

Redis和GCP AI服务搭建RAG参考架构解决方案
source link: https://weedge.github.io/post/doraemon/redis_gcp_rag_stack/
Go to the source link to view the article. You can view the picture content, updated content and better typesetting reading experience. If the link is broken, please click the button below to view the snapshot at that time.
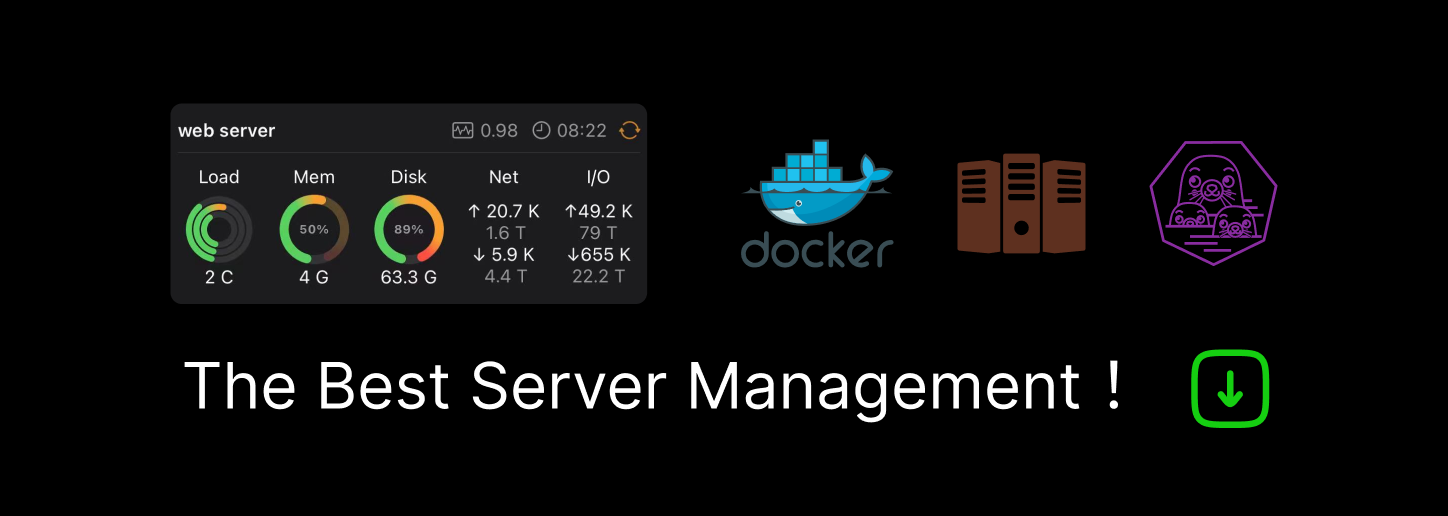
Redis和GCP AI服务搭建RAG参考架构解决方案
本文主要是讲解一个快速搭建比如RAG pipeline相关应用参考方案,结合云厂商GCP AI服务,以及redis stack | vector index,借助 Google Cloud Platform 上易用的开发SDK, 以及使用redislabs 提供的免费30M内存空间服务;GCP新用户前三个月好像是免费使用一些服务,而且提供 $300 的赠金使用,对于前期学习和使用体验服务还是不错的选择,而且个人感觉学习文档很齐全,不会很零散。但是解决方案相对AWS要少些,毕竟AWS做的很深入,搭建解决方案很方便,集成开发工具比较齐全,特别是serverless lambda服务,可以看下以前写的文章『 用户行为分析方案设计』通过CDK构建解决方案stack(用于前期架构推演,不要YY,要动手,节约成本是干出来的)。
以前注册的,忘记用了。。。
笔记地址:https://github.com/weedge/doraemon-nb/blob/main/Google_BigQuery_Palm_Redis.ipynb
注:这里使用redis作为向量索引数据库,也可以结合其他向量索引库来搭建相应方案。主要目的是熟悉GCP服务和redis cloud服务。
☁️ Google 的 Vertex AI 通过引入生成式 AI扩展了其能力。这项先进技术配备了专门的控制台工作室体验、专用 API 和 Python SDK,专为部署和管理 Google 强大的 PaLM 语言模型实例而设计(更多示例代码)。PaLM 模型专注于文本生成、摘要、聊天完成和嵌入创建,正在重塑自然语言处理和机器学习的边界。
⚡ redis stack 提供向量数据库功能,具有高效的 API 用于创建向量索引、管理、选择距离度量、相似性搜索和混合过滤。当与它的多功能数据结构(包括列表、哈希、JSON 和集合)结合使用时,redis stack 作为打造高质量基于大型语言模型(LLM)的应用程序的最佳解决方案而脱颖而出。它体现了简化的架构和性能,使其成为生产环境中不可或缺的工具。
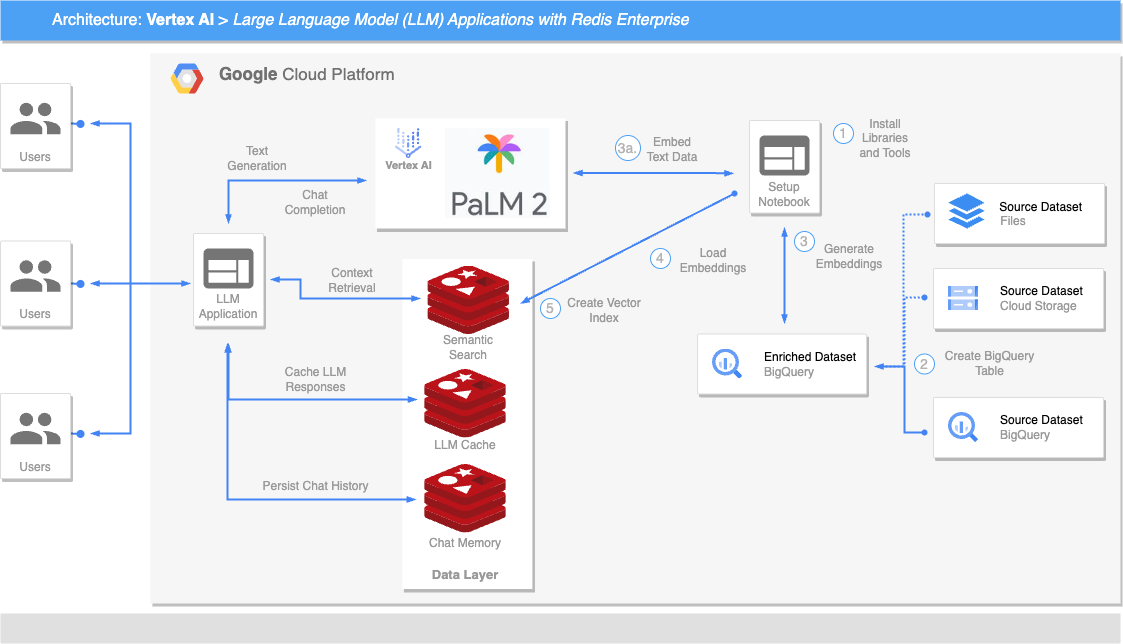
接下来,我们将通过几种与 Vertex AI LLM 和 Redis Enterprise 相关的设计模式,确保最佳的生产性能。
1. 先决条件
在我们开始之前,我们必须安装一些必需的库,与 Google 进行身份验证,创建 Redis 数据库,并初始化其他必需的组件。
安装必需的库
!pip install redis "google-cloud-aiplatform==1.25.0" --upgrade --user
本地安装 Redis(可选)
如果您在其他地方已经运行了安装了 Redis Stack 的 Redis 数据库,那么您不需要在这台机器上运行它。可以跳过这一步,直接进行“连接到 Redis 服务器”的步骤。
curl -fsSL https://packages.redis.io/gpg | sudo gpg --dearmor -o /usr/share/keyrings/redis-archive-keyring.gpg
echo "deb [signed-by=/usr/share/keyrings/redis-archive-keyring.gpg] https://packages.redis.io/deb $(lsb_release -cs) main" | sudo tee /etc/apt/sources.list.d/redis.list
sudo apt-get update > /dev/null 2>&1
sudo apt-get install redis-stack-server > /dev/null 2>&1
redis-stack-server --daemonize yes
在云上使用免费的 Redis Cloud 账户
您也可以激活 Redis Cloud 的永久免费实例。激活步骤如下:
- 访问 https://redis.com/try-free/
- 注册(使用基于 Gmail 的注册是最简便的方式)
- 创建新订阅
- 使用以下选项:
- 固定计划,选择部署服务到 Google Cloud或者 aws都可以
