

Deleting data distributed throughout a microservice architecture
source link: https://blog.twitter.com/engineering/en_us/topics/infrastructure/2020/deleting-data-distributed-throughout-your-microservices-architecture.html
Go to the source link to view the article. You can view the picture content, updated content and better typesetting reading experience. If the link is broken, please click the button below to view the snapshot at that time.
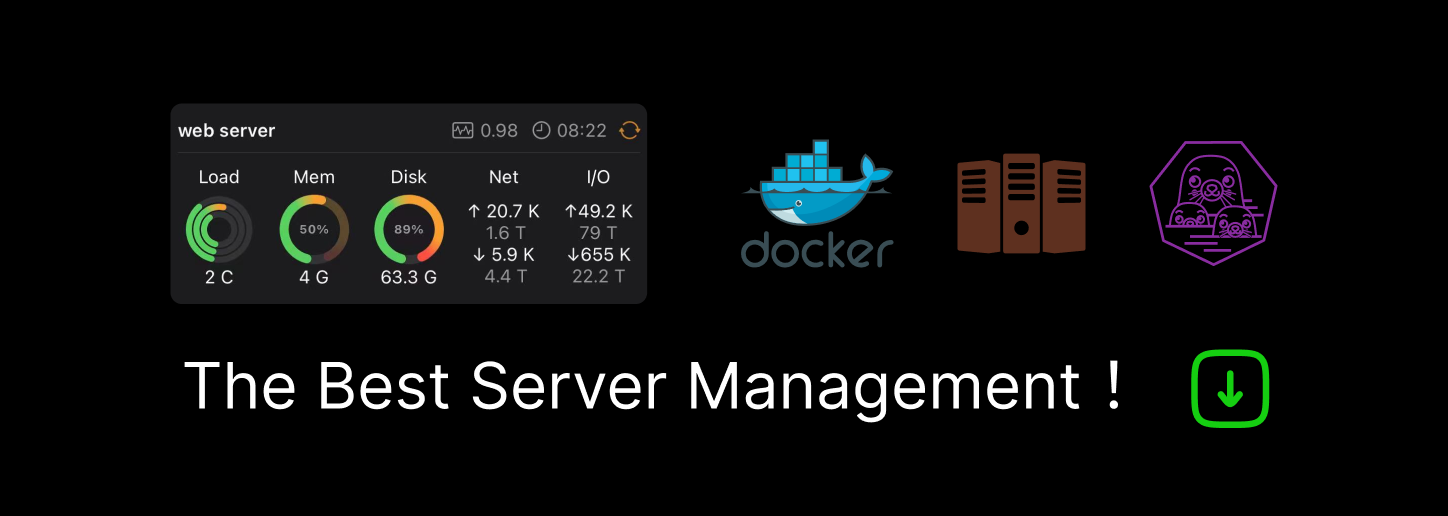
Microservices architectures tend to distribute responsibility for data throughout an organization. This poses challenges to ensuring that data is deleted. A common solution is to set an organization-wide standard of per-dataset or per-record retentions. There will always be data, however, that spans multiple datasets and records. This data is often distributed throughout your microservices architecture, requiring coordination between systems and teams to delete it.
One solution is to think of data deletion not as an event, but as a process. At Twitter, we call this process “erasure” and coordinate data deletion between systems using an erasure pipeline. In this post, we’ll discuss how to set up an erasure pipeline, including data discoverability, access, and processing. We’ll also touch on common problems and how to ensure ongoing maintenance of an erasure pipeline.
Discoverability
First, you’ll need to find the data that needs to be deleted. Data about a given event, user, or record could be in online or offline datasets, and may be owned by disparate parts of your organization. So your first job will be to use your knowledge of your organization, the expertise of your peers, and organization-wide communication channels to compile a list of all relevant data.
Data Access and Processing Methods
The data you find will usually be accessible to you in one of three ways. Online data will be mutable via (1) a real-time API or (2) an asynchronous mutator. Offline warehoused data will be mutable via (3) a parallel-distributed processing framework like MapReduce . In order to reach every piece of data, your pipeline will need to support each of these three processing methods.
Data mutable via a real-time API is the simplest. Your erasure pipeline can call that API to perform data deletion tasks. Once the API calls have succeeded for each piece of data, the data has been deleted and your erasure pipeline is finished.
The downside of this approach is that it assumes every data deletion task can be completed within the span of an API call, usually seconds or milliseconds, when it may take longer. In this case, your erasure pipeline has to get a bit more complicated. Examples of data that can’t be deleted in the span of an API call include data that is exported to offline snapshots, or data that exists in multiple backend systems and caches. This data denormalization is inherent to your microservices architecture and increases performance. It also means that responsibility for the data’s lifecycle is delegated to the team who owns the data’s APIs and business logic.
You’ll need to inform data owners that data deletion needs to happen. Your erasure pipeline can publish erasure events to a distributed queue, like Kafka , which partner teams subscribe to in order to initiate data deletion. They process the erasure event and call back to your team to confirm that the data was deleted.
Finally, there may be completely offline datasets containing data that needs to be deleted, such as snapshots or model training data. In these cases, you can provide an offline dataset which partner teams use to remove erasable data from their datasets. This offline dataset can be as simple as persisted logs from your erasure event publisher.
An Erasure Pipeline
The erasure pipeline we’ve described thus far has a few key requirements. It must:
- Accept incoming erasure requests
- Track and persist which pieces of data have been deleted
- Call synchronous APIs to delete data
- Publish erasure events for asynchronous erasure
- Generate an offline dataset of erasure events
An example erasure pipeline might look like this:
This Tweet is unavailable
This Tweet is unavailable.
Recommend
About Joyk
Aggregate valuable and interesting links.
Joyk means Joy of geeK