

Play with Spark: Building Spark MLLib in a Play Spark Application
source link: https://blog.knoldus.com/play-with-spark-building-spark-mllib-in-a-play-spark-application/
Go to the source link to view the article. You can view the picture content, updated content and better typesetting reading experience. If the link is broken, please click the button below to view the snapshot at that time.
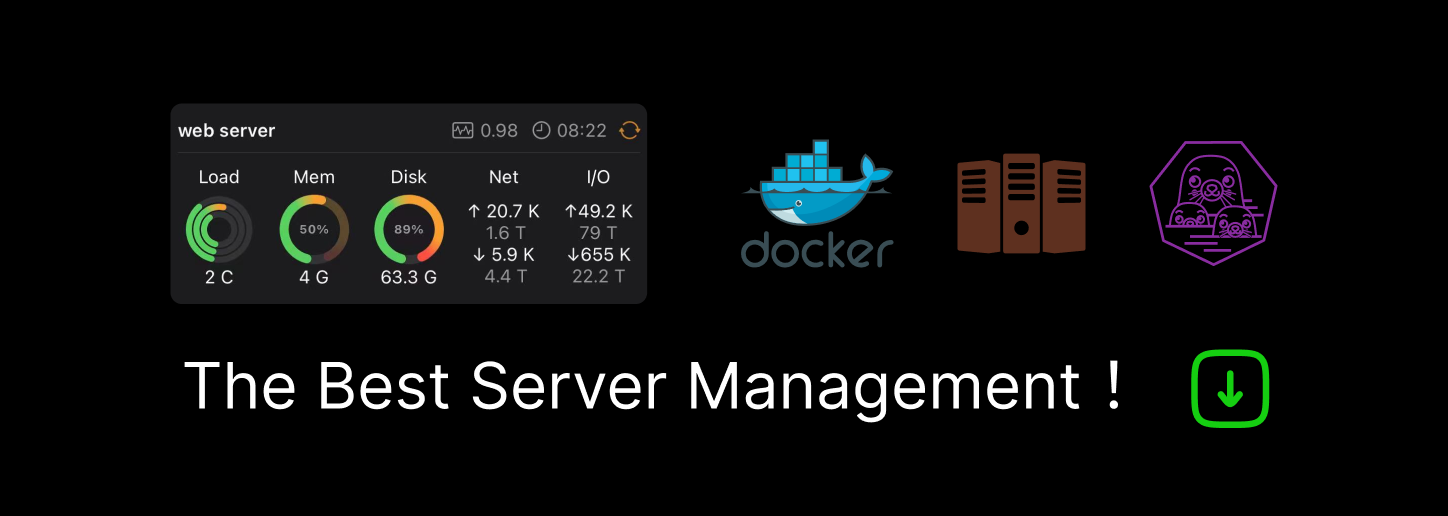
Play with Spark: Building Spark MLLib in a Play Spark Application
In our last post of Play with Spark! series, we saw how to integrate Spark SQL in a Play Scala application. Now in this blog we will see how to add Spark MLLib feature in a Play Scala application.
Spark MLLib is a new component under active development. It was first released with Spark 0.8.0. It contains some common machine learning algorithms and utilities, including classification, regression, clustering, collaborative filtering, dimensionality reduction, as well as some optimization primitives. For detailed list of available algorithms click here.
To add Spark MLLib feature in a Play Scala application follow these steps:
1). Add following dependencies in build.sbt file
xxxxxxxxxx
libraryDependencies ++= Seq(
"org.apache.spark" %% "spark-core" % "1.0.1",
"org.apache.spark" %% "spark-mllib" % "1.0.1"
)
The dependency – “org.apache.spark” %% “spark-mllib” % “1.0.1” is specific to Spark MLLib.
As you can see that we have upgraded to Spark 1.0.1 (latest release of Apache Spark).