- 新的 30Mb 免费数据库
- 创建新的 RedisStack 数据库
如果您是第一次在 Redis Cloud 注册 - 那么最后几步默认会自动为您执行(默认使用aws)。请记录下新数据库的主机、端口和默认密码。您可以在接下来的代码块中使用这些信息,而不是默认的 localhost
。
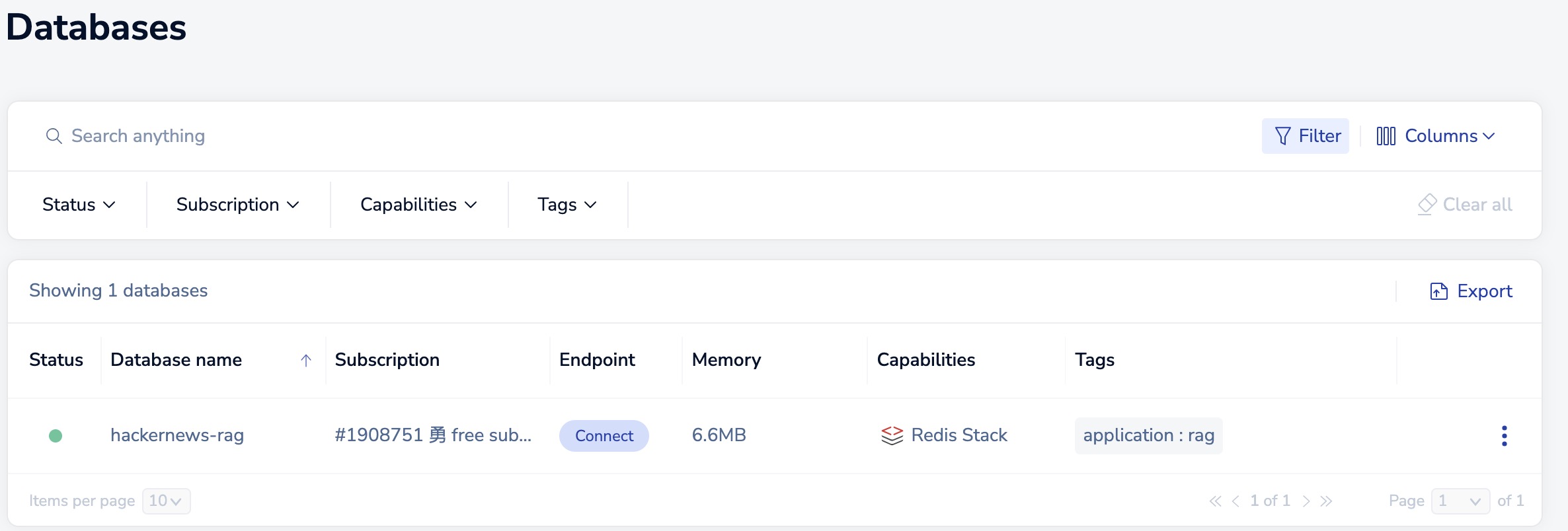
连接到 Redis 服务器
如果您正在连接到外部的 Redis 实例,请将下面的连接参数替换为您自己的。
import os
import redis
# Redis connection params
REDIS_HOST = os.getenv("REDIS_HOST", "redis-10610.c274.us-east-1-3.ec2.cloud.redislabs.com") #"redis-10610.c274.us-east-1-3.ec2.cloud.redislabs.com"
REDIS_PORT = os.getenv("REDIS_PORT", "10610") #10610
REDIS_PASSWORD = os.getenv("REDIS_PASSWORD", "******") #"pobhBJP7Psicp2gV0iqa2ZOc1WdXXXXX"
# Create Redis client
redis_client = redis.Redis(
host=REDIS_HOST,
port=REDIS_PORT,
password=REDIS_PASSWORD
)
# Test connection
redis_client.ping()
# Clear Redis database (optional)
redis_client.flushdb()
GCP认证
如果使用google的colab操作,直接认证用户,然后会提示关联到对应google账户。
from google.colab import auth
auth.authenticate_user()
print('Authenticated')
from getpass import getpass
设置所在项目id 和 区域服务
# input your GCP project ID and region for Vertex AI
PROJECT_ID = getpass("PROJECT_ID:") #'central-beach-194106'
REGION = input("REGION:") #'us-central1'
如果是本地,可以使用gcloud
cli来操作 (类似 aws
cli工具)
初始 Vertex AI 组件
import vertexai
vertexai.init(project=PROJECT_ID, location=REGION)
2. 创建 BigQuery 表
第二步涉及到为我们的 LLM 应用程序准备数据集。我们使用了来自 Google BigQuery 的一个免费(公开)的黑客新闻数据集。
利用 BigQuery 构建机器学习应用程序是一种常见模式,因为它具有强大的查询和分析能力。
我们将从为这个数据集创建我们自己的 BigQuery 表开始。此外,如果您有不同的数据集要处理,您可以遵循类似的模式,甚至可以将 CSV 从 Google Cloud Storage 存储桶加载到 BigQuery 中。
第一步是从公共数据源创建一个新表。
from google.cloud import bigquery
# Create bigquery client
bq = bigquery.Client(project=PROJECT_ID)
TABLE_NAME = input("Input a Big Query TABLE_NAME:") #hackernews
DATASET_ID = f"{PROJECT_ID}.google_redis_llms"
# Create dataset
dataset = bigquery.Dataset(DATASET_ID)
dataset.location = "US"
dataset = bq.create_dataset(dataset, timeout=30, exists_ok=True)
# Define table ID
TABLE_ID = f"{DATASET_ID}.{TABLE_NAME}"
# Create source table
def create_source_table(table_id: str):
create_job = bq.query(f'''
CREATE OR REPLACE TABLE `{table_id}` AS (
SELECT
title, text, time, timestamp, id
FROM `bigquery-public-data.hacker_news.full`
WHERE
type ='story'
LIMIT 1000
)
''')
res = create_job.result() # Make an API request
