

Flink快速了解(7)——Async I/O
source link: https://niyanchun.com/flink-quick-learning-7-async-io.html
Go to the source link to view the article. You can view the picture content, updated content and better typesetting reading experience. If the link is broken, please click the button below to view the snapshot at that time.
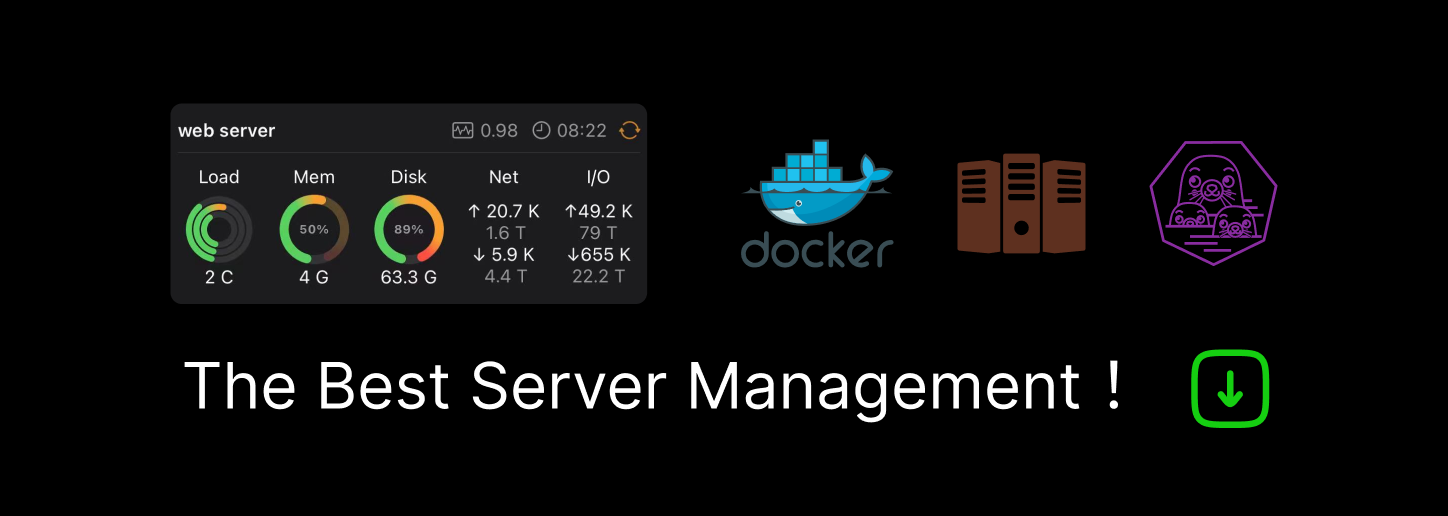
Flink快速了解(7)——Async I/O
上篇介绍了常见的算子,本文介绍另外一个重要的算子:Async I/O,即异步IO。它是流中频繁访问外部数据的利器,特别是当访问比较耗时的时候。
先考虑一个实际中挺常见的场景:一个流处理程序中对于每个事件都要查一次外部的维表(比如HBase,这里暂不考虑缓存机制)做关联,那在Flink中如何实现呢?典型的做法就是增加一个map/flatmap
,在里面做一下查询关联。这样功能没问题,但这个查询很容易会变成系统的瓶颈,特别是当外部查询比较耗时的时候。好在Flink里面有一个异步IO算子,可以很好的解决这个问题。
异步IO是阿里巴巴贡献给Flink非常重要的一个特性,在Flink 1.2版本中正式发布,对应的提案是FLIP-12: Asynchronous I/O Design and Implementation。这个特性解决的问题和所有其它的异步IO、IO多路复用技术是一致的:IO往往比较耗时,通过异步IO可以提高系统的吞吐量。这个Async I/O特性只不过是流处理场景里面的异步IO而已,原理没有什么特殊之处,看下官方的一个图:
左侧是同步IO,可以看到大部分时间用来等待了;右侧是异步IO,提升效果很明显。当然,通过提高任务的并行度也能一定程度的缓解同步IO的问题,这种方式有点类似于网络编程早期的per-connection-per-thread模型,但这种模式不够彻底,而且提高并行度的代价比较高。道理都懂,就不再赘述了,下面看怎么用。
回想一下网络编程中的异步IO(这里指的是IO多路复用技术),必须要内核支持select、poll/epoll才可以。Flink的异步IO也类似,需要访问外部数据的客户端支持异步请求才可以。如果不支持的话,也可以通过线程池技术模拟异步请求,当然效果上会差一些,但一般还是比同步IO强的。具体到编码层面,分3个步骤:
- 实现
AsyncFunction
接口,这个接口的作用是分发请求。Flink内置了一个实现类RichAsyncFunction
,一般我们继承这个类即可。 - 在
AsyncFunction#asyncInvoke(...)
