

微信服务号爬虫
source link: http://fancyerii.github.io/2021/09/09/wechat-fuwuhao-crawler/
Go to the source link to view the article. You can view the picture content, updated content and better typesetting reading experience. If the link is broken, please click the button below to view the snapshot at that time.
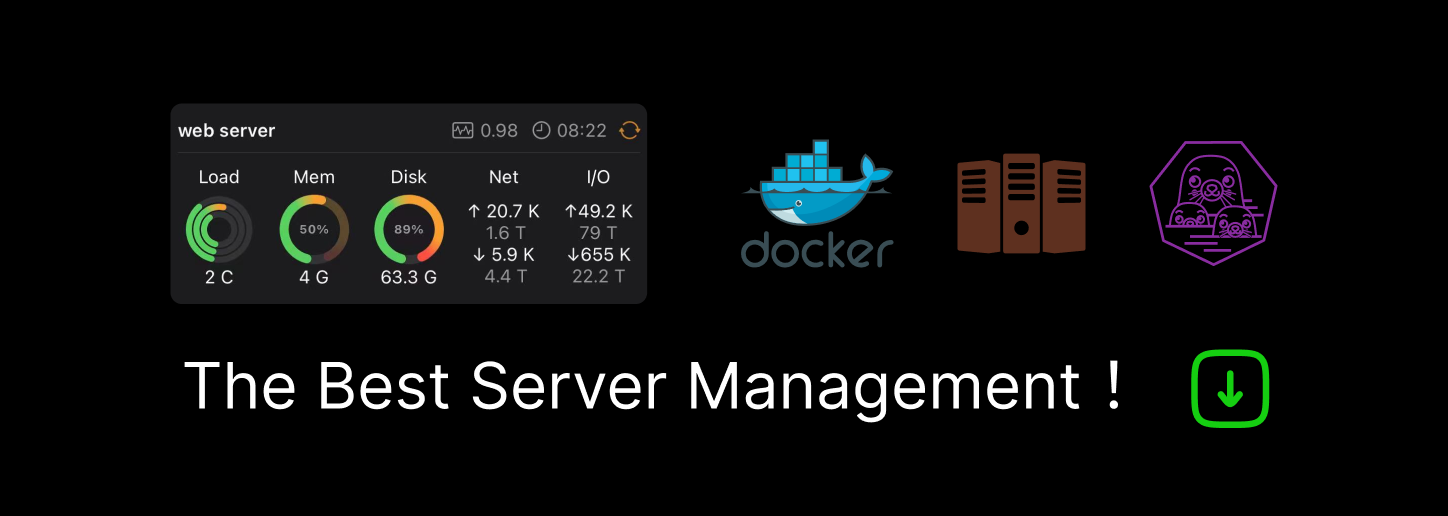
微信服务号爬虫 - 李理的博客
微信公众号爬虫v1.2实现了订阅号的抓取,但是没有实现服务号的抓取,原因是服务号的页面无法通过pywinauto得到页面的信息,从而无法模拟人的操作点击文章。本文通过视觉的页面分析,让爬虫模拟人类分析出文章的位置,从而实现服务号抓取。
目录
订阅号与服务号的区别
微信公众号分为订阅号与服务号,根据申请的主题不同又分为普通订阅号、普通服务号、认证订阅号和认证服务号。认证的订阅号/服务号权限更大,但是对于爬虫来说我们只需要分为订阅号和服务号就行。订阅号和服务号的详细区别请参考微信公众号:订阅号与服务号的区别对比。不过从爬虫的角度来说,它们最主要的区别如下在于其消息展示方式不同,从而页面不同。
订阅号
服务号
我们可以看到,所有的订阅号会集合在一起展示。比如”新智元”这个订阅号,展示分为三个区域:”订阅号”->”新智元”->列表页。点击列表页中的一篇文章会弹出一个浏览器窗口,这是我们之前的抓取方式:不断用PageDown翻页,点击打开浏览器,抓取,关闭浏览器……。
而服务号是单独展示的,要查看历史消息,我们需要点击右上角的”…“按钮,从而弹出列表页。而且点击这个列表页的文章时不会弹出新的窗口,而是在当前窗口打开,因此返回列表页不能关闭窗口,而是要点击后退按钮,如下图所示:
服务号文章是在列表页直接打开
通过后退按钮返回列表页
服务号列表页分析
我们前面抓取订阅号时,可以直接通过pywinauto得到列表页中每篇文章的block的坐标信息,从而可以控制爬虫模拟进行点击。但是对于服务号,没有办法得到正确的坐标信息。我不知道是什么原因,开了个issue也没解决。当然,对于人类来说,我们并不需要知道这些metadata,而是通过视觉的分析判断某一块区域就是一篇文章,从而点击这篇文章。而我们这篇文章主要解决的问题,也就是对服务号内容列表页进行页面分析,定位每篇文章。
尝试过的方法
下面是待分析的服务号列表页内容:
页面布局分析
从问题来说,这是一个页面布局分析问题。因为最近看到一篇论文和它开源的代码,于是拿来试试。论文题为LayoutParser: A Unified Toolkit for DeepLearning Based Document Image Analysis,简单的试了一下,效果并不理想:
import layoutparser as lp
import cv2
#image = cv2.imread("a.png")
image = cv2.imread("paper.png")
image = image[..., ::-1]
model = lp.Detectron2LayoutModel('lp://PubLayNet/faster_rcnn_R_50_FPN_3x/config',
extra_config=["MODEL.ROI_HEADS.SCORE_THRESH_TEST", 0.8],
label_map={0: "Text", 1: "Title", 2: "List", 3:"Table", 4:"Figure"})
layout = model.detect(image)
lp.draw_box(image, layout, box_width=3)
cv2.imwrite("lp.png", image)
PubLayNet模型应该只是用论文的数据训练的,因此效果不好,完全没有分析出来。
tesseract引擎
ocr一般分为detection和recognition两个步骤。我们前面使用tesseract来抽取阅读数时使用的单行模式,跳过了检测步骤,我们也可以让tesseract来做检测然后识别:
from pytesseract import Output
import pytesseract
import argparse
import cv2
ap = argparse.ArgumentParser()
ap.add_argument("-i", "--image", required=True,
help="path to input image to be OCR'd")
ap.add_argument("-c", "--min-conf", type=int, default=0,
help="mininum confidence value to filter weak text detection")
args = vars(ap.parse_args())
options = "-l {} --psm {}".format("chi_sim", "3")
image = cv2.imread(args["image"])
rgb = cv2.cvtColor(image, cv2.COLOR_BGR2RGB)
results = pytesseract.image_to_data(rgb,
output_type=Output.DICT,
config=options)
for i in range(0, len(results["text"])):
# extract the bounding box coordinates of the text region from
# the current result
x = results["left"][i]
y = results["top"][i]
w = results["width"][i]