table = bq.get_table(table_id)
return table
# Create table
table = create_source_table(TABLE_ID)
# List schema
table.schema
上面的数据集包含黑客新闻帖子的记录,包括标题、文本、时间、id和时间戳。让我们提取一些示例行并进行检查。
# Query 5 sample records from BQ
query_job = bq.query(f'''
SELECT *
FROM {TABLE_ID}
LIMIT 5
''')
query_job.result().to_dataframe()
具体表格数据见操作笔记
3. 生成 Embeddings
使用 Vertex AI 嵌入模型创建文本嵌入
使用 Google 开发的 Vertex AI API for text embeddings。
如需详细了解嵌入,请参阅 Meet AI 的多工具:向量嵌入。如需学习有关嵌入的基础机器学习速成课程,请参阅嵌入
使用gpc 进行相关embeddings的case: https://github.com/GoogleCloudPlatform/generative-ai/tree/main/embeddings
文本嵌入是内容的密集向量表示,如果两个内容在语义上相似,它们各自的嵌入在嵌入向量空间中的位置也相近。这种表示可以用来解决常见的自然语言处理(NLP)任务,例如:
- 语义搜索:根据语义相似性对搜索文本进行排名。
- 推荐:返回与给定文本具有相似文本属性的项目。
- 分类:返回与给定文本相似的文本属性的项目类别。
- 聚类:对与给定文本的文本属性相似的项目进行聚类。
- 异常检测:返回与给定文本的文本属性关系最小的项目。
textembedding-gecko
模型接受最多 3,072 个输入标记(即单词),并输出 768 维的向量嵌入。
定义嵌入辅助函数
我们定义了一个辅助函数 embedding_model_with_backoff
,用于从文本列表创建嵌入,同时通过指数退避来使其对Vertex AI API 配额具有弹性,使用tenacity库。
我们还定义了一种方法,将浮点数数组转换为字节字符串,以便在 Redis 中高效存储(稍后使用)。
from typing import Generator, List, Any
from tenacity import retry, stop_after_attempt, wait_random_exponential
from vertexai.preview.language_models import TextEmbeddingModel
# Embedding model definition from VertexAI PaLM API
embedding_model = TextEmbeddingModel.from_pretrained("textembedding-gecko@001")
VECTOR_DIMENSIONS = 768
@retry(wait=wait_random_exponential(min=1, max=20), stop=stop_after_attempt(3))
def embed_text(text=[]):
embeddings = embedding_model.get_embeddings(text)
return [each.values for each in embeddings]
# Convert embeddings to bytes for Redis storage
def convert_embedding(emb: List[float]):
return np.array(emb).astype(np.float32).tobytes()
嵌入文本数据
目前,我们在 BigQuery 中创建的表(如上所述)包含了我们希望嵌入并为大型语言模型(LLMs)提供可用的黑客新闻文章记录。
为了节省这台机器的 RAM 使用量,我们将分批次迭代 BigQuery 中的文章,创建嵌入,并将它们写入 Redis,Redis 在此被用作向量数据库。
import pandas as pd
import numpy as np
QUERY_TEMPLATE = f"""
SELECT id, title, text
FROM {TABLE_ID}
LIMIT {{limit}} OFFSET {{offset}};
"""
def query_bigquery_batches(
max_rows: int,
rows_per_batch: int,
start_batch: int = 0
) -> Generator[pd.DataFrame, Any, None]:
# Generate batches from a table in big query
for offset in range(start_batch, max_rows, rows_per_batch):