中实现一个回调函数,在回调函数中获取异步执行的结果,并且传递给ResultFuture
。 - 将异步操作应用到某个流上面。
下面是官方给的一段示例代码:
// This example implements the asynchronous request and callback with Futures that have the
// interface of Java 8's futures (which is the same one followed by Flink's Future)
/**
* An implementation of the 'AsyncFunction' that sends requests and sets the callback.
* 第1步:实现`AsyncFunction`接口
*/
class AsyncDatabaseRequest extends RichAsyncFunction<String, Tuple2<String, String>> {
/** The database specific client that can issue concurrent requests with callbacks */
private transient DatabaseClient client;
@Override
public void open(Configuration parameters) throws Exception {
client = new DatabaseClient(host, post, credentials);
}
@Override
public void close() throws Exception {
client.close();
}
@Override
public void asyncInvoke(String key, final ResultFuture<Tuple2<String, String>> resultFuture) throws Exception {
// issue the asynchronous request, receive a future for result
final Future<String> result = client.query(key);
// set the callback to be executed once the request by the client is complete
// the callback simply forwards the result to the result future
// 第2步:实现一个回调函数
CompletableFuture.supplyAsync(new Supplier<String>() {
@Override
public String get() {
try {
// 获取异步执行的结果
return result.get();
} catch (InterruptedException | ExecutionException e) {
// Normally handled explicitly.
return null;
}
}
}).thenAccept( (String dbResult) -> {
// 并且传递给`ResultFuture`
resultFuture.complete(Collections.singleton(new Tuple2<>(key, dbResult)));
});
}
}
// create the original stream
DataStream<String> stream = ...;
// apply the async I/O transformation
// 第3步:将异步操作应用到某个流上面。
DataStream<Tuple2<String, String>> resultStream =
AsyncDataStream.unorderedWait(stream, new AsyncDatabaseRequest(), 1000, TimeUnit.MILLISECONDS, 100);
代码的执行逻辑是这样的:
- 流里面的每一个事件都会调用
asyncInvoke
,然后我们在这个方法里面实现访问外部数据的异步请求,比如上面代码中的client.query(key)
,然后得到一个异步对象(比如Future对象)。 - 给这个异步对象定义一个回调函数,比如上面代码中的
CompletableFuture.supplyAsync(...).thenAccept(...)
,然后在该回调函数获取异步任务的执行结果,并且交给ResultFuture
,这一步非常重要,待会还会再提到。
为了防止异步IO无限堆积或者某些请求挂死,一般都会提供超时设置和最高的异步并发数,Flink的异步IO也不例外。上面代码中最后一行的unorderedWait
参数中的1000就是异步任务的超时时间,100就是同时允许的并发请求数,称为Capacity。那超时后如何处理呢?看下AsyncFunction
接口:
public interface AsyncFunction<IN, OUT> extends Function, Serializable {
/**
* Trigger async operation for each stream input.
*
* @param input element coming from an upstream task
* @param resultFuture to be completed with the result data
* @exception Exception in case of a user code error. An exception will make the task fail and
* trigger fail-over process.
*/
void asyncInvoke(IN input, ResultFuture<OUT> resultFuture) throws Exception;
/**
* {@link AsyncFunction#asyncInvoke} timeout occurred. By default, the result future is
* exceptionally completed with a timeout exception.
*
* @param input element coming from an upstream task
* @param resultFuture to be completed with the result data
*/
default void timeout(IN input, ResultFuture<OUT> resultFuture) throws Exception {
resultFuture.completeExceptionally(
new TimeoutException("Async function call has timed out."));
}
}
除了定义了一个asyncInvoke
方法,还定义了一个timeout
,并且提供了一个默认实现。当异步任务超时后,就会回调该方法,默认是抛出异常,即整个任务失败。一般我们需要重新实现该方法。那如果并发数达到了限制会怎么样?答案是会阻塞,产生反压。
另外需要注意的一个地方就是事件的有序性。因为是异步请求,所以返回时间是不确定的,Flink的异步IO既支持保证事件的原始顺序,也可以不保证。异步IO的内部实现是依靠队列的,当需要保证有序的时候,实际是将先得到结果但排在后面的事件缓存起来,所以必然会增加一些延迟和资源占用。而无序可以获得更好的实时性和吞吐,但需要注意的是当使用Event Time的时候,这个无序并不是全局无序,而是在两个watermark之间的事件无序,整体还是要遵从watermark机制的。无序使用AsyncDataStream.unorderedWait(...)
,有序使用AsyncDataStream.orderedWait(...)