h = results["height"][i]
text = results["text"][i]
conf = int(results["conf"][i])
cv2.rectangle(image, (x, y), (x+w, y+h), (0, 255, 0), 2)
cv2.imwrite("tess.png", image)
调用pytesseract.image_to_data方法可以得到除了文本的其它信息:boundingbox(left,top,width,height)还有置信度得分。结果如下图:
tessearct的分析结果
可以发现效果很不好,首先日期没有被检测出来(这是我们后面定位的关键稳定特征);而且汉字块都不连续。个人猜测tesseract虽然识别有中文的模型(效果也一般),但是检测可能还是英文的数据,所以效果不好。
EasyOCR
EasyOCR是一个非常流行的OCR工具,支持多种语言,而且还有一个Web的Demo,因此我也尝试了一下,结果如下:
EasyOCR检测结果
EasyOCR识别结果
可以看到EasyOCR的检测结果还行,识别也勉强,但是识别日期问题很大,”x年x月x日”基本都识别错了,我猜测是语言模型的问题。
East算法
EAST: An Efficient and Accurate Scene Text Detector是OpenCV提供的场景文字检测模型,不了解的读者可以阅读论文或者参考OpenCV Text Detection (EAST text detector)或者Deep Learning based Text Detection Using OpenCV (C++/Python)。代码参考的是第一篇文章。首先是east.py:
import numpy as np
EAST_OUTPUT_LAYERS = [
"feature_fusion/Conv_7/Sigmoid",
"feature_fusion/concat_3"]
def decode_predictions(scores, geometry, minConf=0.5):
# grab the number of rows and columns from the scores volume, then
# initialize our set of bounding box rectangles and corresponding
# confidence scores
(numRows, numCols) = scores.shape[2:4]
rects = []
confidences = []
# loop over the number of rows
for y in range(0, numRows):
# extract the scores (probabilities), followed by the
# geometrical data used to derive potential bounding box
# coordinates that surround text
scoresData = scores[0, 0, y]
xData0 = geometry[0, 0, y]
xData1 = geometry[0, 1, y]
xData2 = geometry[0, 2, y]
xData3 = geometry[0, 3, y]
anglesData = geometry[0, 4, y]
# loop over the number of columns
for x in range(0, numCols):
# grab the confidence score for the current detection
score = float(scoresData[x])
# if our score does not have sufficient probability,
# ignore it
if score < minConf:
continue
# compute the offset factor as our resulting feature
# maps will be 4x smaller than the input image
(offsetX, offsetY) = (x * 4.0, y * 4.0)
# extract the rotation angle for the prediction and
# then compute the sin and cosine
angle = anglesData[x]
cos = np.cos(angle)
sin = np.sin(angle)
h = xData0[x] + xData2[x]
w = xData1[x] + xData3[x]
offset = ([
offsetX + (cos * xData1[x]) + (sin * xData2[x]),
offsetY - (sin * xData1[x]) + (cos * xData2[x])])
# derive the top-right corner and bottom-right corner of
# the rotated bounding box
topLeft = ((-sin * h) + offset[0], (-cos * h) + offset[1])
topRight = ((-cos * w) + offset[0], (sin * w) + offset[1])
# compute the center (x, y)-coordinates of the rotated
# bounding box
cX = 0.5 * (topLeft[0] + topRight[0])
cY = 0.5 * (topLeft[1] + topRight[1])