query = QUERY_TEMPLATE.format(limit=rows_per_batch, offset=offset)
query_job = bq.query(query)
rows = query_job.result()
df = rows.to_dataframe()
# Join title and text fields
df["content"] = df.apply(lambda r: "Title: " + r.title + ". Content: " + r.text, axis=1)
yield df
在下面,我们定义了一些辅助函数,用于处理单个数据行、将批次写入 Redis、从 BigQuery 查询源数据,以及使用 Vertex AI 创建文本嵌入。
import math
from tqdm.auto import tqdm
# Redis key helper function
def redis_key(key_prefix: str, id: str) -> str:
return f"{key_prefix}:{id}"
# Process a single dataset record
def process_record(record: dict) -> dict:
return {
'id': record['id'],
'embedding': record['embedding'],
'text': record['text'],
'title': record['title']
}
# Load batch of data into Redis as HASH objects
def load_redis_batch(
redis_client: redis.Redis,
dataset: list,
key_prefix: str = "doc",
id_column: str = "id",
):
pipe = redis_client.pipeline()
for i, record in enumerate(tqdm(dataset)):
record = process_record(record)
key = redis_key(key_prefix, record[id_column])
pipe.hset(key, mapping=record)
pipe.execute()
# Run the entire process
def create_embeddings_bigquery_redis(redis_client):
# Create generator from BigQuery
max_rows = 1000
rows_per_batch = 100
bq_content_query = query_bigquery_batches(max_rows, rows_per_batch)
for batch in tqdm(bq_content_query):
# Split batch into smaller chunks for embedding generation
batch_splits = np.array_split(batch, math.ceil(rows_per_batch/5))
# Create embeddings
batch["embedding"] = [
convert_embedding(embedding)
for split in batch_splits
for embedding in embed_text(split.content)
]
# Write batch to Redis
batch = batch.to_dict("records")
load_redis_batch(redis_client, batch)
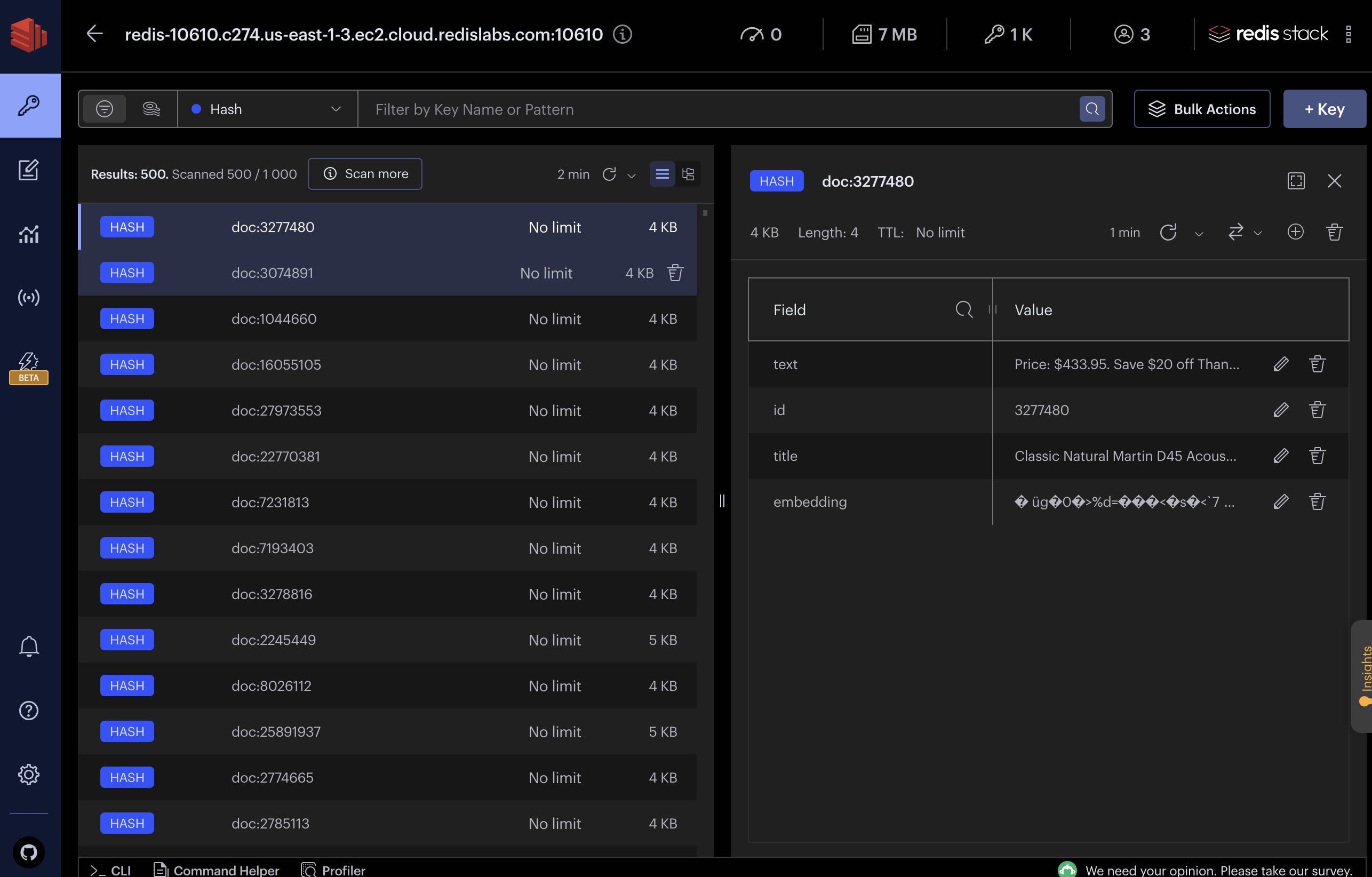
4. 加载嵌入
现在我们已经有了一个函数来生成 BigQuery 批次、创建文本嵌入以及将批次写入 Redis,我们可以运行这一个函数来处理我们整个数据集:
create_embeddings_bigquery_redis(redis_client)
# Validate how many records are stored in Redis
redis_client.dbsize()
5. 创建向量索引
现在我们已经创建了代表我们数据集中文本的嵌入,并将它们存储在 Redis 中,我们将创建一个辅助索引,以实现对嵌入的高效搜索。要了解更多关于 Redis 中向量相似性功能的信息,请查看这些文档 以及 这些 Redis AI 资源。
为什么我们需要启用搜索功能呢? 使用 Redis 进行向量相似性搜索可以让我们检索与输入问题或查询相似或相关的文本数据块。这对于我们的示例生成式 AI / LLM 应用程序来说将非常有帮助。
from redis.commands.search.field import (
NumericField,
TagField,
TextField,
VectorField,
)
