

Continuous Feedback: Taking AI From Good To Great - DZone AI
source link: https://dzone.com/articles/continuous-feedback-is-key-to-taking-your-ai-from
Go to the source link to view the article. You can view the picture content, updated content and better typesetting reading experience. If the link is broken, please click the button below to view the snapshot at that time.
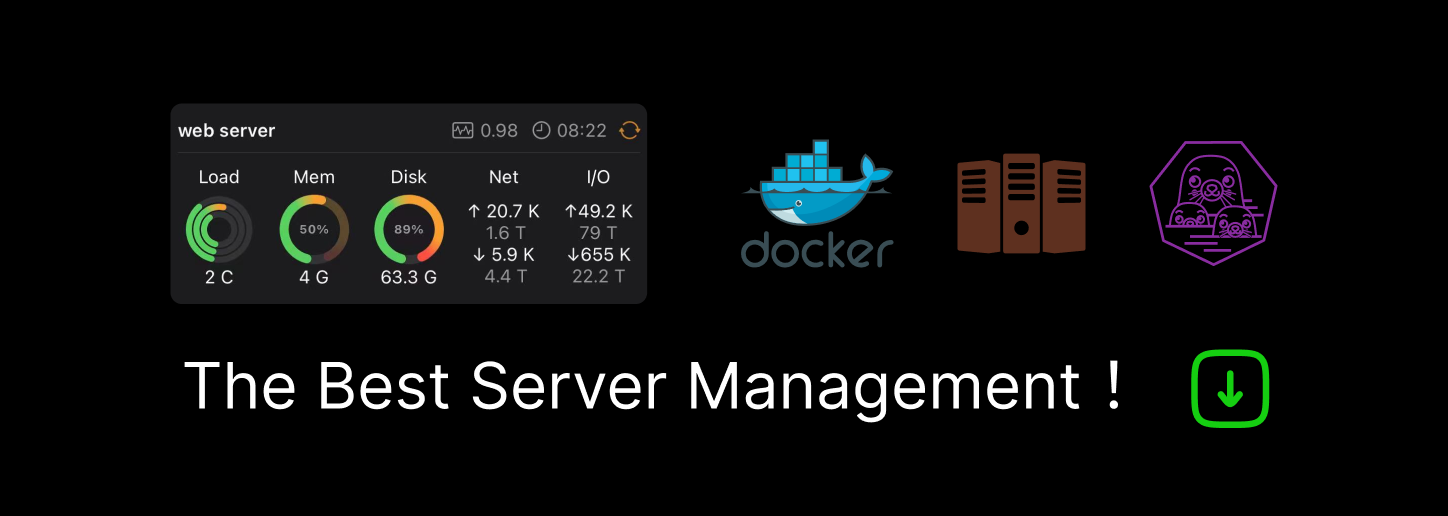
Continuous Feedback Is Key To Taking Your AI From “Good to Great”
Optimizing AI systems has many challenges. The secret to success is continuous feedback, which bridges the gap between business outcomes and AI workflows.
Join the DZone community and get the full member experience.
Join For FreeDeploying AI instantly brought value and growth to many businesses. However, it is well established that sustaining the value over time, not to mention maximizing it, could be quite challenging. Continuous optimization is the key to successful AI deployments. Begin with a product that’s good enough, learn from how it performs in the real world (especially as the data environment changes), and then improve; then learn and improve again, and so on. It’s a bit of an obvious insight, but it is rare for AI-driven products to be perfect from day one.
To accomplish continuous optimization you need continuous feedback. You need “eyes and ears” observing your data and models and telling you whether they’re performing well. That’s easier said than done, for various reasons. These reasons are outlined below.
Before we go there, let’s first establish what we mean by continuous feedback. It is bridging the gap between business outcomes and AI workflows. Are you able to demonstrate how your AI is impacting business KPIs? How about how changes in your AI are impacting changes in the business KPIs? If there are changes in business KPIs that are rooted in changes in your AI, can you detect that? If the answers are YES, then you have continuous feedback.
Now, back to the challenges of attaining continuous feedback for your AI.
1. Defining the Desired Outcomes
First, you need a clear definition of what success looks like, and it’s not always obvious. For example, two recommendation systems may look very similar; but in fact, they have very different strategic objectives. On one hand, the goal is to drive immediate conversions, like you would on an eCommerce website. On the other hand, it is to drive general customer satisfaction, such as content suggestions from a streaming service. It’s not always the easiest, but it is critical to measure your AI in general, based on indicators of the main objective.
2. Disparate Systems
Once the business indicators (of the desired outcomes) are well defined, you need to track them and repeatedly analyze them in the context of changes in your AI (data, features, inferences, etc). A common challenge around doing that is that the business indicators “live” in a different environment, outside of the AI stack. For instance, in an eCommerce recommendation system, the business indicators may be clicks and conversions and these are tracked in your marketing stack. Can the AI team access these indicators easily and consistently for continuous feedback? In our experience, not very often.
3. Timing
In some cases, the business indicators are well defined, and the AI team can access them, but the challenge is timing. This means that the indicators are measured at a later time, and are not available when (or shortly after) your AI is at play. An example of this is credit models. A prediction of an individual’s creditworthiness is used to automatically approve a loan or line of credit. Then, it could be months or even years before the accuracy of that credit risk prediction can be determined. In other cases, the lag time may not be as extreme (maybe just days or weeks), so it will be worth designing a mechanism and implementing an integration that will provide that feedback once the data is available. It is important to plan for timing challenges in which it can be mitigated through partial feedback (a subset of data to feed the system), manual feedback (adding a human into the loop), or proxy feedback (e.g., shrinking confidence scores).
4. Expensive
The last challenge that we will outline for continuous feedback is that in some cases it can be very expensive to obtain the indicators of success. What we mean here is that often the indicator of success requires a human in the loop. An annotator to provide a “ground truth” label. Labeling data may require expertise (e.g., medical experts to verify the read of an X-ray) or simply work hours (going through a million images), either of which could prove costly.
Conclusion
Continuous feedback will improve your AI over time and accelerate business value, giving you confidence in your data pipelines, inference engines, and your model environment overall. While it’s not always easy to achieve, forward-thinking AI teams see continuous feedback as a critical element of production-grade AI and work to institute it.
Recommend
About Joyk
Aggregate valuable and interesting links.
Joyk means Joy of geeK