

【丢弃】条件概率,条件期望,条件方差
source link: https://www.guofei.site/2017/08/02/conditionalprobability.html
Go to the source link to view the article. You can view the picture content, updated content and better typesetting reading experience. If the link is broken, please click the button below to view the snapshot at that time.
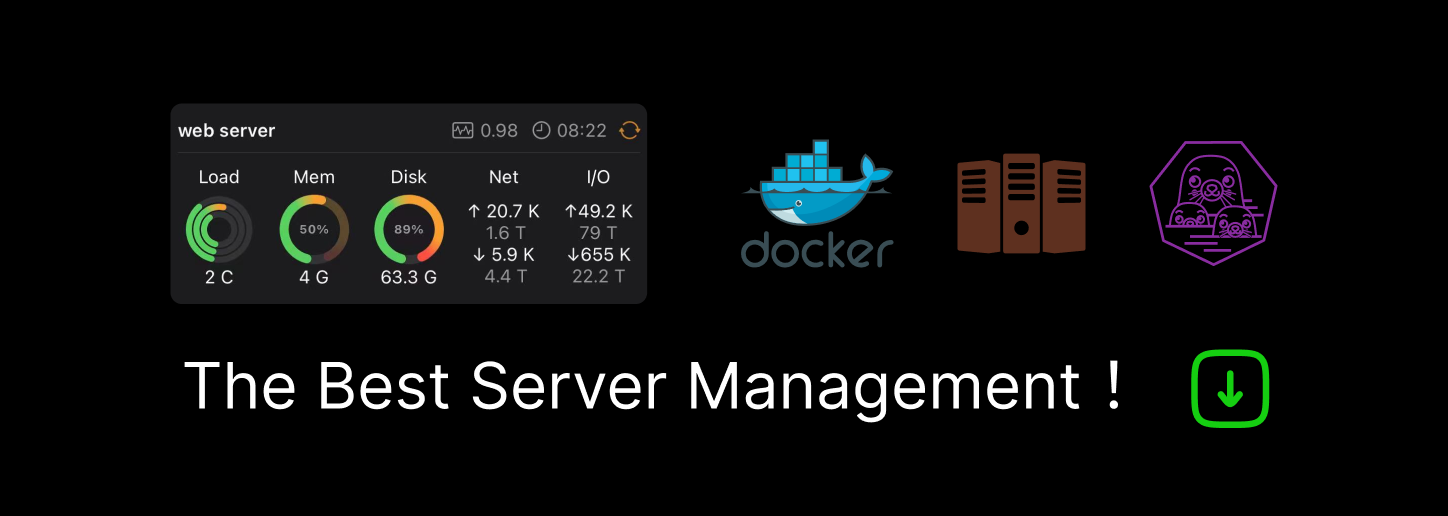
【丢弃】条件概率,条件期望,条件方差
2017年08月02日Author: Guofei
文章归类: ,文章编号: 450
版权声明:本文作者是郭飞。转载随意,但需要标明原文链接,并通知本人
原文链接:https://www.guofei.site/2017/08/02/conditionalprobability.html
这篇很多地方写的不够清楚,两年后做了更新,看这里
本文介绍概念:
条件概率
条件期望
条件方差
条件概率
给定一个样本空间
S,一个完全事件集合
ε={A∣A⊂S}ε={A∣A⊂S},一个概率测度
Pr:ε→[0,1]Pr:ε→[0,1],
给定事件B∈ε,Pr(B)>0B∈ε,Pr(B)>0,
那么,对于A∈εA∈ε,定义 条件概率 为Pr(A∣B)=P(A∩B)P(B)Pr(A∣B)=P(A∩B)P(B)
这种定义的好处是,Pr(⋆∣B)Pr(⋆∣B)符合概率测度的定义
条件PDF
p.d.f是f(x,y)f(x,y)
定义:f(x∣y)=f(x,y)f(y)f(x∣y)=f(x,y)f(y)
定理: f(y)=∑yf(x∣y)f(y)f(y)=∑yf(x∣y)f(y)
条件期望
联合期望 定义为:
E[g(x1,x2)]=∑(x1,x2)g(x1,x2)f(x1,x2)E[g(x1,x2)]=∑(x1,x2)g(x1,x2)f(x1,x2)
条件期望 定义为:
E[g(X1)∣X2=x2]=∑x1g(x1)f(x1∣x2)E[g(X1)∣X2=x2]=∑x1g(x1)f(x1∣x2)
记为E(g(X1)∣X2)E(g(X1)∣X2)
注意:
条件期望可以视为这样一个函数:s∈S,s→E(g(X1)∣X2(s))s∈S,s→E(g(X1)∣X2(s))
E(g(X1)∣X2)E(g(X1)∣X2)本身是一个随机变量。
定理:全期望定理(law of total expactation)
EX2[EX1∣X2[g(X1)∣X2]]=∑x1g(x1)f(x1)EX2[EX1∣X2[g(X1)∣X2]]=∑x1g(x1)f(x1)
也就是说,
E[X]=E[E[X∣Y]]E[X]=E[E[X∣Y]]
条件方差
方差 定义为:
E[(X−EX)2]E[(X−EX)2]
定理:Var(X)=EX2−(EX)2Var(X)=EX2−(EX)2