from redis.commands.search.indexDefinition import IndexDefinition, IndexType
from redis.commands.search.query import Query
INDEX_NAME = "google:idx"
PREFIX = "doc:"
VECTOR_FIELD_NAME = "embedding"
# Store vectors in redis and create index
# 对hash中的 embedding字段建向量索引FLAT,维度768,相似度测量方法:cosine
def create_redis_index(
redis_client: redis.Redis,
vector_field_name: str = VECTOR_FIELD_NAME,
index_name: str = INDEX_NAME,
prefix: list = [PREFIX],
dim: int = VECTOR_DIMENSIONS #768
):
# Construct index
try:
redis_client.ft(index_name).info()
print("Existing index found. Dropping and recreating the index", flush=True)
redis_client.ft(index_name).dropindex(delete_documents=False)
except:
print("Creating new index", flush=True)
# Create new index
redis_client.ft(index_name).create_index(
(
VectorField(
vector_field_name, "FLAT",
{
"TYPE": "FLOAT32",
"DIM": dim,
"DISTANCE_METRIC": "COSINE",
}
)
),
definition=IndexDefinition(prefix=prefix, index_type=IndexType.HASH)
)
# Create index
create_redis_index(redis_client)
# Inspect index attributes
redis_client.ft(INDEX_NAME).info()
# Retreive single HASH from Redis
key = redis_client.keys()[1]
print(key)
redis_client.hgetall(key)
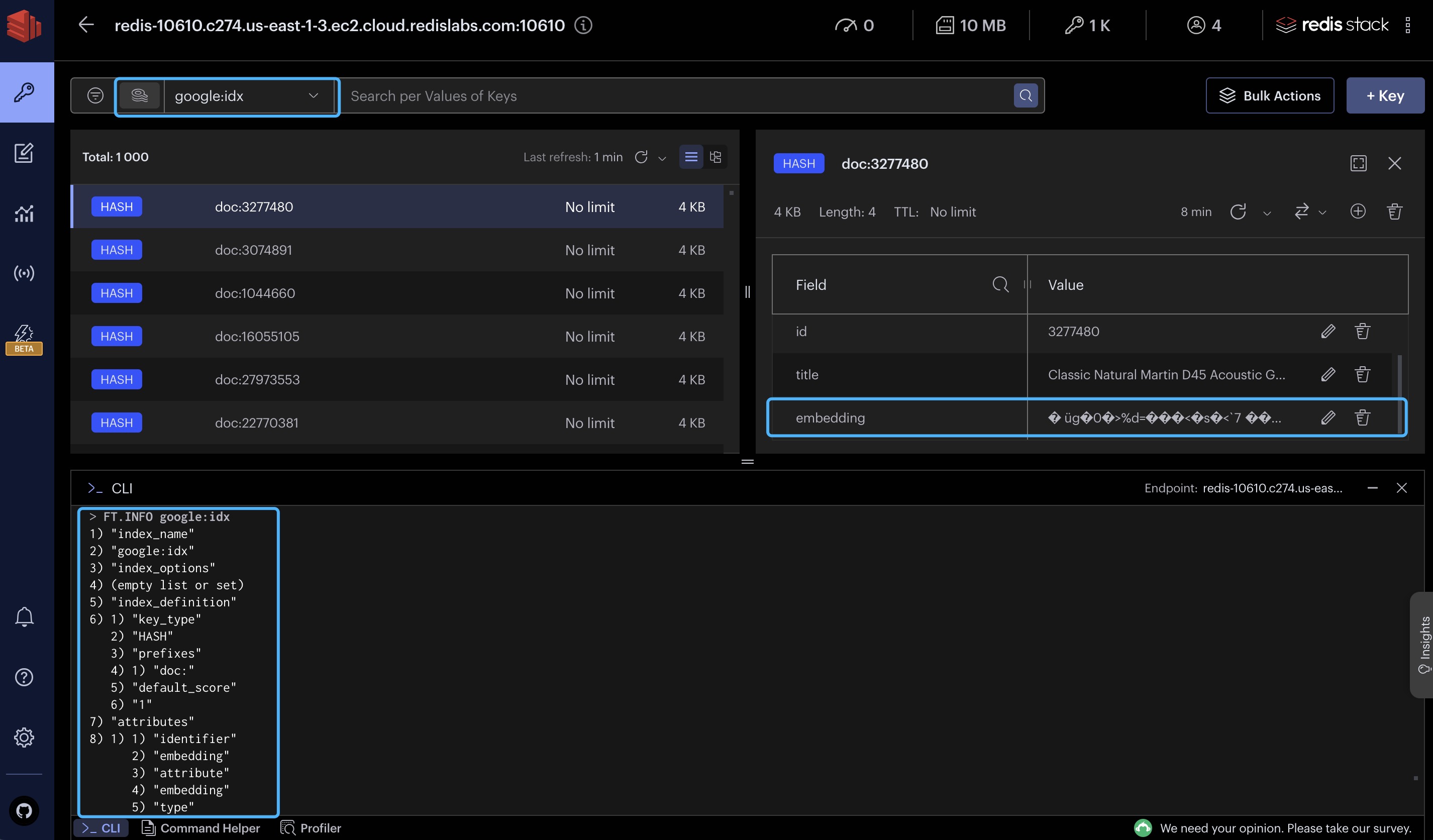
Redis数据存储完全加载了来自BigQuery的数据子集,包括使用Vertex AI PaLM api创建的文本嵌入。
构建 LLM 应用程序
随着 Redis 作为向量数据库完全加载,并且我们可以利用强大的 PaLM API,我们可以在这个技术栈上构建许多 AI 应用程序。下面我们将简要描述每个应用程序及其用例:
- 文档检索 - 搜索文档,只返回与给定查询最相关的文档。
- 产品推荐 - 根据购物者喜欢的产品的属性和描述,推荐具有相似属性和描述的产品。
- 聊天机器人 - 提供一个对话式界面,用于信息检索或客户服务。
- 文本摘要与生成 - 从相关信息源生成新的内容,以加速团队产出。
- 欺诈/异常检测 - 基于与其他已知实体的属性相似性,识别异常和潜在的欺诈事件、交易或项目。
更多应用case见:https://github.com/redis-developer/redis-ai-resources
LLM 设计模式
为了构建这类应用程序,下面我们强调了四种技术设计模式和技巧,Redis Enterprise 在这些方面非常有用,可以提升 LLM 的性能:
- 语义搜索
- 检索增强生成(Retrieval Augmented Generation,RAG)
- 缓存
- 内存
结合这些模式的某种组合是推荐的最佳实践,这些实践来自于全球各地企业用例和开源用户的经验和总结。
简单的语义搜索
语义搜索,在大型语言模型(LLMs)的背景下,是一种复杂的搜索技术,它超越了字面上的关键词匹配,以理解用户查询背后的上下文含义和意图。利用 Google Vertex AI 平台的强大能力和 Redis 向量数据库的功能,语义搜索可以从大量文本数据集中映射和提取深层次的知识,包括细微的关系和隐藏的模式。
这使得应用程序能够返回在上下文中相关的搜索结果,通过提供有意义的回应,增强了用户体验,即使是对复杂或模糊的搜索词也是如此。因此,语义搜索不仅提高了搜索结果的准确性和相关性,还使应用程序能够以更类似人类、直观的方式与用户互动。
语义搜索的一般过程包括三个步骤:
- 创建查询向量
- 执行向量搜索
- 审查并返回结果
# 1. Create query vector
query = "What is the best computer operating system for software dev?"