# our rotated bounding box information consists of the
# center (x, y)-coordinates of the box, the width and
# height of the box, as well as the rotation angle
box = ((cX, cY), (w, h), -1 * angle * 180.0 / np.pi)
# update our detections and confidences lists
rects.append(box)
confidences.append(score)
return (rects, confidences)
然后是测试代码localize_text_opencv.py:
# import the necessary packages
from east import EAST_OUTPUT_LAYERS
from east import decode_predictions
import numpy as np
import argparse
import time
import cv2
ap = argparse.ArgumentParser()
ap.add_argument("-i", "--image", required=True,
help="path to input image")
ap.add_argument("-e", "--east", required=True,
help="path to input EAST text detector")
ap.add_argument("-w", "--width", type=int, default=320,
help="resized image width (should be multiple of 32)")
ap.add_argument("-t", "--height", type=int, default=320,
help="resized image height (should be multiple of 32)")
ap.add_argument("-c", "--min-conf", type=float, default=0.5,
help="minimum probability required to inspect a text region")
ap.add_argument("-n", "--nms-thresh", type=float, default=0.4,
help="non-maximum suppression threshold")
args = vars(ap.parse_args())
image = cv2.imread(args["image"])
(origH, origW) = image.shape[:2]
# set the new width and height and then determine the ratio in change
# for both the width and height
(newW, newH) = (args["width"], args["height"])
rW = origW / float(newW)
rH = origH / float(newH)
print("[INFO] loading EAST text detector...")
net = cv2.dnn.readNet(args["east"])
blob = cv2.dnn.blobFromImage(image, 1.0, (newW, newH),
(123.68, 116.78, 103.94), swapRB=True, crop=False)
start = time.time()
net.setInput(blob)
(scores, geometry) = net.forward(EAST_OUTPUT_LAYERS)
end = time.time()
print("[INFO] text detection took {:.6f} seconds".format(end - start))
# decode the predictions form OpenCV's EAST text detector and then
# apply non-maximum suppression (NMS) to the rotated bounding boxes
(rects, confidences) = decode_predictions(scores, geometry,
minConf=args["min_conf"])
idxs = cv2.dnn.NMSBoxesRotated(rects, confidences,
args["min_conf"], args["nms_thresh"])
# ensure that at least one text bounding box was found
if len(idxs) > 0:
# loop over the valid bounding box indexes after applying NMS
for i in idxs.flatten():
# compute the four corners of the bounding box, scale the
# coordinates based on the respective ratios, and then
# convert the box to an integer NumPy array
box = cv2.boxPoints(rects[i])
box[:, 0] *= rW
box[:, 1] *= rH
box = np.int0(box)
cv2.polylines(image, [box], True, (0, 255, 0), 2)
cv2.imwrite("east.png", image)
模型可以去这里下载,然后运行:
python localize_text_opencv.py -i a.png -e frozen_east_text_detection.pb
结果如下:
EAST算法的检测结果
日期依然没有检测出来!
