

Warmup小记 - 鱼与鱼
source link: https://www.cnblogs.com/gongyanzh/p/16126287.html
Go to the source link to view the article. You can view the picture content, updated content and better typesetting reading experience. If the link is broken, please click the button below to view the snapshot at that time.
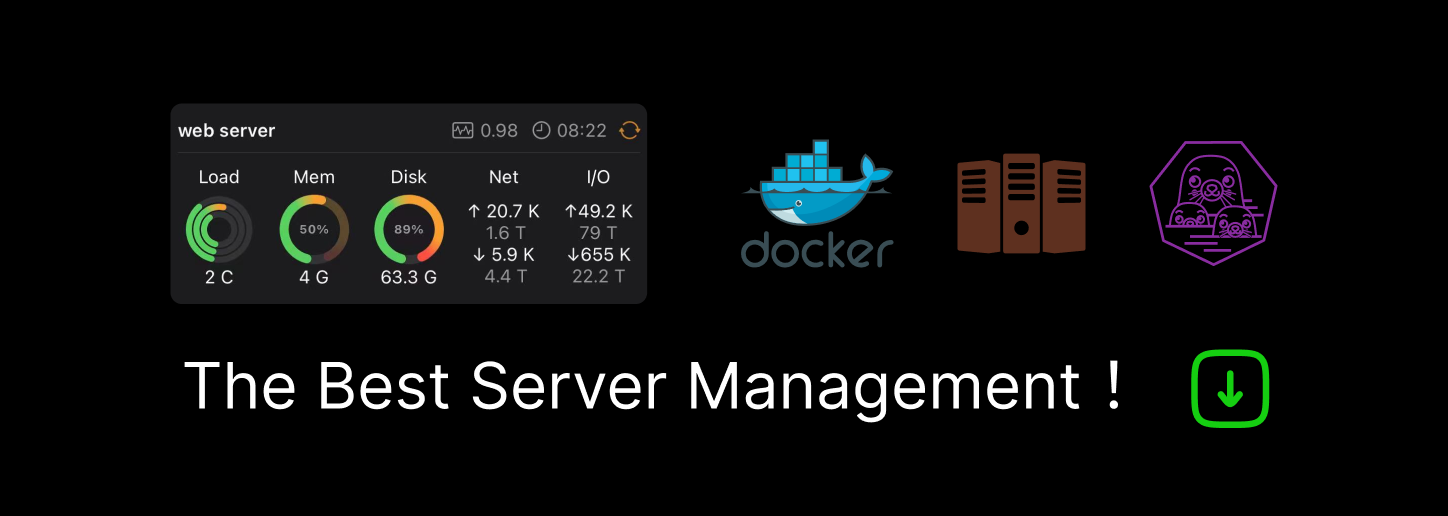
什么是warmup
热身,在刚刚开始训练时以很小的学习率进行训练,使得网络熟悉数据,随着训练的进行学习率慢慢变大,到了一定程度,以设置的初始学习率进行训练,接着过了一些inter后,学习率再慢慢变小;
学习率变化:上升——平稳——下降
为什么用warmup
- 有助于减缓模型在初始阶段对mini-batch的提前过拟合现象,保持分布的平稳
- 有助于保持模型深层的稳定性
可以认为,刚开始模型对数据的“分布”理解为零,或者是说“均匀分布”(当然这取决于你的初始化);在第一轮训练的时候,每个数据点对模型来说都是新的,模型会很快地进行数据分布修正,如果这时候学习率就很大,极有可能导致开始的时候就对该数据“过拟合”,后面要通过多轮训练才能拉回来,浪费时间。当训练了一段时间(比如两轮、三轮)后,模型已经对每个数据点看过几遍了,或者说对当前的batch而言有了一些正确的先验,较大的学习率就不那么容易会使模型学偏,所以可以适当调大学习率。这个过程就可以看做是warmup。
当模型训到一定阶段后(比如十个epoch),模型的分布就已经比较固定了,或者说能学到的新东西就比较少了。如果还沿用较大的学习率,就会破坏这种稳定性,用我们通常的话说,就是已经接近loss的local optimal了,为了靠近这个point,我们就要慢慢来。
这里只摘录了一小段,参考文献 [1] 解释的很好。
learning rate schedule
warmup和learning schedule是类似的,只是学习率变化不同。如图
learning rate schedule
tensorflow 中有几种不同的learning rate schedule,以上图的3种为例,更多schedule可以直达官网
# CosineDecay
cosine_learning_rate_schedule = tf.keras.optimizers.schedules.CosineDecay(0.001,4000)
plt.plot(cosine_learning_rate_schedule(tf.range(40000, dtype=tf.float32)),label="cosine")
# ExponentialDecay
exp_learning_rate_schedule = tf.keras.optimizers.schedules.ExponentialDecay(
0.001, 4000, 0.9, staircase=False, name=None
)
plt.plot(exp_learning_rate_schedule(tf.range(40000, dtype=tf.float32)),label="exp")
# PiecewiseConstantDecay
boundaries = [10000, 20000,30000]
values = [0.001, 0.0008, 0.0004,0.0001]
piecewise_learning_rate_schedule = tf.keras.optimizers.schedules.PiecewiseConstantDecay(
boundaries, values)
plt.plot([piecewise_learning_rate_schedule(step) for step in tf.range(40000, dtype=tf.float32)],label="piecewise")
# 自定义 Schedule
my_learning_rate_schedule = MySchedule(0.001)
