

【CNN】Object Localization
source link: https://www.guofei.site/2019/05/18/CNN.html
Go to the source link to view the article. You can view the picture content, updated content and better typesetting reading experience. If the link is broken, please click the button below to view the snapshot at that time.
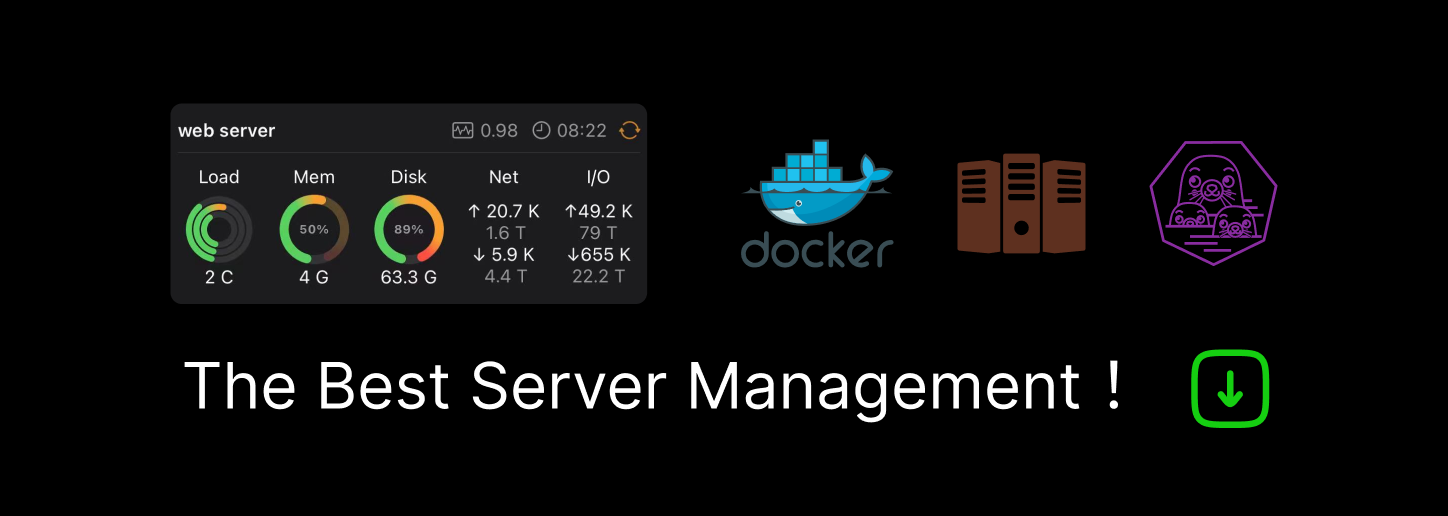
【CNN】Object Localization
2019年05月18日Author: Guofei
文章归类: 2-5-CV ,文章编号: 450
版权声明:本文作者是郭飞。转载随意,但需要标明原文链接,并通知本人
原文链接:https://www.guofei.site/2019/05/18/CNN.html
吴恩达的课程笔记
难度排列
- image classification
- classification with localization
- detection(multiple objects)
classification with localization
纯classification 最后一层是 softmax 层。但classification 除了softmax 层之外,还输出一个bounding box(bx,by,bh,bw),分别代表中心、宽高
yTyT=[pc,bx,by,bh,bw,c1,c2,c3,…,cn]
其中pc代表是否有目标物品,ci代表label
L(y^,y)={∑(y^i−yi)2(y^1−y1)2ify1=1ify1=0L(y^,y)={∑(y^i−yi)2ify1=1(y^1−y1)2ify1=0
当然你也可以分别用square error(坐标部分)+log-likelyhood error
Landmark Detection
- 目的:你想让算法识别并输出脸上的各个关键点的位置。做法:把坐标作为y来学习
sliding windows detection
用已经训练好的 CNN 算法扫描图片的每一部分。
缺点是计算量极大。
分析扫描过程发现,其实有很多计算是重复的,那么可以用下面的方法来节省算力:
- FC实际上可以看成一个卷积层。
- 用原本的卷积网络就可以了
注意,原模型的 stride 相当于扫描的 stride
缺点是不能找到精确的位置(精度取决于算法结构中的 stride)
Bounding Box Predictions
YOLO algorithm(you only look once)
Y做成这样:$19198,其中,其中19*19对应原图片上的每个grid,8对应的是对应原图片上的每个grid,8对应的是y^T$=[pc,bx,by,bh,bw,c1,c2,c3,…,cn]
注意:
- 如果某个物体跨越多个 grid,也只有一个grid 对应的 y 的pc是1.
- 每个grid 的左上是(0,0),右下是(1,1)
- bh,bw也是相对于边界长度的,可以大于1
- Intersection Over Union(IOU)算法画出的边界,和ground truth 画出的边界,两者的交集除以两者的并集。
- 完全正确,则IOU=1,
- 一般认为0.5以上是“正确”,你也可以用其它阈值。
- Non-max Suppression. 有可能一辆车覆盖很多 grid,然后很多grid 都各自预测出一辆车的位置。Pc是概率,选出一个概率最大的。
- step1 discard all boxes with pc≤0.6pc≤0.6
- step2 pick the largest pcpc
- step3 discard any remaining box with IoU≥0.5≥0.5with the box output in step2
- step4 back to step2
- Anchor Boxes. 如果某个grid 有多个物体,怎么办?y多倍
R-CNN
- region-CNN:先运行一个 segment algorithm,然后每个区域上做label+bounding box
- Fast R-CNN:先运行一个 segment algorithm,然后每个区域上做label+bounding box,但是用卷积的方法
- Faster R-CNN:用卷积
但是 Faster R-CNN还是比YOLO慢一些
您的支持将鼓励我继续创作!
Recommend
About Joyk
Aggregate valuable and interesting links.
Joyk means Joy of geeK