。
同步模式&线程池模式&异步模式对比demo
这部分主要是给了两个示例,演示如何使用Flink异步IO。
第一个例子中包含了3种场景:
- 在
flatmap
中实现外部访问的同步IO - 使用线程池实现的异步IO,且不保证顺序
- 使用线程池实现的异步IO,且保证顺序
其中,为了体现异步IO的优势,并没有真正访问数据库,而是使用了一个sleep操作,模拟比较耗时的IO操作。
public void update(Tuple2<String, Integer> tuple2) throws SQLException {
// 为了模拟耗时IO,这里使用sleep替换真正的数据库操作
//ps.setLong(1, balance);
//ps.setLong(2, 1);
//ps.execute();
try {
Thread.sleep(tuple2.f1);
} catch (InterruptedException e) {
e.printStackTrace();
}
}
注意:虽然没有真正访问数据库,但整个代码都是按照模拟真实场景写的,只是把里面执行数据库操作的换成了sleep,所以运行时还是会连接数据库、创建连接池。如果要自己运行代码,请修改代码中的数据库连接地址。另外,3个场景使用的数据源是同一个,而sleep的时间也是在数据源中定义好的,所以它们的IO耗时是相同的:
static List<Tuple2<String, Integer>> dataset() {
List<Tuple2<String, Integer>> dataset = new ArrayList<>(10);
for (int i = 0; i < 10; i++) {
// f0: 元素名称 f1: sleep的时间,模拟该元素需要的IO耗时
dataset.add(new Tuple2<>("e" + i, i % 3 + 1));
}
return dataset;
}
下面是完整代码:
public class ThreadPoolAsyncDemoFakeQuery {
public static void main(String[] args) throws Exception {
// --------------------------------- Async IO + Unordered -------------------------
StreamExecutionEnvironment envAsyncUnordered = StreamExecutionEnvironment.getExecutionEnvironment();
envAsyncUnordered.setParallelism(1);
envAsyncUnordered.setBufferTimeout(0);
DataStream<Tuple2<String, Integer>> source = envAsyncUnordered.fromCollection(dataset());
DataStream<String> result = AsyncDataStream.unorderedWait(source, new ThreadPoolAsyncMysqlRequest(),
10, TimeUnit.SECONDS, 10);
result.print();
System.out.println("Async + UnorderedWait:");
envAsyncUnordered.execute("unorderedWait");
// --------------------------------- Async IO + Ordered -------------------------
StreamExecutionEnvironment envAsyncOrdered = StreamExecutionEnvironment.getExecutionEnvironment();
envAsyncOrdered.setParallelism(1);
envAsyncOrdered.setBufferTimeout(0);
AsyncDataStream.orderedWait(envAsyncOrdered.fromCollection(dataset()),
new ThreadPoolAsyncMysqlRequest(), 10, TimeUnit.SECONDS, 10)
.print();
System.out.println("Async + OrderedWait");
envAsyncOrdered.execute("orderedWait");
// --------------------------------- Sync IO -------------------------
StreamExecutionEnvironment envSync = StreamExecutionEnvironment.getExecutionEnvironment();
envSync.setParallelism(1);
envSync.setBufferTimeout(0);
envSync.fromCollection(dataset())
.process(new ProcessFunction<Tuple2<String, Integer>, String>() {
private transient MysqlClient client;
@Override
public void open(Configuration parameters) throws Exception {
super.open(parameters);
client = new MysqlClient();
}
@Override
public void processElement(Tuple2<String, Integer> value, Context ctx, Collector<String> out) throws Exception {
try {
client.update(value);
out.collect(value + ": " + System.currentTimeMillis());
} catch (Exception e) {
e.printStackTrace();
}
}
}).print();
System.out.println("Sync IO:");
envSync.execute("Sync IO");
}
static List<Tuple2<String, Integer>> dataset() {
List<Tuple2<String, Integer>> dataset = new ArrayList<>(10);
for (int i = 0; i < 10; i++) {
// f0: 元素名称 f1: sleep的时间
dataset.add(new Tuple2<>("e" + i, i % 3 + 1));
}
return dataset;
}
static class ThreadPoolAsyncMysqlRequest extends RichAsyncFunction<Tuple2<String, Integer>, String> {
private transient ExecutorService executor;
private transient MysqlClient client;
@Override
public void open(Configuration parameters) throws Exception {
super.open(parameters);
executor = Executors.newFixedThreadPool(30);
client = new MysqlClient();
}
@Override
public void asyncInvoke(Tuple2<String, Integer> input, ResultFuture<String> resultFuture) {
executor.submit(() -> {
long current = System.currentTimeMillis();
String output = input + ":" + current;
try {
client.update(input);
resultFuture.complete(Collections.singleton(output));
} catch (SQLException e) {
e.printStackTrace();
resultFuture.complete(Collections.singleton(input + ": " + e.getMessage()));
}
});
}
@Override
public void timeout(Tuple2<String, Integer> input, ResultFuture<String> resultFuture) throws Exception {
