

What role does AI play in recommendation systems and engines?
source link: https://www.algolia.com/blog/ai/what-role-does-ai-play-in-recommendation-systems-and-engines/
Go to the source link to view the article. You can view the picture content, updated content and better typesetting reading experience. If the link is broken, please click the button below to view the snapshot at that time.
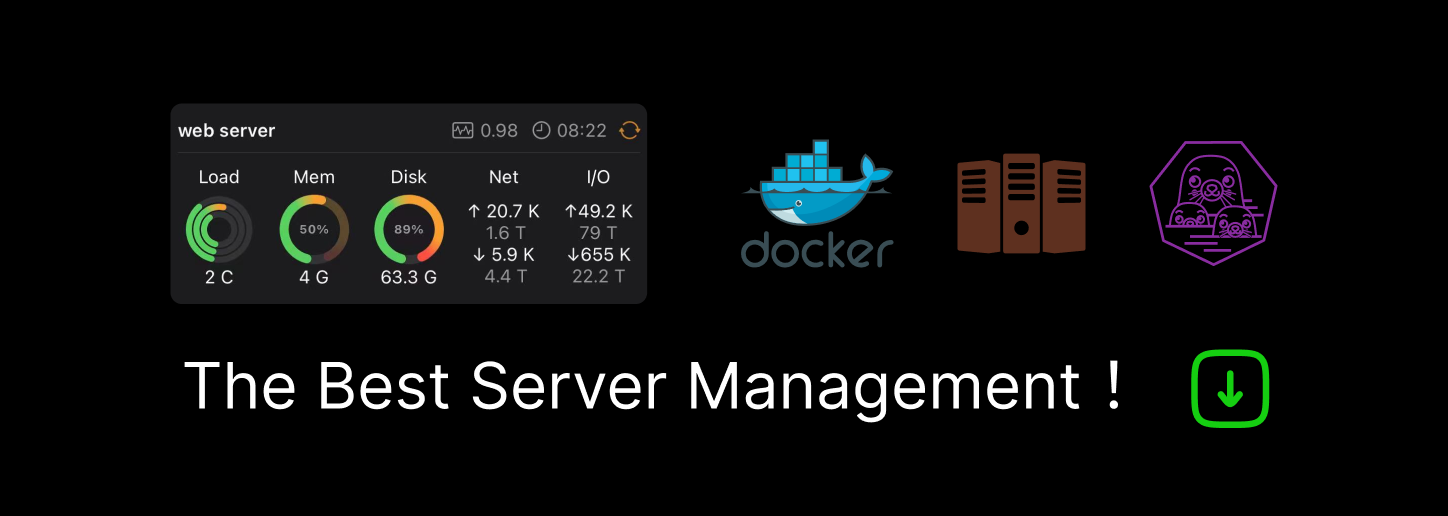
What role does AI play in recommendation systems and engines?
Feb 14th 2024ai
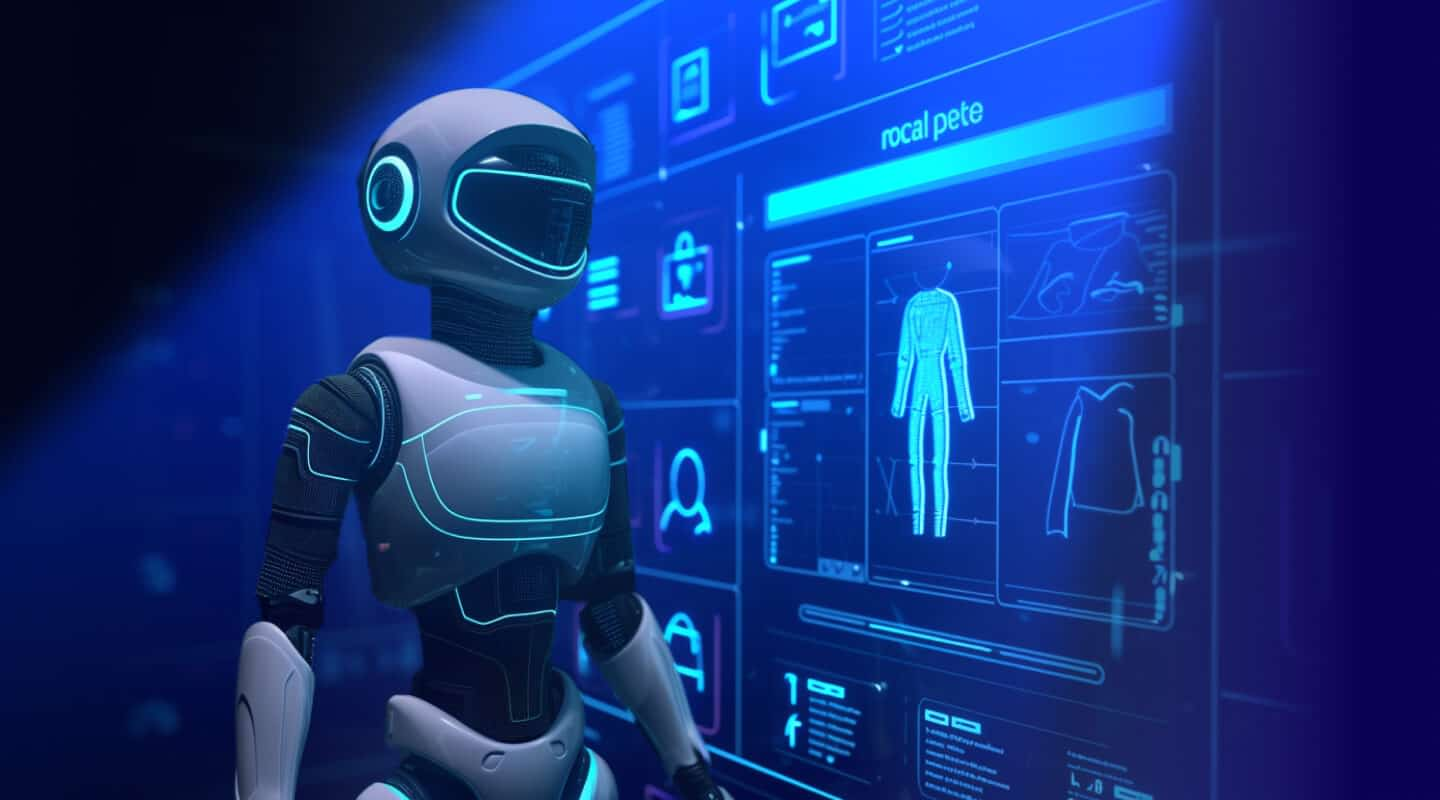
You put that in your cart. How about this cool thing to go with it?
You liked that? Here are some other items frequently bought together by our customers.
If an AI-aided recommendations engine could talk, it might say things like this. After all, an AI-powered system means a smarter one than the traditional kind, right?
Of course.
Modern recommendation systems, which have sprung from the efforts of one person attempting to declutter their email in-box to create a major Internet phenomenon, are complex. They’re also ubiquitous: in this era of customer data collection and Big Data applications, millions of consumers use them on a daily basis.
How are they different now from when they first became a thing?
Historically, recommendation models have reflected users’ current actions — the most recent item clicked, the current search term. But now, with the rise of artificial intelligence, they’re considerably more sophisticated. With AI models, tracking the sequence of user behavior provides considerably richer insight on a specific user’s goals and interests.
AI-based solutions are attuned to the ways companies target customers interact with products and services during a given session. Specific search terms, clicks, conversions, and other trackable events from a visitor’s current session help create relevant recommendations tailored to what they’re seeking.
What’s an AI-powered recommendation system?
An AI-powered recommendation system, then, is an algorithm, typically aided by machine learning, that suggests content based on amassed user data, such as purchase history, clicks, conversions, and demographics. With this technology, a recommendation engine comes up with personalized suggestions for people based on data collected about their activities on a website or in an app.
And, as with many things, when it comes to recommendation systems, AI is nothing short of a game changer if you want to enhance your user engagement. There’s no comparison with the quality of what’s recommended compared with traditional recommendation technology.
How does AI improve a recommendation engine?