plt.plot([my_learning_rate_schedule(step) for step in tf.range(40000, dtype=tf.float32)],label="warmup")
plt.title("Learning rate schedule")
plt.ylabel("Learning Rate")
plt.xlabel("Train Step")
plt.legend()
# 自定义 Schedule
class MySchedule(tf.keras.optimizers.schedules.LearningRateSchedule):
def __init__(self, initial_learning_rate, warmup_steps=4000):
super(MySchedule, self).__init__()
self.initial_learning_rate = initial_learning_rate
self.warmup_steps = warmup_steps
def __call__(self, step):
if step > self.warmup_steps:
return self.initial_learning_rate * self.warmup_steps * step ** -1
else:
return self.initial_learning_rate * step * (self.warmup_steps ** -1)
warmup in transformer
Noam Optimizer
class CustomSchedule(tf.keras.optimizers.schedules.LearningRateSchedule):
def __init__(self, d_model, warmup_steps=4000):
super(CustomSchedule, self).__init__()
self.d_model = d_model
self.d_model = tf.cast(self.d_model, tf.float32)
self.warmup_steps = warmup_steps
def __call__(self, step):
arg1 = tf.math.rsqrt(step)
arg2 = step * (self.warmup_steps ** -1.5)
return tf.math.rsqrt(self.d_model) * tf.math.minimum(arg1, arg2)
learning_rate = CustomSchedule(d_model)
optimizer = tf.keras.optimizers.Adam(learning_rate, beta_1=0.9, beta_2=0.98,
epsilon=1e-9)
temp_learning_rate_schedule = CustomSchedule(128)
plt.plot(temp_learning_rate_schedule(tf.range(40000, dtype=tf.float32)))
plt.ylabel("Learning Rate")
plt.xlabel("Train Step")
关于warmup参数
一般可取训练steps的10%,参考BERT。这里可以根据具体任务进行调整,主要需要通过warmup来使得学习率可以适应不同的训练集合,另外我们也可以通过训练误差观察loss抖动的关键位置,找出合适的学习率。[4]
references
【1】神经网络中 warmup 策略为什么有效;有什么理论解释么? - 香侬科技的回答 - 知乎 https://www.zhihu.com/question/338066667/answer/771252708
【2】tf官方文档 tf.keras.optimizers.schedules. https://www.tensorflow.org/versions/r2.6/api_docs/python/tf/keras/optimizers/schedules
【4】聊一聊学习率预热linear warmup. https://cloud.tencent.com/developer/article/1929850
Recommend
About Joyk
Aggregate valuable and interesting links.
Joyk means Joy of geeK