

[PyTorch 学习笔记] 5.2 Hook 函数与 CAM 算法
source link: https://zhuanlan.zhihu.com/p/222496848
Go to the source link to view the article. You can view the picture content, updated content and better typesetting reading experience. If the link is broken, please click the button below to view the snapshot at that time.
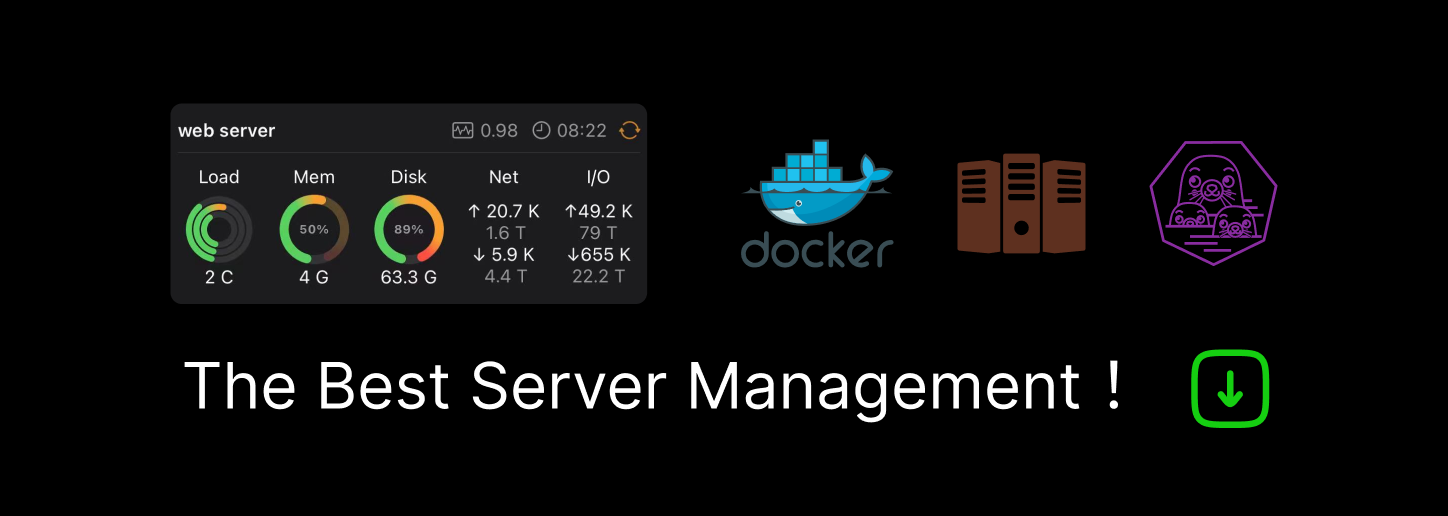
[PyTorch 学习笔记] 5.2 Hook 函数与 CAM 算法
本章代码:
这篇文章主要介绍了如何使用 Hook 函数提取网络中的特征图进行可视化,和 CAM(class activation map, 类激活图)
Hook 函数概念
Hook 函数是在不改变主体的情况下,实现额外功能。由于 PyTorch 是基于动态图实现的,因此在一次迭代运算结束后,一些中间变量如非叶子节点的梯度和特征图,会被释放掉。在这种情况下想要提取和记录这些中间变量,就需要使用 Hook 函数。
PyTorch 提供了 4 种 Hook 函数。
torch.Tensor.register_hook(hook)
功能:注册一个反向传播 hook 函数,仅输入一个参数,为张量的梯度。
hook
函数:
hook(grad)
- grad:张量的梯度
代码如下:
w = torch.tensor([1.], requires_grad=True)
x = torch.tensor([2.], requires_grad=True)
a = torch.add(w, x)
b = torch.add(w, 1)
y = torch.mul(a, b)
# 保存梯度的 list
a_grad = list()
# 定义 hook 函数,把梯度添加到 list 中
def grad_hook(grad):
a_grad.append(grad)
# 一个张量注册 hook 函数
handle = a.register_hook(grad_hook)
y.backward()
# 查看梯度
print("gradient:", w.grad, x.grad, a.grad, b.grad, y.grad)
# 查看在 hook 函数里 list 记录的梯度
print("a_grad[0]: ", a_grad[0])
handle.remove()
结果如下:
gradient: tensor([5.]) tensor([2.]) None None None
a_grad[0]: tensor([2.])