2). Create a file app/utils/SparkMLLibUtility.scala & add following code to it
xxxxxxxxxx
package utils
import org.apache.spark.SparkContext
import org.apache.spark.SparkConf
import org.apache.spark.mllib.regression.LabeledPoint
import org.apache.spark.mllib.linalg.Vectors
import org.apache.spark.mllib.classification.NaiveBayes
object SparkMLLibUtility {
def SparkMLLibExample {
val conf = new SparkConf(false) // skip loading external settings
.setMaster("local[4]") // run locally with enough threads
.setAppName("firstSparkApp")
.set("spark.logConf", "true")
.set("spark.driver.host", "localhost")
val sc = new SparkContext(conf)
val data = sc.textFile("public/data/sample_naive_bayes_data.txt") // Sample dataset
val parsedData = data.map { line =>
val parts = line.split(',')
LabeledPoint(parts(0).toDouble, Vectors.dense(parts(1).split(' ').map(_.toDouble)))
}
// Split data into training (60%) and test (40%).
val splits = parsedData.randomSplit(Array(0.6, 0.4), seed = 11L)
val training = splits(0)
val test = splits(1)
val model = NaiveBayes.train(training, lambda = 1.0)
val prediction = model.predict(test.map(_.features))
val predictionAndLabel = prediction.zip(test.map(_.label))
val accuracy = 1.0 * predictionAndLabel.filter(x => x._1 == x._2).count() / test.count()
println("Accuracy = " + accuracy * 100 + "%")
}
}
In above code we have used Naive Bayes algorithm as an example.
3). In above code you can notice that we have parsed data into Vectors object of Spark.
xxxxxxxxxx
val parsedData = data.map { line =>
val parts = line.split(',')
LabeledPoint(parts(0).toDouble, Vectors.dense(parts(1).split(' ').map(_.toDouble)))
}
Reason for using Vectors object of Spark instead of Vector class of Scala is that, Vectors object of Spark contains both Dense & Sparse methods for parsing both dense & sparse type of data. This allows us to analyze data according to its properties.
4). Next we observe that we have split data in 2 parts – 60% for training & 40% for testing.
xxxxxxxxxx
// Split data into training (60%) and test (40%).
val splits = parsedData.randomSplit(Array(0.6, 0.4), seed = 11L)
val training = splits(0)
val test = splits(1)
5). Then we trained our model using Naive Bayes algorithm & training data.
xxxxxxxxxx
val model = NaiveBayes.train(training, lambda = 1.0)
6). At last we used our model to predict the labels/class of test data.
xxxxxxxxxx
val prediction = model.predict(test.map(_.features))
val predictionAndLabel = prediction.zip(test.map(_.label))
val accuracy = 1.0 * predictionAndLabel.filter(x => x._1 == x._2).count() / test.count()
println("Accuracy = " + accuracy * 100 + "%")
Then to find how good our model is, we calculated the Accuracy of the predicted labels.
So, we see that how easy it is to use any algorithm available in Spark MLLib to perform predictive analytics on data. For more information on Spark MLLib click here.
To download a Demo Application click here.
Written by Himanshu Gupta
Himanshu Gupta is a lead consultant having more than 4 years of experience. He is always keen to learn new technologies. He not only likes programming languages but Data Analytics too. He has sound knowledge of "Machine Learning" and "Pattern Recognition". He believes that the best result comes when everyone works as a team. He likes Coding, listening to music, watch movies, and read science fiction books in his free time.
Post navigation
6 thoughts on “Play with Spark: Building Spark MLLib in a Play Spark Application3 min read”
-
kunlqt says:
Reblogged this on Kunlqt's Blog.
Loading... -
Have you been able to get it working with play 2.3.2?
I’m getting a lot of strange errors. probably because of that akka version mismatch between spark and playLoading...-
Himanshu Gupta says:
Leon Radley (@LeonRadley) Play & Spark uses different version of Akka. That is why, we get a lot of strange errors. So, we have to use a common version of Akka.
Using Akka 2.2.3 can solve this problem.Loading...
-
-
Himanshu Gupta says:
Reblogged this on himanshu2014.
Loading... -
pushpendupurkait says:
Reblogged this on pushpendupurkait.
Loading... -
Arpit Suthar says:
Reblogged this on Arpit Suthar.
Loading...
Comments are closed.
Recommend
About Joyk
Aggregate valuable and interesting links.
Joyk means Joy of geeK