query_vector = embed_text([query])[0]
# Our query has been converted to a list of floats (this is a truncated view)
query_vector[:10]
# Helper method to perform KNN similarity search in Redis
def similarity_search(query: str, k: int, return_fields: tuple, index_name: str = INDEX_NAME) -> list:
# create embedding from query text
query_vector = embed_text([query])[0]
# create redis query object
redis_query = (
Query(f"*=>[KNN {k} @{VECTOR_FIELD_NAME} $embedding AS score]")
.sort_by("score")
.return_fields(*return_fields)
.paging(0, k)
.dialect(2)
)
# execute the search
results = redis_client.ft(index_name).search(
redis_query, query_params={"embedding": convert_embedding(query_vector)}
)
return pd.DataFrame([t.__dict__ for t in results.docs ]).drop(columns=["payload"])
# 2. Perform vector similarity search with given query
results = similarity_search(query, k=5, return_fields=("score", "title", "text"))
# 3. Review and return the results
display(results)
具体表格数据见操作笔记
上述结果表明,我们针对软件开发者推荐的操作系统的搜索,找到了一些可能有助于回答这个问题的 Hacker News 的帖子。可尝试使用不同的索引类型和距离度量
检索增强生成(RAG)
检索增强生成(Retrieval Augmented Generation,RAG)在大型语言模型(LLMs)的范围内,是一种结合特定领域数据知识和生成模型的技术,用于增强产生丰富上下文问题回答的能力。本质上,RAG 通过从文档或数据的知识库中检索相关信息,然后再生成回答的方式进行工作。这使得通用的基础模型能够在运行时访问这些数据源,这与微调不是同一回事。
RAG 利用 Redis 作为低延迟向量数据库的优势进行高效的检索操作,以及利用 Google 的 Vertex AI 生成连贯的文本回答。在 LLM 应用程序中,RAG 使得对上下文的深入理解成为可能,即使是对复杂的查询也能返回高度细腻的回答。这种模式增强了应用程序的交互能力,提供了更精确和信息丰富的回答,从而显著丰富了用户体验。
为了构建一个用于问答的RAG管道,我们需要使用Vertex PaLM API来生成文本(text-bison@001
)。
from vertexai.preview.language_models import TextGenerationModel
# Define generation model
generation_model = TextGenerationModel.from_pretrained("text-bison@001")
response = generation_model.predict(prompt="What is a large language model?")
print("Example response:\n", response.text)
为了能够在引用特定领域的来源(如我们的示例 hackernews 数据集)的同时回答问题,我们必须构建一个 RAG 管道:
-
首先在知识库(存储在 Redis 中)上使用用户查询进行语义搜索,以找到有助于语言模型智能回答的相关来源。
-
这些来源(称为上下文)被“塞入”提示(输入)中。
-
最后,完整的提示被传递给语言模型进行文本生成。
def create_prompt(prompt_template: str, **kwargs) -> str:
return prompt_template.format(**kwargs)
def rag(query: str, prompt: str, verbose: bool = True) -> str:
"""
Simple pipeline for performing retrieval augmented generation with
Google Vertex PaLM API and Redis Enterprise.
"""
# Perform a vector similarity search in Redis
if verbose:
print("Pulling relevant data sources from Redis", flush=True)
relevant_sources = similarity_search(query, k=3, return_fields=("text",))
if verbose:
print("Relevant sources found!", flush=True)
# Combine the relevant sources and inject into the prompt
sources_text = "-" + "\n-".join([source for source in relevant_sources.text.values])
full_prompt = create_prompt(
prompt_template=prompt,
sources=sources_text,
query=query
)
if verbose:
print("\nFull prompt:\n\n", full_prompt, flush=True)
# Perform text generation to get a response from PaLM API
response = generation_model.predict(prompt=full_prompt)
return response.text
下面是一个示例提示模板。您可以随意编辑和调整为语言模型设置上下文的初始句子,以执行我们预期的操作。对提示设计进行调整和迭代的过程通常被称为“提示工程”。
PROMPT = """You are a helpful virtual technology and IT assistant. Use the hacker news posts below as relevant context and sources to help answer the user question. Don't blindly make things up.
SOURCES:
{sources}
QUESTION:
{query}?