System.out.printf("%s timeout\n", input);
resultFuture.complete(Collections.singleton(input + ": time out"));
}
@Override
public void close() throws Exception {
client.close();
super.close();
}
}
static class MysqlClient {
static final String JDBC_DRIVER = "com.mysql.jdbc.Driver";
static final String DB_URL = "jdbc:mysql://10.9.1.18:3306/test?useSSL=false";
private Connection conn;
private PreparedStatement ps;
public MysqlClient() throws Exception {
Class.forName(JDBC_DRIVER);
conn = DriverManager.getConnection(DB_URL, "root", "root123.");
ps = conn.prepareStatement("UPDATE account SET balance = ? WHERE id = ?;");
}
public void update(Tuple2<String, Integer> tuple2) throws SQLException {
// 为了模拟耗时IO,这里使用sleep替换真正的数据库操作
//ps.setLong(1, balance);
//ps.setLong(2, 1);
//ps.execute();
try {
Thread.sleep(tuple2.f1);
} catch (InterruptedException e) {
e.printStackTrace();
}
}
public void close() {
try {
if (conn != null) {
conn.close();
}
if (ps != null) {
ps.close();
}
} catch (Exception e) {
e.printStackTrace();
}
}
}
}
代码输出如下:
Async + UnorderedWait:
(e0,1):1617109474293
(e3,1):1617109474294
(e1,2):1617109474293
(e4,2):1617109474294
(e2,3):1617109474293
(e6,1):1617109474295
(e9,1):1617109474297
(e7,2):1617109474296
(e5,3):1617109474295
(e8,3):1617109474297
Async + OrderedWait
(e0,1):1617109474891
(e1,2):1617109474891
(e2,3):1617109474891
(e3,1):1617109474891
(e4,2):1617109474892
(e5,3):1617109474892
(e6,1):1617109474893
(e7,2):1617109474893
(e8,3):1617109474893
(e9,1):1617109474893
Sync IO:
(e0,1): 1617109475257
(e1,2): 1617109475260
(e2,3): 1617109475264
(e3,1): 1617109475265
(e4,2): 1617109475268
(e5,3): 1617109475272
(e6,1): 1617109475274
(e7,2): 1617109475277
(e8,3): 1617109475281
(e9,1): 1617109475283
可以看到:
- Async + UnorderedWait:耗时4ms,且输出的事件是乱序的
- Async + OrderedWait:耗时2ms,输出事件是保持原有顺序的
- Sync IO:耗时26ms,输出的事件也是保持原有顺序的
该程序运行多次,输出不太稳定,但Async方式都是远远小于Sync的。因为数据量比较小,而且耗时都比较平均,所以无序的优势不明显,有时甚至还会比有序高。这个例子并不是一个严格的性能测试,但却可以用来体现Async相比于Sync的明显优势。
第二个例子则是使用真实的异步请求客户端执行真正的操作。这里使用了jasync-sql用于异步访问MySQL。完整代码如下:
public class AsyncDemo {
public static void main(String[] args) throws Exception {
final long repeat = 100L;
// --------------------------------- Async IO + Unordered -------------------------
StreamExecutionEnvironment envAsyncUnordered = StreamExecutionEnvironment.getExecutionEnvironment();
envAsyncUnordered.setParallelism(1);
envAsyncUnordered.setBufferTimeout(0);
DataStream<Long> source = envAsyncUnordered.fromSequence(1, repeat);
DataStream<String> result = AsyncDataStream.unorderedWait(source, new AsyncMysqlRequest(),
10, TimeUnit.SECONDS, 10);
result.print();
System.out.println("Async + UnorderedWait:");
envAsyncUnordered.execute("unorderedWait");
}
static class AsyncMysqlRequest extends RichAsyncFunction<Long, String> {
Connection conn;
@Override
public void open(Configuration parameters) throws Exception {
super.open(parameters);
conn = MySQLConnectionBuilder.createConnectionPool("jdbc:mysql://{your-ip}:3306/test?user=root&password={your-password}");
conn.connect().get();
}
@Override
public void asyncInvoke(Long input, ResultFuture<String> resultFuture) throws Exception {
List<Long> balance = new ArrayList<>(1);
balance.add(input);
CompletableFuture<QueryResult> future =
conn.sendPreparedStatement("SELECT * FROM account WHERE balance = ?;", balance);
// 如果执行成功
future.thenAccept(queryResult -> {
resultFuture.complete(Collections.singleton(balance + ":" + System.currentTimeMillis()));
});
// 如果执行异常
future.exceptionally(e -> {
e.printStackTrace();
resultFuture.complete(Collections.singleton(balance + e.getMessage() + ":" + System.currentTimeMillis()));
return null;
});
}
@Override
public void timeout(Long input, ResultFuture<String> resultFuture) throws Exception {
System.out.printf("%d timeout\n", input);
resultFuture.complete(Collections.singleton(input + ": time out"));
}
}
}
使用注意点
- 不要在
AsyncFunction#asyncInvoke(...)