在反向传播结束后,非叶子节点张量的梯度被清空了。而通过hook
函数记录的梯度仍然可以查看。
hook
函数里面可以修改梯度的值,无需返回也可以作为新的梯度赋值给原来的梯度。代码如下:
w = torch.tensor([1.], requires_grad=True)
x = torch.tensor([2.], requires_grad=True)
a = torch.add(w, x)
b = torch.add(w, 1)
y = torch.mul(a, b)
a_grad = list()
def grad_hook(grad):
grad *= 2
return grad*3
handle = w.register_hook(grad_hook)
y.backward()
# 查看梯度
print("w.grad: ", w.grad)
handle.remove()
w.grad: tensor([30.])
torch.nn.Module.register_forward_hook(hook)
功能:注册 module 的前向传播hook
函数,可用于获取中间的 feature map。
hook
函数:
hook(module, input, output)
- module:当前网络层
- input:当前网络层输入数据
- output:当前网络层输出数据
下面代码执行的功能是 的卷积和
的池化。我们使用
register_forward_hook()
记录中间卷积层输入和输出的 feature map。
class Net(nn.Module):
def __init__(self):
super(Net, self).__init__()
self.conv1 = nn.Conv2d(1, 2, 3)
self.pool1 = nn.MaxPool2d(2, 2)
def forward(self, x):
x = self.conv1(x)
x = self.pool1(x)
return x
def forward_hook(module, data_input, data_output):
fmap_block.append(data_output)
input_block.append(data_input)
# 初始化网络
net = Net()
net.conv1.weight[0].detach().fill_(1)
net.conv1.weight[1].detach().fill_(2)
net.conv1.bias.data.detach().zero_()
# 注册hook
fmap_block = list()
input_block = list()
net.conv1.register_forward_hook(forward_hook)
# inference
fake_img = torch.ones((1, 1, 4, 4)) # batch size * channel * H * W
output = net(fake_img)
# 观察
print("output shape: {}\noutput value: {}\n".format(output.shape, output))
print("feature maps shape: {}\noutput value: {}\n".format(fmap_block[0].shape, fmap_block[0]))
print("input shape: {}\ninput value: {}".format(input_block[0][0].shape, input_block[0]))
输出如下:
output shape: torch.Size([1, 2, 1, 1])
output value: tensor([[[[ 9.]],
[[18.]]]], grad_fn=<MaxPool2DWithIndicesBackward>)
feature maps shape: torch.Size([1, 2, 2, 2])
output value: tensor([[[[ 9., 9.],
[ 9., 9.]],
[[18., 18.],
[18., 18.]]]], grad_fn=<ThnnConv2DBackward>)
input shape: torch.Size([1, 1, 4, 4])
input value: (tensor([[[[1., 1., 1., 1.],
[1., 1., 1., 1.],
[1., 1., 1., 1.],
[1., 1., 1., 1.]]]]),)
torch.Tensor.register_forward_pre_hook()
功能:注册 module 的前向传播前的hook
函数,可用于获取输入数据。
hook
函数:
hook(module, input)
- module:当前网络层
- input:当前网络层输入数据
torch.Tensor.register_backward_hook()
功能:注册 module 的反向传播的hook
函数,可用于获取梯度。
hook
函数:
hook(module, grad_input, grad_output)
- module:当前网络层
- input:当前网络层输入的梯度数据
- output:当前网络层输出的梯度数据
代码如下:
class Net(nn.Module):
def __init__(self):
super(Net, self).__init__()
self.conv1 = nn.Conv2d(1, 2, 3)
self.pool1 = nn.MaxPool2d(2, 2)
def forward(self, x):
x = self.conv1(x)
x = self.pool1(x)
return x
def forward_hook(module, data_input, data_output):
fmap_block.append(data_output)
input_block.append(data_input)
def forward_pre_hook(module, data_input):
print("forward_pre_hook input:{}".format(data_input))
def backward_hook(module, grad_input, grad_output):
print("backward hook input:{}".format(grad_input))
print("backward hook output:{}".format(grad_output))
# 初始化网络
net = Net()
net.conv1.weight[0].detach().fill_(1)
net.conv1.weight[1].detach().fill_(2)
net.conv1.bias.data.detach().zero_()
# 注册hook
fmap_block = list()
input_block = list()
net.conv1.register_forward_hook(forward_hook)
net.conv1.register_forward_pre_hook(forward_pre_hook)
net.conv1.register_backward_hook(backward_hook)
# inference
fake_img = torch.ones((1, 1, 4, 4)) # batch size * channel * H * W
output = net(fake_img)
loss_fnc = nn.L1Loss()
target = torch.randn_like(output)
loss = loss_fnc(target, output)
loss.backward()
输出如下:
forward_pre_hook input:(tensor([[[[1., 1., 1., 1.],
[1., 1., 1., 1.],
[1., 1., 1., 1.],
[1., 1., 1., 1.]]]]),)
backward hook input:(None, tensor([[[[0.5000, 0.5000, 0.5000],
[0.5000, 0.5000, 0.5000],
[0.5000, 0.5000, 0.5000]]],
[[[0.5000, 0.5000, 0.5000],
[0.5000, 0.5000, 0.5000],
[0.5000, 0.5000, 0.5000]]]]), tensor([0.5000, 0.5000]))
backward hook output:(tensor([[[[0.5000, 0.0000],
[0.0000, 0.0000]],
[[0.5000, 0.0000],
[0.0000, 0.0000]]]]),)
hook
函数实现机制
hook
函数实现的原理是在module
的__call()__
函数进行拦截,__call()__
函数可以分为 4 个部分:
- 第 1 部分是实现 _forward_pre_hooks
- 第 2 部分是实现 forward 前向传播
- 第 3 部分是实现 _forward_hooks
- 第 4 部分是实现 _backward_hooks
由于卷积层也是一个module
,因此可以记录_forward_hooks
。
def __call__(self, *input, **kwargs):
# 第 1 部分是实现 _forward_pre_hooks
for hook in self._forward_pre_hooks.values():
result = hook(self, input)
if result is not None:
if not isinstance(result, tuple):
result = (result,)
input = result
# 第 2 部分是实现 forward 前向传播
if torch._C._get_tracing_state():
result = self._slow_forward(*input, **kwargs)
else:
result = self.forward(*input, **kwargs)
# 第 3 部分是实现 _forward_hooks
for hook in self._forward_hooks.values():
hook_result = hook(self, input, result)
if hook_result is not None:
result = hook_result
# 第 4 部分是实现 _backward_hooks
if len(self._backward_hooks) > 0:
var = result
while not isinstance(var, torch.Tensor):
if isinstance(var, dict):
var = next((v for v in var.values() if isinstance(v, torch.Tensor)))
else:
var = var[0]
grad_fn = var.grad_fn
if grad_fn is not None:
for hook in self._backward_hooks.values():
wrapper = functools.partial(hook, self)
functools.update_wrapper(wrapper, hook)
grad_fn.register_hook(wrapper)
return result
Hook 函数提取网络的特征图
下面通过hook
函数获取 AlexNet 每个卷积层的所有卷积核参数,以形状作为 key,value 对应该层多个卷积核的 list。然后取出每层的第一个卷积核,形状是 [1, in_channle, h, w],转换为 [in_channle, 1, h, w],使用 TensorBoard 进行可视化,代码如下:
writer = SummaryWriter(comment='test_your_comment', filename_suffix="_test_your_filename_suffix")
# 数据
path_img = "imgs/lena.png" # your path to image
normMean = [0.49139968, 0.48215827, 0.44653124]
normStd = [0.24703233, 0.24348505, 0.26158768]
norm_transform = transforms.Normalize(normMean, normStd)
img_transforms = transforms.Compose([
transforms.Resize((224, 224)),
transforms.ToTensor(),
norm_transform
])
img_pil = Image.open(path_img).convert('RGB')
if img_transforms is not None:
img_tensor = img_transforms(img_pil)
img_tensor.unsqueeze_(0) # chw --> bchw
# 模型
alexnet = models.alexnet(pretrained=True)
# 注册hook
fmap_dict = dict()
for name, sub_module in alexnet.named_modules():
if isinstance(sub_module, nn.Conv2d):
key_name = str(sub_module.weight.shape)
fmap_dict.setdefault(key_name, list())
# 由于AlexNet 使用 nn.Sequantial 包装,所以 name 的形式是:features.0 features.1
n1, n2 = name.split(".")
def hook_func(m, i, o):
key_name = str(m.weight.shape)
fmap_dict[key_name].append(o)
alexnet._modules[n1]._modules[n2].register_forward_hook(hook_func)
# forward
output = alexnet(img_tensor)
# add image
for layer_name, fmap_list in fmap_dict.items():
fmap = fmap_list[0]# 取出第一个卷积核的参数
fmap.transpose_(0, 1) # 把 BCHW 转换为 CBHW
nrow = int(np.sqrt(fmap.shape[0]))
fmap_grid = vutils.make_grid(fmap, normalize=True, scale_each=True, nrow=nrow)
writer.add_image('feature map in {}'.format(layer_name), fmap_grid, global_step=322)
使用 TensorBoard 进行可视化如下:
CAM(class activation map, 类激活图)
暂未完成。列出两个参考文章。
参考资料
如果你觉得这篇文章对你有帮助,不妨点个赞,让我有更多动力写出好文章。
Recommend
About Joyk
Aggregate valuable and interesting links.
Joyk means Joy of geeK