ANSWER:"""
query = "What are the best operating systems for software development?"
response = rag(query=query, prompt=PROMPT)
print(response)
query = "What are some commonly reported problems with MacBooks and MacOS for software dev purposes?"
response = rag(query=query, prompt=PROMPT)
print(response)
显然,这个示例数据集(hackernews)并不是我们可以使用的唯一示例,它当然也不是现成的“production”。这也只是为了教学目的而使用了实际数据的一个子集(1000条记录)。
但是,这个示例演示了如何将外部数据源和llm结合起来,以显示更有用的信息。
LLM 缓存
LLM 缓存是一种用于优化大型语言模型(LLM)应用程序性能的高级策略。利用 Redis 的超快速内存数据存储功能,LLM 缓存能够存储并快速检索由 Google 的 Vertex AI(PaLM)生成的预先计算的响应。这意味着,尤其是对于重复查询,生成响应的计算成本高昂的过程被显著减少,从而实现更快的响应时间和高效的资源利用。因此,将 Google 强大的生成式 AI 能力与 Redis 高性能的缓存系统相结合,为 LLM 应用程序提供了一个更具可扩展性和性能的架构,提高了整体用户体验和应用程序的可靠性。
LLM 的缓存主要有两种模式:
LLM 的标准缓存涉及简单地匹配以前提供过的确切短语或提示。我们可以返回之前使用的 LLM 响应,以加快系统的整体吞吐量并减少冗余计算。
# Some boiler plate helper methods
import hashlib
def hash_input(prefix: str, _input: str):
return prefix + hashlib.sha256(_input.encode("utf-8")).hexdigest()
def standard_check(key: str):
# function to perform a standard cache check
res = redis_client.hgetall(key)
if res:
return res[b'response'].decode('utf-8')
def cache_response(query: str, response: str):
key = hash_input("llmcache:", query)
redis_client.hset(key, mapping={"prompt": query, "response": response})
# LLM Cache wrapper / decorator function
def standard_llmcache(llm_callable):
def wrapper(*args, **kwargs):
# Check LLM Cache first
key = hash_input("llmcache:", *args, **kwargs)
response = standard_check(key)
# Check if we have a cached response we can use
if response:
return response
# Otherwise execute the llm callable here
response = llm_callable(*args, **kwargs)
cache_response(query, response)
return response
return wrapper