内部执行比较耗时的操作,比如同步等待异步请求的结果(应该放到回调中执行)。因为一个流中每个Partition只有一个AsyncFunction实例,一个实例里面的数据是顺序调用asyncInvoke
的,如果在里面执行耗时操作,那异步效果将大打折扣,如果同步等待异步的结果,那其实就退化成同步IO了。 - 异步请求超时回调默认是抛出异常,这样会导致整个Flink Job退出。这一般不是我们想要的,所以大多数时候都需要覆写timeout方法。
- 在自定义的回调函数里面一定要使用
ResultFuture#complete
或ResultFuture#completeExceptionally
将执行结果传递给ResultFuture,否则异步请求会一直堆积在队列里面。当队列满了以后,整个任务流就卡主了。 - Flink异步IO也是支持Checkpoint的,所以故障后可以恢复,提供Exactly-Once语义保证。
AsyncWaitOperator
这里结合目前最新的Flink 1.12.1版本从源码角度简单分析一下Async I/O的实现,这里引用了Jark博客中的几张图(见文末引用部分)。
如图,Flink Async I/O实质是通过队列的方式实现了一个异步的生产者-消费者模型。其中队列就是StreamElementQueue
;生产者就是就是我们的主流,通过AsyncDataStream.xxxWait
将数据放入队列;消费者就是图中的Emitter。调用流程就是流中的一个事件到了以后,先放入队列中,同时调用通过AsyncFunction#asyncInvoke(...)
定义的异步逻辑。当异步逻辑执行完成后,就会将队列中该事件标记为已完成,然后Emitter将该事件的结果发送到下游继续执行。实现Async I/O最主要的类是AsyncWaitOperator
,我把代码做了精简,保留了体现上面步骤的部分代码,具体见代码中的注释。
@Internal
public class AsyncWaitOperator<IN, OUT>
extends AbstractUdfStreamOperator<OUT, AsyncFunction<IN, OUT>>
implements OneInputStreamOperator<IN, OUT>, BoundedOneInput {
/** Capacity of the stream element queue. */
private final int capacity;
/** Output mode for this operator. */
private final AsyncDataStream.OutputMode outputMode;
/** Timeout for the async collectors. */
private final long timeout;
/** Queue, into which to store the currently in-flight stream elements. */
// 队列
private transient StreamElementQueue<OUT> queue;
@Override
public void setup(
StreamTask<?, ?> containingTask,
StreamConfig config,
Output<StreamRecord<OUT>> output) {
super.setup(containingTask, config, output);
switch (outputMode) {
case ORDERED:
queue = new OrderedStreamElementQueue<>(capacity);
break;
case UNORDERED:
queue = new UnorderedStreamElementQueue<>(capacity);
break;
default:
throw new IllegalStateException("Unknown async mode: " + outputMode + '.');
}
this.timestampedCollector = new TimestampedCollector<>(output);
}
@Override
public void processElement(StreamRecord<IN> element) throws Exception {
// add element first to the queue
// 将事件放入队列
final ResultFuture<OUT> entry = addToWorkQueue(element);
final ResultHandler resultHandler = new ResultHandler(element, entry);
// register a timeout for the entry if timeout is configured
// 设置异步回调的超时处理
if (timeout > 0L) {
final long timeoutTimestamp =
timeout + getProcessingTimeService().getCurrentProcessingTime();
final ScheduledFuture<?> timeoutTimer =
getProcessingTimeService()
.registerTimer(
timeoutTimestamp,
timestamp ->
userFunction.timeout(
element.getValue(), resultHandler));
resultHandler.setTimeoutTimer(timeoutTimer);
}
// 调用异步逻辑
userFunction.asyncInvoke(element.getValue(), resultHandler);
}
/**
* Add the given stream element to the operator's stream element queue. This operation blocks
* until the element has been added.
*
* <p>Between two insertion attempts, this method yields the execution to the mailbox, such that
* events as well as asynchronous results can be processed.
*
* @param streamElement to add to the operator's queue
* @throws InterruptedException if the current thread has been interrupted while yielding to
* mailbox
* @return a handle that allows to set the result of the async computation for the given
* element.
*/
private ResultFuture<OUT> addToWorkQueue(StreamElement streamElement)
throws InterruptedException {
Optional<ResultFuture<OUT>> queueEntry;
while (!(queueEntry = queue.tryPut(streamElement)).isPresent()) {
mailboxExecutor.yield();
}
return queueEntry.get();
}
/**
* Outputs one completed element. Watermarks are always completed if it's their turn to be
* processed.
*
* <p>This method will be called from {@link #processWatermark(Watermark)} and from a mail
* processing the result of an async function call.
*/
private void outputCompletedElement() {
if (queue.hasCompletedElements()) {
// emit only one element to not block the mailbox thread unnecessarily
queue.emitCompletedElement(timestampedCollector);
}
}
}
可以看到processElement
方法中先将事件放入队列,然后注册超时定时器,最后再调用异步处理逻辑。其中addToWorkQueue
中使用了一个while循环往队列里面放入事件,也就是如果队列满了,这个插入操作会一直阻塞在这里。也就是前文提到的:如果并发的异步请求数达到了Capacity的值,流就会阻塞产生反压。然后,在outputCompletedElement
方法中实现了将完成的异步请求事件发送到下游的操作。
前文提到了Flink Async I/O提供了有序和无序两种保证,这部分功能是通过不同的队列实现的。上面的代码中也能看到有序的时候使用的是OrderedStreamElementQueue
,无序的时候使用的是UnorderedStreamElementQueue
,队列的大小就是最大并发的异步请求数Capacity。这两个队列都是图中StreamElementQueue
接口的具体实现,先看下这个接口的定义:
/** Interface for stream element queues for the {@link AsyncWaitOperator}. */
@Internal
public interface StreamElementQueue<OUT> {
/**
* Tries to put the given element in the queue. This operation succeeds if the queue has
* capacity left and fails if the queue is full.