条件方差 定义为:
Var[X∣Y]=∑i(xi−E[X∣Y])2f(xi∣Y)Var[X∣Y]=∑i(xi−E[X∣Y])2f(xi∣Y)
定理:Var[X]=E[Var[X∣Y]]+Var[E[X∣Y]]Var[X]=E[Var[X∣Y]]+Var[E[X∣Y]]
总结
f(x∣y)=f(x,y)/fY(y)f(x∣y)=f(x,y)/fY(y)
VarX=EX2−(EX)2VarX=EX2−(EX)2
E(X∣Y)=∑xf(x∣y)E(X∣Y)=∑xf(x∣y)
Var(X∣Y)=∑x(x−ux∣y)2f(x∣y)Var(X∣Y)=∑x(x−ux∣y)2f(x∣y)
EX=E(E(x∣y))EX=E(E(x∣y))
VarX=E(Var(X∣Y))+Var(E(X∣Y))VarX=E(Var(X∣Y))+Var(E(X∣Y))
您的支持将鼓励我继续创作!
Recommend
-
42
首先回忆下均值和方差的定义,若存在$n$个数为$x_1, x_2, \dots, x_n$,则均值$\mu$为: $$\mu = \frac{x_1+x_2+\dots+x_n}{n}$$ 均值衡量的是数值集中在哪个数值附近。令标准差为$\sigma$,则方差$\sigma^2$为:
-
19
Sweet Snippet 之 方差计算
-
10
除夕年夜,奈雪的茶出其不意向香港联交所递交了IPO招股书,这意味着如果没有意外,“新式茶饮第一股”的桂冠将要落入奈雪的茶手中。 “奈雪的茶上市背后也有我做过的不少贡献。”在得知奈雪的茶将要赴港上市后,90后林霜在朋友圈发了一条如上...
-
7
数学篇 - 概率之联合概率、条件概率、边缘概率和贝叶斯法则(笔记)2020-09-13公式符号解释: P(A|B).是B已知下的A的条件概率联合概率(Joint Probability)各种解释...
-
6
摘要这一篇会过一遍条件概率公式, 贝叶斯公式, 边缘概率密度分布. 最后会给出一个例子, 把上面的知识点都用上. 这一篇会介绍关于条件概率公式, 贝叶斯公式和一个详细的例子计算. 之前其实我们有一篇类似的文章,
-
5
HMM引入马尔科夫假设,当前状态只与前一时刻状态有关,然而在很多情况下知道前一时刻状态与后一时刻状态对当前状态的判定有准确,例如分词。这种情况下,MEMM解决去掉了HMM的观察独立性假设,解决HMM的问题。但是,由于MEMM存在局部归一化问题,导致其倾向于选...
-
4
TorusSailing「Topcoder 12984」TorusSailing 有 的网格,一人从 随机游走,每次等概率向下或向右,问走到 的期望步数。 数据范围:。...
-
2
条件期望:Conditional Expectation 举例详解之入门之入门之草履虫都说听懂了 ...
-
2
【概率论】4-7:条件期望(Conditional Expectation) ...
-
3
条件数学期望 离散型随机变量 二维离散型随机变量 (...
About Joyk
Aggregate valuable and interesting links.
Joyk means Joy of geeK