

【Matlab工具箱】感知机
source link: https://www.guofei.site/2016/05/06/MatlabToolboxPerceptron.html
Go to the source link to view the article. You can view the picture content, updated content and better typesetting reading experience. If the link is broken, please click the button below to view the snapshot at that time.
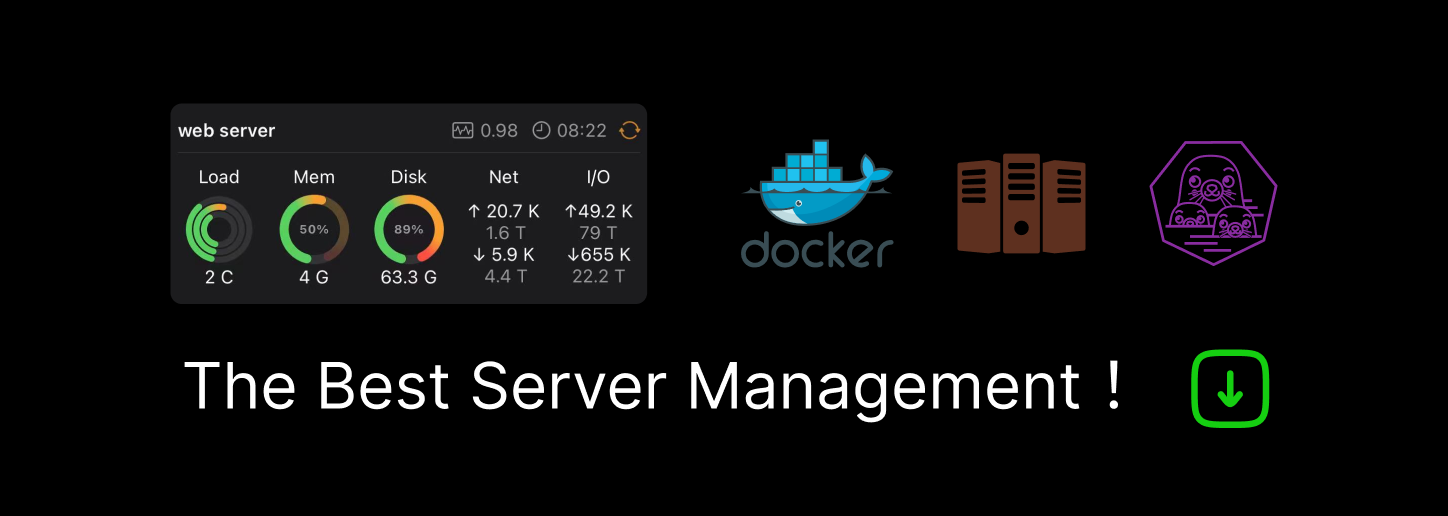
【Matlab工具箱】感知机
2016年05月06日Author: Guofei
文章归类: 趣文,文章编号:
版权声明:本文作者是郭飞。转载随意,但需要标明原文链接,并通知本人
原文链接:https://www.guofei.site/2016/05/06/MatlabToolboxPerceptron.html
这里是Matlab自带的感知机工具箱介绍
1、生成
newp生成
net=newp(P,T,TF,LF)%
P=[-1 1;-1 1];%输入向量的范围,是一个R*2矩阵,R表示向量维度
T=1; %输出节点个数
TF='hardlim'; %传输函数,可取值为hardlim(默认),hardlims
LF='learnp'; %学习函数,可取值为learnp(默认)或learnpn; learnpn比learp对输入量大小的变化不敏感,
hardlim(x)=1(x≥0),0(x<0)
hardlims(x)=1(x≥0),-1(x<0)
init:初始化
net=init(net)
2、训练
train:会出图
[net,tr]=train(net,P,T,Pi,Ai)
P:网络输入
T:网络期望输出
Pi:初始输入延迟
Ai:初始层延迟
tr:训练记录,包括部数epoch和性能perf
adapt:神经网络的自适应
[net,Y,E,Pf,Af,tr]=adapt(net,P,T,Pi,Ai)
3、仿真:sim
[Y,Pf,Af,E,perf]=sim(net,P,Pi,Ai)
Pf:最终输出延迟
Af:最终层延迟
E:网络的误差
perf:网络的性能(Performance)
4、评价
mae:平均绝对误差性能函数 Mean Absolute Error
net.performFcn
net.performParam
perf=mae(E)
计算公式:
mae:MAE=Σ|e|NMAE=Σ|e|N
mse:均方误差,Σe2NΣe2N
sse:误差平方和SSE=Σe2
放上一个动图
最后放上一个动图,演示神经网络学习的动态过程
%感知机动图
%%
%数据生成:与main3完全相同
clear;clc;close;
s = rng(5,'v5normal')%随机种子
%生成数据点
m1=20;m2=20;
p1=random('norm',0,1,2,m1);p2=random('norm',2,1,2,m2);p=[p1,p2];
t=[zeros(1,m1),ones(1,m2)];%分类标志
plotpv(p,t)
%%
net=newp([-1 1;-1 1],1)%???参数是p的范围,神经元数量
hold on
linehandle=plotpc(net.iw{1},net.b{1})
%%
net.adaptparam.passes=10;%???显示步数
for a=1:30%训练次数
[net,Y,E]=adapt(net,p,t);
linehandle=plotpc(net.iw{1},net.b{1},linehandle);%更新handle
pause(0.1)
end
您的支持将鼓励我继续创作!
Recommend
About Joyk
Aggregate valuable and interesting links.
Joyk means Joy of geeK