*
* <p>This method returns a handle to the inserted element that allows to set the result of the
* computation.
*
* @param streamElement the element to be inserted.
* @return A handle to the element if successful or {@link Optional#empty()} otherwise.
*/
Optional<ResultFuture<OUT>> tryPut(StreamElement streamElement);
/**
* Emits one completed element from the head of this queue into the given output.
*
* <p>Will not emit any element if no element has been completed (check {@link
* #hasCompletedElements()} before entering any critical section).
*
* @param output the output into which to emit
*/
void emitCompletedElement(TimestampedCollector<OUT> output);
/**
* Checks if there is at least one completed head element.
*
* @return True if there is a completed head element.
*/
boolean hasCompletedElements();
/**
* Returns the collection of {@link StreamElement} currently contained in this queue for
* checkpointing.
*
* <p>This includes all non-emitted, completed and non-completed elements.
*
* @return List of currently contained {@link StreamElement}.
*/
List<StreamElement> values();
/**
* True if the queue is empty; otherwise false.
*
* @return True if the queue is empty; otherwise false.
*/
boolean isEmpty();
/**
* Return the size of the queue.
*
* @return The number of elements contained in this queue.
*/
int size();
}
接口定义了5个方法:
tryPut
:向队列中插入数据;emitCompletedElement
:向下游发送已经完成的异步请求,即图上中的Emitter;hasCompletedElements
:判断是否有已经完成的异步请求values
:当前队列中的事件isEmpty
:队列是否为空size
:队列大小
下面分别看下有序和无序队列是如何实现这个接口的。
有序队列OrderedStreamElementQueue
有序实现比较简单,先进先出就行了。所以OrderedStreamElementQueue的代码量不多,我就全贴这里了:
@Internal
public final class OrderedStreamElementQueue<OUT> implements StreamElementQueue<OUT> {
private static final Logger LOG = LoggerFactory.getLogger(OrderedStreamElementQueue.class);
/** Capacity of this queue. */
private final int capacity;
/** Queue for the inserted StreamElementQueueEntries. */
private final Queue<StreamElementQueueEntry<OUT>> queue;
public OrderedStreamElementQueue(int capacity) {
Preconditions.checkArgument(capacity > 0, "The capacity must be larger than 0.");
this.capacity = capacity;
this.queue = new ArrayDeque<>(capacity);
}
@Override
public boolean hasCompletedElements() {
return !queue.isEmpty() && queue.peek().isDone();
}
@Override
public void emitCompletedElement(TimestampedCollector<OUT> output) {
if (hasCompletedElements()) {
final StreamElementQueueEntry<OUT> head = queue.poll();
head.emitResult(output);
}
}
@Override
public List<StreamElement> values() {
List<StreamElement> list = new ArrayList<>(this.queue.size());
for (StreamElementQueueEntry e : queue) {
list.add(e.getInputElement());
}
return list;
}
@Override
public boolean isEmpty() {
return queue.isEmpty();
}
@Override
public int size() {
return queue.size();
}
@Override
public Optional<ResultFuture<OUT>> tryPut(StreamElement streamElement) {
if (queue.size() < capacity) {
StreamElementQueueEntry<OUT> queueEntry = createEntry(streamElement);
queue.add(queueEntry);
LOG.debug(
"Put element into ordered stream element queue. New filling degree "
+ "({}/{}).",
queue.size(),
capacity);
return Optional.of(queueEntry);
} else {
LOG.debug(
"Failed to put element into ordered stream element queue because it "
+ "was full ({}/{}).",
queue.size(),
capacity);
return Optional.empty();
}
}
private StreamElementQueueEntry<OUT> createEntry(StreamElement streamElement) {
if (streamElement.isRecord()) {
return new StreamRecordQueueEntry<>((StreamRecord<?>) streamElement);
}
if (streamElement.isWatermark()) {
return new WatermarkQueueEntry<>((Watermark) streamElement);
}
throw new UnsupportedOperationException("Cannot enqueue " + streamElement);
}
}
里面就使用了一个ArrayDeque
实现了队列,事件按顺序进入队列,出队列调用的是queue.poll()
,所以顺序自然是保证的。在出队列的时候会先判断是否有已经完成的异步请求,即hasCompletedElements
,看下它的实现:
@Override
public boolean hasCompletedElements() {
return !queue.isEmpty() && queue.peek().isDone();
}
代码里面调用queue中头部元素(peek)的isDone
方法。队列里面的元素是StreamElementQueueEntry
接口类型,该接口继承自ResultFuture
接口,且对应的实现类是StreamRecordQueueEntry
,我们看下里面相关的实现代码:
class StreamRecordQueueEntry<OUT> implements StreamElementQueueEntry<OUT> {
@Nonnull private final StreamRecord<?> inputRecord;
private Collection<OUT> completedElements;
StreamRecordQueueEntry(StreamRecord<?> inputRecord) {
this.inputRecord = Preconditions.checkNotNull(inputRecord);
}
@Override
public boolean isDone() {
return completedElements != null;
}
@Nonnull
@Override
public StreamRecord<?> getInputElement() {
return inputRecord;
}
@Override
public void emitResult(TimestampedCollector<OUT> output) {
output.setTimestamp(inputRecord);
for (OUT r : completedElements) {
output.collect(r);
}
}
@Override
public void complete(Collection<OUT> result) {
this.completedElements = Preconditions.checkNotNull(result);
}
}
可以看到isDone
方法的逻辑是判断completedElements
是否为null,而completedElements
的赋值就是在complete
方法中的。这就是为什么我们必须在AsyncFunction#asyncInvoke(...)
