdWeight = createTwoDimensionWeight(input_x_size, # 创建二次项的权重变量
field_size,
vector_dimension) # n * f * k
y_ = inference(input_x, trainx_field,zeroWeights,oneDimWeights,thirdWeight)
l2_norm = tf.reduce_sum(
tf.add(
tf.multiply(lambda_w, tf.pow(oneDimWeights, 2)),
tf.reduce_sum(tf.multiply(lambda_v, tf.pow(thirdWeight, 2)),axis=[1,2])
)
)
loss = tf.log(1 + tf.exp(input_y * y_)) + l2_norm
train_step = tf.train.GradientDescentOptimizer(learning_rate=lr).minimize(loss)
训练
接下来就是训练了,每次只用喂一个数据就好:
input_x_batch = trainx[t]
input_y_batch = trainy[t]
predict_loss,_, steps = sess.run([loss,train_step, global_step],
feed_dict={input_x: input_x_batch, input_y: input_y_batch})
Recommend
About Joyk
Aggregate valuable and interesting links.
Joyk means Joy of geeK