PaddleOCR
这里参考了PaddleHub一键OCR中文识别(超轻量8.1M模型,火爆)(2020/09/21更新版),代码如下:
import matplotlib.pyplot as plt
import matplotlib.image as mpimg
# 待预测图片
test_img_path = ["./example.jpg"]
# 展示其中广告信息图片
img1 = mpimg.imread(test_img_path[0])
plt.figure(figsize=(10,10))
plt.imshow(img1)
plt.axis('off')
plt.show()
import paddlehub as hub
#ocr = hub.Module(name="chinese_ocr_db_crnn_mobile")
ocr = hub.Module(name="chinese_ocr_db_crnn_server")
import cv2
# 读取测试文件夹test.txt中的照片路径
np_images =[cv2.imread(image_path) for image_path in test_img_path]
results = ocr.recognize_text(
images=np_images, # 图片数据,ndarray.shape 为 [H, W, C],BGR格式;
use_gpu=True, # 是否使用 GPU;若使用GPU,请先设置CUDA_VISIBLE_DEVICES环境变量
output_dir='ocr_result', # 图片的保存路径,默认设为 ocr_result;
visualization=True, # 是否将识别结果保存为图片文件;
box_thresh=0.5, # 检测文本框置信度的阈值;
text_thresh=0.5) # 识别中文文本置信度的阈值;
for result in results:
data = result['data']
save_path = result['save_path']
for infomation in data:
print('text: ', infomation['text'], '\nconfidence: ', infomation['confidence'], '\ntext_box_position: ', infomation['text_box_position'])
效果如下:
PaddleOCR的结果
可以发现它的效果非常好,检测和识别都非常准确。
实际解决方法
从上面的调研来看,使用PaddleOCR应该可以满足我们的需求,但是要使用PaddleOCR需要安装PaddlePaddle框架,而且也需要一定的计算资源。对于上面的问题,我们其实注意能够定位到“X年X月X日”就可以了,因此我最终使用的是OpenCV里非常简单的图像处理技巧来定位,然后用tesseract来识别日期(因为我们已经引入了tesseract,如果之前没有一个ocr引擎,也许可以考虑PaddleOCR,不过我们只是用ocr识别数字和日期,因此tesseract也勉强够用了)。
检测的代码如下:
# Import required packages
import cv2
import pytesseract
# Read image from which text needs to be extracted
img = cv2.imread("a.png")
# Preprocessing the image starts
# Convert the image to gray scale
gray = cv2.cvtColor(img, cv2.COLOR_BGR2GRAY)
# Performing OTSU threshold
ret, thresh1 = cv2.threshold(gray, 200, 255, cv2.THRESH_BINARY_INV)
# Specify structure shape and kernel size.
# Kernel size increases or decreases the area
# of the rectangle to be detected.
# A smaller value like (10, 10) will detect
# each word instead of a sentence.
rect_kernel = cv2.getStructuringElement(cv2.MORPH_RECT, (12, 12))
# Appplying dilation on the threshold image
dilation = cv2.dilate(thresh1, rect_kernel, iterations=1)
# Finding contours
contours, hierarchy = cv2.findContours(dilation, cv2.RETR_EXTERNAL,
cv2.CHAIN_APPROX_NONE)
# Creating a copy of image
im2 = img.copy()
# Looping through the identified contours
# Then rectangular part is cropped and passed on
# to pytesseract for extracting text from it
# Extracted text is then written into the text file
for cnt in contours:
x, y, w, h = cv2.boundingRect(cnt)
# Drawing a rectangle on copied image
rect = cv2.rectangle(im2, (x, y), (x + w, y + h), (0, 255, 0), 2)
cv2.imshow("Text Detection", im2)
cv2.waitKey(0)
最终检测的结果如下图所示:
检测结果
检测到了之后我们可以遍历所有的文本框,使用tesseract识别出日期。
def locate_articles(pil_img_array, kernel=12, thrshold=200, x_max=50):
img = cv2.cvtColor(pil_img_array, cv2.COLOR_RGB2BGR)
gray = cv2.cvtColor(img, cv2.COLOR_BGR2GRAY)
ret, thresh1 = cv2.threshold(gray, thrshold, 255, cv2.THRESH_BINARY_INV)
rect_kernel = cv2.getStructuringElement(cv2.MORPH_RECT, (kernel, kernel))
# Appplying dilation on the threshold image
dilation = cv2.dilate(thresh1, rect_kernel, iterations=1)
# Finding contours
contours, hierarchy = cv2.findContours(dilation, cv2.RETR_EXTERNAL,
cv2.CHAIN_APPROX_NONE)
contours = sort_contours(contours, method="top-to-bottom")[0]
date_list = []
for cnt in contours:
x, y, w, h = cv2.boundingRect(cnt)
if x < x_max and w > 20:
region = Image.fromarray(pil_img_array[y:y+h, x:x+w])
text = _clear_text(ocr(region))
if not _is_date(text):
continue
date_list.append((x, y, w, h))
return date_list
我们通过imutils.sort_contours对文本框从上到下排序,而且根据先验知识,我们知道日期块的比较靠左边,所以可以进行一下过滤,然后用tesseract进行ocr,通过正则表达式判断是否日期。imutils需要使用pip install imutils安装。
Recommend
About Joyk
Aggregate valuable and interesting links.
Joyk means Joy of geeK