中定义的回调函数中调用ResultFuture#complete
的原因。如果不这样,队列中的异步事件就无法被标记为已完成,当队列满了以后,整个系统的数据流就卡主了。当然异常时调用ResultFuture#completeExceptionally
也是OK的,这个在AsyncWaitOperator.ResultHandler
类中也有调用。
最后附一张来自Jark博客的图:
无序队列UnorderedStreamElementQueue
无序队列队列相对复杂一些。如果是使用Processing Time,那就是全局无序,也比较简单;但当使用Event Time的时候,如前文所述,就不是全局无序了,在相邻的两个watermark产生的区间内(代码实现中称为一个Segment)事件可以无序;但不同的区间还是要有序,即整体还是必须遵从Watermark的限制。所以为了实现这种机制,无序队列引入了Segment,一个Segment表示相邻watermark产生的一个区间。也就是Segment内无序,Segment之间有序。我们看下代码实现。
@Internal
public final class UnorderedStreamElementQueue<OUT> implements StreamElementQueue<OUT> {
private static final Logger LOG = LoggerFactory.getLogger(UnorderedStreamElementQueue.class);
/** Capacity of this queue. */
private final int capacity;
/** Queue of queue entries segmented by watermarks. */
private final Deque<Segment<OUT>> segments;
private int numberOfEntries;
public UnorderedStreamElementQueue(int capacity) {
Preconditions.checkArgument(capacity > 0, "The capacity must be larger than 0.");
this.capacity = capacity;
// most likely scenario are 4 segments <elements, watermark, elements, watermark>
this.segments = new ArrayDeque<>(4);
this.numberOfEntries = 0;
}
@Override
public Optional<ResultFuture<OUT>> tryPut(StreamElement streamElement) {
if (size() < capacity) {
StreamElementQueueEntry<OUT> queueEntry;
if (streamElement.isRecord()) {
queueEntry = addRecord((StreamRecord<?>) streamElement);
} else if (streamElement.isWatermark()) {
queueEntry = addWatermark((Watermark) streamElement);
} else {
throw new UnsupportedOperationException("Cannot enqueue " + streamElement);
}
numberOfEntries++;
LOG.debug(
"Put element into unordered stream element queue. New filling degree "
+ "({}/{}).",
size(),
capacity);
return Optional.of(queueEntry);
} else {
LOG.debug(
"Failed to put element into unordered stream element queue because it "
+ "was full ({}/{}).",
size(),
capacity);
return Optional.empty();
}
}
private StreamElementQueueEntry<OUT> addRecord(StreamRecord<?> record) {
// ensure that there is at least one segment
Segment<OUT> lastSegment;
if (segments.isEmpty()) {
lastSegment = addSegment(capacity);
} else {
lastSegment = segments.getLast();
}
// entry is bound to segment to notify it easily upon completion
StreamElementQueueEntry<OUT> queueEntry =
new SegmentedStreamRecordQueueEntry<>(record, lastSegment);
lastSegment.add(queueEntry);
return queueEntry;
}
private Segment<OUT> addSegment(int capacity) {
Segment newSegment = new Segment(capacity);
segments.addLast(newSegment);
return newSegment;
}
private StreamElementQueueEntry<OUT> addWatermark(Watermark watermark) {
Segment<OUT> watermarkSegment;
if (!segments.isEmpty() && segments.getLast().isEmpty()) {
// reuse already existing segment if possible (completely drained) or the new segment
// added at the end of
// this method for two succeeding watermarks
watermarkSegment = segments.getLast();
} else {
watermarkSegment = addSegment(1);
}
StreamElementQueueEntry<OUT> watermarkEntry = new WatermarkQueueEntry<>(watermark);
watermarkSegment.add(watermarkEntry);
// add a new segment for actual elements
addSegment(capacity);
return watermarkEntry;
}
@Override
public boolean hasCompletedElements() {
return !this.segments.isEmpty() && this.segments.getFirst().hasCompleted();
}
@Override
public void emitCompletedElement(TimestampedCollector<OUT> output) {
if (segments.isEmpty()) {
return;
}
final Segment currentSegment = segments.getFirst();
numberOfEntries -= currentSegment.emitCompleted(output);
// remove any segment if there are further segments, if not leave it as an optimization even
// if empty
if (segments.size() > 1 && currentSegment.isEmpty()) {
segments.pop();
}
}
@Override
public List<StreamElement> values() {
List<StreamElement> list = new ArrayList<>();
for (Segment s : segments) {
s.addPendingElements(list);
}
return list;
}
@Override
public boolean isEmpty() {
return numberOfEntries == 0;
}
@Override
public int size() {
return numberOfEntries;
}
/** An entry that notifies the respective segment upon completion. */
static class SegmentedStreamRecordQueueEntry<OUT> extends StreamRecordQueueEntry<OUT> {
private final Segment<OUT> segment;
SegmentedStreamRecordQueueEntry(StreamRecord<?> inputRecord, Segment<OUT> segment) {
super(inputRecord);
this.segment = segment;
}
@Override
public void complete(Collection<OUT> result) {
super.complete(result);
segment.completed(this);
}
}
/**
* A segment is a collection of queue entries that can be completed in arbitrary order.
