

Pandas Tricks that Expedite Data Analysis Process
source link: https://mc.ai/pandas-tricks-that-expedite-data-analysis-process/
Go to the source link to view the article. You can view the picture content, updated content and better typesetting reading experience. If the link is broken, please click the button below to view the snapshot at that time.
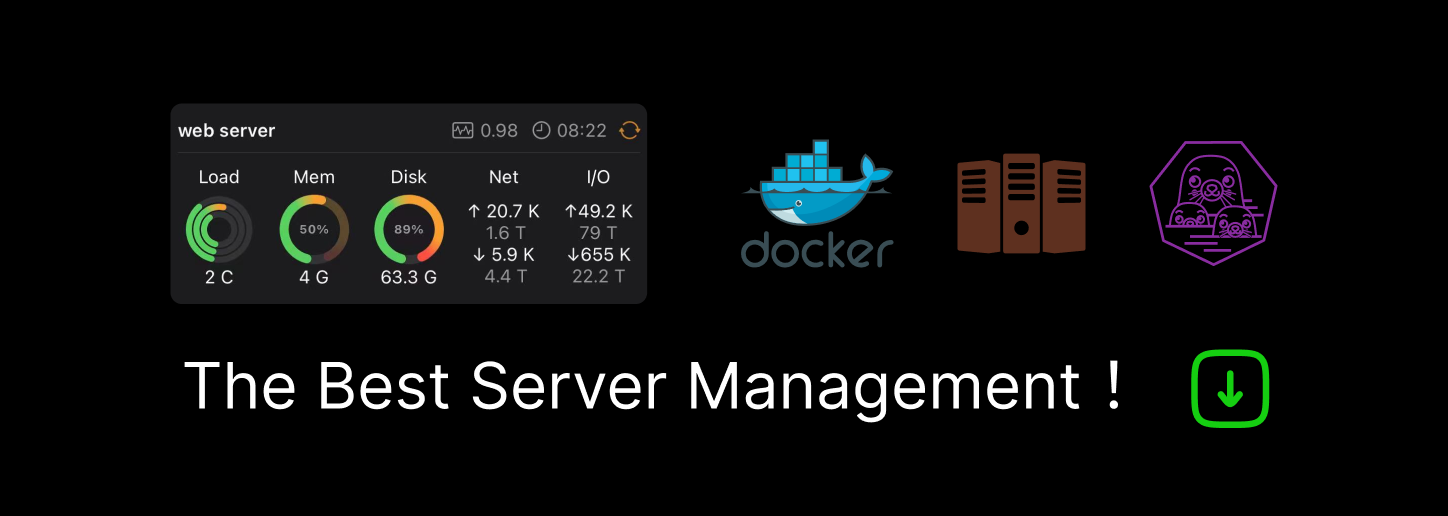
Pandas Tricks that Expedite Data Analysis Process
Speed-up your data analysis process with these simple tricks.
Pandas is a very powerful and versatile Python data analysis library that expedites the preprocessing steps of data science projects. It provides numerous functions and methods that are quite useful in data analysis.
As always we start with importing numpy and pandas.
import numpy as np import pandas as pd
Let’s create a sample dataframe to work on. Pandas is a versatile library that usually offers multiple ways to do a task. Thus, there are many ways to create a dataframe. One common method is to pass a dictionary that includes columns as key-value pairs.
values = np.random.randint(10, size=10)years = np.arange(2010,2020)groups = ['A','A','B','A','B','B','C','A','C','C']df = pd.DataFrame({'group':groups, 'year':years, 'value':values})df
We also used numpy to create arrays to be used as values in columns. np.arange returns a range values within specified interval. np.random.randint returns random integer values based on the specified range and size.
The dataframe contains some yearly values of 3 different groups. We may only be interested in yearly values but there are some cases in which we also need a cumulative sum. Pandas provides an easy-to-use function to calculate cumulative sum which is cumsum .
df['cumsum'] = df['value'].cumsum()df
We created a column named “cumsum” which contains cumulative sum of the numbers in value column. However, it does not take the groups into consideration. This kind of cumulative values may be useless in some cases because we are not able to distinguish between groups. Don’t worry! There is a very simple and convenient solution for this issue. We can apply groupby function.
df['cumsum'] = df[['value','group']].groupby('group').cumsum()df
We first applied groupby on “group” column then cumsum function. Now the values are summed up within each group. To make the dataframe look nicer, we may want to sort the values based on group instead of year so that we can visually separate groups.
df.sort_values(by='group').reset_index()
We applied sort_values function and reset the index with reset_index function. As we can see in the returned dataframe, original index is kept as a column. We can eliminate it by setting drop parameter of reset_index function as True.
df = df.sort_values(by='group').reset_index(drop=True)df
It looks better now. When we want to add a new column to a dataframe, it is added at the end by default. However, pandas offers the option to add the new column in any position using insert function.
new = np.random.randint(5, size=10)df.insert(2, 'new_col', new)df
We specified the position by passing an index as first argument. This value must be an integer. Column indices start from zero just like row indices. The second argument is column name and the third argument is the object that includes values which can be Series or an array-like object.
Consider we want to remove a column from a dataframe but also want store keep that column as a separate series. One way is to assign the column to a series and then use drop function. A simpler way is to use pop functionn.
value = df.pop('value')df
With one line of code, we remove the value column from the dataframe and store it in a pandas series.
We sometimes need to filter a dataframe based on a condition or apply a mask to get certain values. One easy way to filter a dataframe is query function. I will use the sample dataframe we have been using. Let’s first insert the “value” column back:
df.insert(2, 'value', value)df
It is very simple to use query function which only requires the condition.
df.query('value < new_col')
It returned the rows in which “value” is less then “new_col”. We can set more complex conditions and also use additional operators.
df.query('2*new_col > value')
We can also combine multiple conditions into one query.
df.query('2*new_col > value & cumsum < 15')
There are many aggregations functions that we can use to calculate basic statistics on columns such as mean, sum, count and so on. We can apply each of these function to a column. However, in some cases, we may need to check more than one type statistics. For instance, both count and mean might be important in some cases. Instead of applying functions separately, pandas offers agg function to apply multiple aggregation functions.
df[['group','value']].groupby('group').agg(['mean','count'])
It makes more sense to see both mean and count. We can easily detect outliers that have extreme mean values but very low number of observations.
Recommend
About Joyk
Aggregate valuable and interesting links.
Joyk means Joy of geeK