# Define a function that invokes the PaLM API wrapped with a cache check
@standard_llmcache
def ask_palm(query: str):
prompt = PROMPT
response = rag(query, prompt, verbose=False)
return response
query = "What are the best operating systems for software development?"
ask_palm(query)
现在,如果我们再问同样的问题,我们应该会得到几乎实时的相同回答。
%%time # for ipynb
ask_palm(query)
语义缓存 (Semantic Caching)
语义缓存基于上述相同的概念,但利用 Redis 的向量数据库特性来查找以前应用过的语义上相似的提示——这使得缓存那些在意义上非常相似但不一定使用完全相同单词和短语的查询的响应成为可能。这提高了“命中率”,使得 LLM 缓存在实践中更加有效。
在langchain开发框架中集成:https://python.langchain.com/docs/integrations/llms/llm_caching#semantic-cache
为应用程序提供对“历史记录”的访问,以记录聊天历史,是一种常见的技术,用于提高模型推理最近或过去对话的能力,从之前的回答中获得上下文,从而提供更准确和可接受的回应。
下面我们设置了简单的辅助函数来在Redis List数据结构中持久化和加载会话历史记录。
import json
def add_message(prompt: str, response: str):
msg = {
"prompt": prompt,
"response": response
}
redis_client.lpush("chat-history", json.dumps(msg))
def get_messages(k: int = 5):
return [json.loads(msg) for msg in redis_client.lrange("chat-history", 0, k)]
query = "Do you have any advice for getting started in the tech field as a software dev?"
response = rag(query, PROMPT, verbose=False)
print(response)
add_message(query, response)
query = "What if I am still in college, any tips there?"
response = rag(query, PROMPT, verbose=False)
print(response)
add_message(query, response)
get_messages()
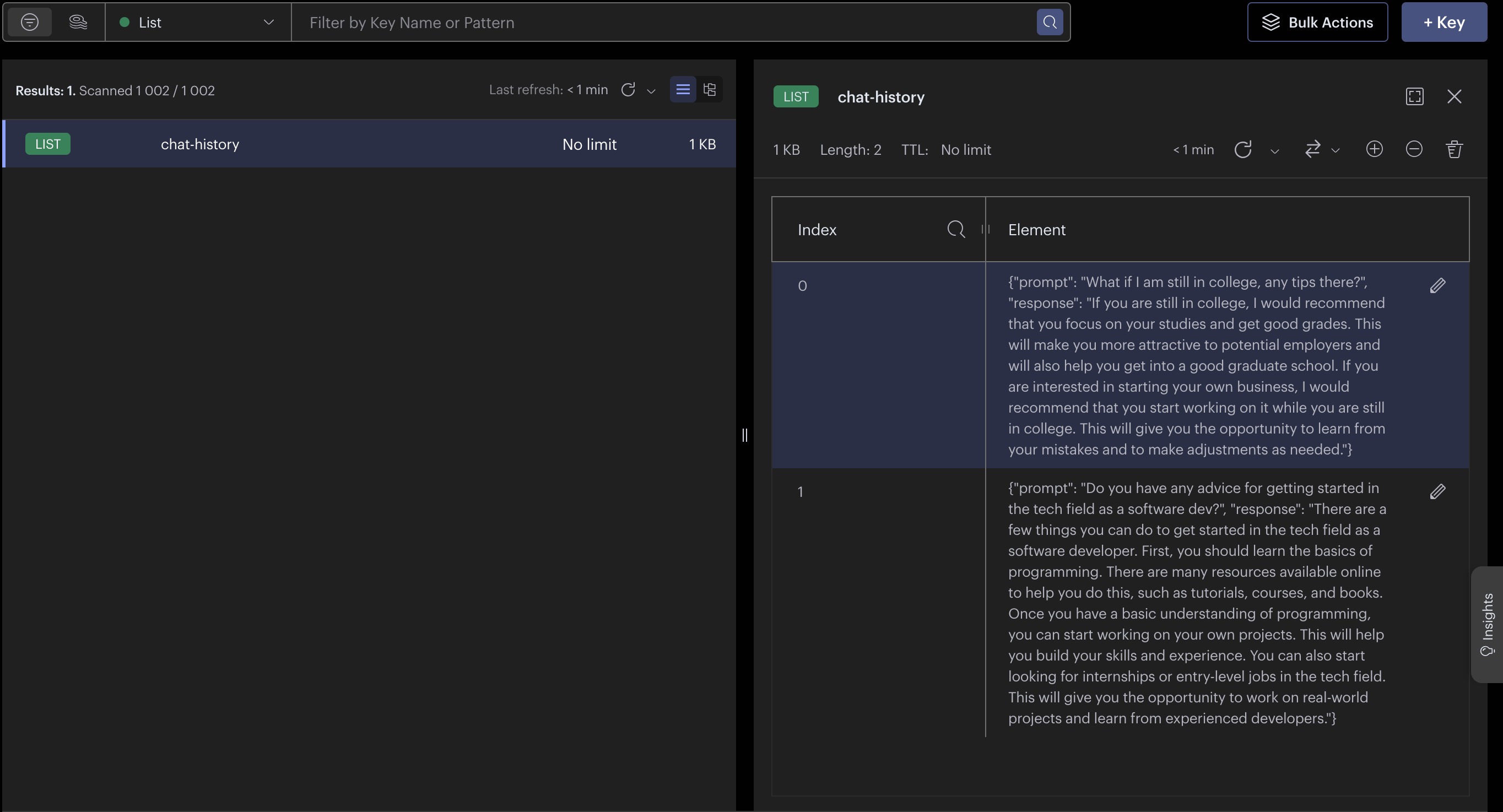
清理bigquery 创建的表
# Clean up bigquery
bq.delete_table(TABLE_ID, not_found_ok=True)
bq.delete_dataset(
DATASET_ID, delete_contents=True, not_found_ok=True
)
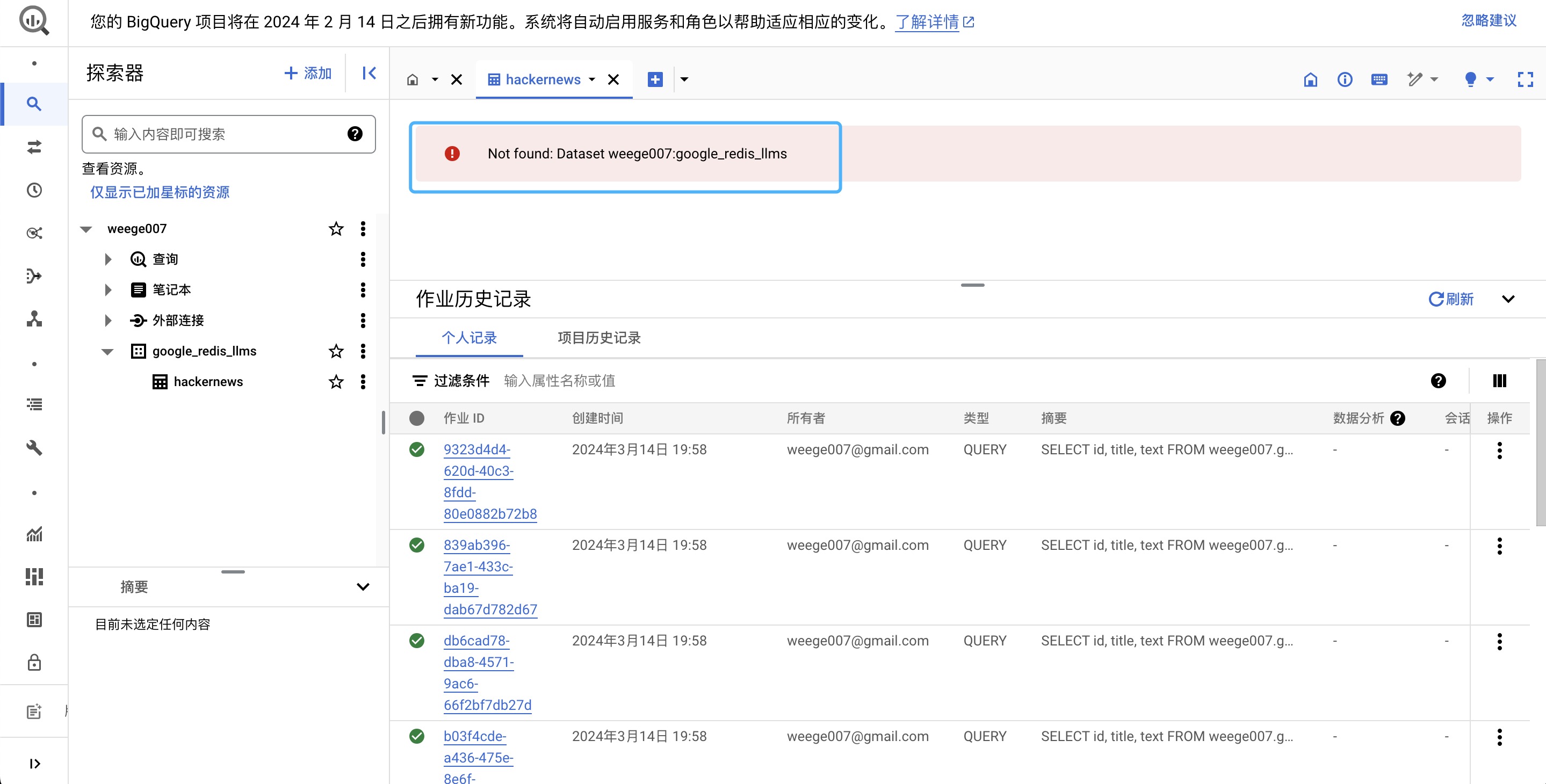
清理redis中的数据,关闭服务
# Clean up redis
#!redis-stack-server stop
redis_client.flushdb();
redis_client.close();
Recommend
About Joyk
Aggregate valuable and interesting links.
Joyk means Joy of geeK