*
* <p>All elements from one segment must be emitted before any element of the next segment is
* emitted.
*/
static class Segment<OUT> {
/** Unfinished input elements. */
private final Set<StreamElementQueueEntry<OUT>> incompleteElements;
/** Undrained finished elements. */
private final Queue<StreamElementQueueEntry<OUT>> completedElements;
Segment(int initialCapacity) {
incompleteElements = new HashSet<>(initialCapacity);
completedElements = new ArrayDeque<>(initialCapacity);
}
/** Signals that an entry finished computation. */
void completed(StreamElementQueueEntry<OUT> elementQueueEntry) {
// adding only to completed queue if not completed before
// there may be a real result coming after a timeout result, which is updated in the
// queue entry but
// the entry is not re-added to the complete queue
if (incompleteElements.remove(elementQueueEntry)) {
completedElements.add(elementQueueEntry);
}
}
/**
* True if there are no incomplete elements and all complete elements have been consumed.
*/
boolean isEmpty() {
return incompleteElements.isEmpty() && completedElements.isEmpty();
}
/**
* True if there is at least one completed elements, such that {@link
* #emitCompleted(TimestampedCollector)} will actually output an element.
*/
boolean hasCompleted() {
return !completedElements.isEmpty();
}
/**
* Adds the segmentd input elements for checkpointing including completed but not yet
* emitted elements.
*/
void addPendingElements(List<StreamElement> results) {
for (StreamElementQueueEntry<OUT> element : completedElements) {
results.add(element.getInputElement());
}
for (StreamElementQueueEntry<OUT> element : incompleteElements) {
results.add(element.getInputElement());
}
}
/**
* Pops one completed elements into the given output. Because an input element may produce
* an arbitrary number of output elements, there is no correlation between the size of the
* collection and the popped elements.
*
* @return the number of popped input elements.
*/
int emitCompleted(TimestampedCollector<OUT> output) {
final StreamElementQueueEntry<OUT> completedEntry = completedElements.poll();
if (completedEntry == null) {
return 0;
}
completedEntry.emitResult(output);
return 1;
}
/**
* Adds the given entry to this segment. If the element is completed (watermark), it is
* directly moved into the completed queue.
*/
void add(StreamElementQueueEntry<OUT> queueEntry) {
if (queueEntry.isDone()) {
completedElements.add(queueEntry);
} else {
incompleteElements.add(queueEntry);
}
}
}
}
队列部分的代码如下:
private final Deque<Segment<OUT>> segments;
// most likely scenario are 4 segments <elements, watermark, elements, watermark>
this.segments = new ArrayDeque<>(4);
/** Unfinished input elements. */
private final Set<StreamElementQueueEntry<OUT>> incompleteElements;
/** Undrained finished elements. */
private final Queue<StreamElementQueueEntry<OUT>> completedElements;
Segment(int initialCapacity) {
incompleteElements = new HashSet<>(initialCapacity);
completedElements = new ArrayDeque<>(initialCapacity);
}
可以看到整体还是一个ArrayDeque
,但队列内部是一个Segment;Segment内部又分成了2个队列:未完成请求队列incompleteElements
和已完成请求的队列completedElements
。Segment内部是无序的,所以incompleteElements使用的数据类型是Set。当某个请求完成后,就转移到completedElements中。此时,如果来的是普通事件,则调用addRecord
将事件放入对应Segment的incompleteElements队列中;如果来的是watermark,则调用addSegment
创建一个新的Segment。如果使用Processing Time,那就永远没有watermark,也就是全局就是一个大的Segment,也就是全局无序。
最后附一张Jark博客的图:
Flink Asycn I/O是阿里结合自身实践场景贡献给社区的一个特性,所以自然是有很多实际需求的。流中关联维表的操作在具体业务中也的确很常见,异步IO就是应对这些场景的利剑。在具体使用时要特别注意本文“使用注意点”一节的几个点。
References:
Recommend
About Joyk
Aggregate valuable and interesting links.
Joyk means Joy of geeK