The secret is machine-learning algorithms that use statistical models. AI-aided recommendation systems:
- Analyze user data, pinpointing patterns
- Handle more-complex data representations
- Enable insights on how people and items are related
- Make predictions based on knowing shopping habits and preferences
- Expand options for consumers through making personalized recommendations
Types of recommendation engines
Some recommender systems do collaborative filtering, some do content-based filtering, and some do both.
Collaborative filtering
A collaborative filtering recommender engine collects and analyzes data on people’s behavior, activities, and preferences, predicting what they’ll like based on their actions’ similarity to those of other users.
There are two types of collaborative filtering systems.
With memory-based collaborative filtering:
- Clusters of users are identified
- Interactions are used to predict actions of similar users
- Clusters of items rated by user A are identified and used to predict interaction with similar item B
- Matrix sparsity can be problematic: the number of user-item interactions may be too low to generate high-quality clusters
With model-based collaborative filtering:
- Machine learning and data-mining techniques are at the core
- Models are trained to make predictions
- A system like this can recommend more items to more people
Within the AI recommendations ecosystem, collaborative filtering algorithms fall into the categories of user, item, hybrid, and matrix factorization. Each is best suited for different types of data sets depending on the number of users, type of items, density of ratings per user, and item categories.
Note: With collaborative filtering, a new user or introduction of a product is a cold-start problem. You might decrease lag time by asking users for data when they land on your home page and sign up, then pair that with available item metadata to relate the new user to existing items.
Content-based filtering
When filtering according to content, similar products are grouped together based on their features. Recommendation algorithms take into account the user’s preferences plus descriptions of items (e.g., product type, color, word length, genre). You know a content-based recommender system is being used when the interface tells you “If you like that, you might also like this.” Or when it shows you an item you were interested in because it’s looking at your past behavior, “thinking” you might still be interested.
What are the drawbacks of a recommendation engine using content-based filtering?
- You have limited insight; you can only recommend what’s similar to something being viewed, bought, or used
- If user interaction shows that someone is interested in only certain categories, the system has no way to reliably recommend items in other categories
- There’s not enough data about new users when they arrive on the site or start using the app
Hybrid filtering
A hybrid recommender system utilizes both collaborative and content-based data. Not surprisingly, its superior filtering workflow produces the best suggestions.
Netflix is a great case study of a company that’s doing it all: it uses hybrid recommendation filtering on its website to improve its customer experience. It also accounts for subscribers’ interests (using collaborative filtering) and for movie descriptions and features (using content-based filtering).
Benefits of AI in recommendation systems
Increasing average order value
There’s no doubt that offering AI-driven recommendations can improve shopping-cart AOV. If a retailer can use cross-sells, for instance, to generate customer interest and sell the person multiple items instead of just one, their profit margins improve.
Ecommerce sites that utilize recommendation engines can drive conversions by suggesting:
- Additional items that other shoppers have bought along with the item being considered
- Items that align with the person’s search query content
- Relevant products that naturally seem to “go with” something in their cart
Aiding discovery
With non-AI recommendations, people were expected to know what they wanted and be able to accurately describe it. With AI-based recommendations, people may never have sought out items or made item discoveries on their own. In other words, the AI element is responsible for educating people about new options they never knew they had, enhancing the end-to-end customer experience on the site. Being able to materially aid people’s discovery — in essence, using automation to upsell and cross-sell — is an unparalleled advantage that can translate into some pretty major financial gains.
Boosting conversions
Due to their track record for improving conversion rates and sales, product recommendation engines are one of the most popular applications of data science. They can be very lucrative: recommendations account for up to 31 percent of ecommerce revenues (Barilliance). On average, 12 percent of customers’ overall purchases are attributed to them.
On Netflix, for instance, 80% of movies watched are generated by the company’s content-recommendation technology. And Netflix’s personalized recommendation system was a factor in a revenue increase of $1 billion in 2021.
Protecting privacy
AI recommendations work best with plenty of data from your site or app. The first-party data you collect has many upsides in terms of data security and privacy and providing a unique user experience for your customers. The recommendations functionality learns based on collected past user behavior, then makes predictions according to a model.
AI recommendations use cases
How widely used are AI recommendation systems? Here are just a few domains in which they’re being applied:
- Ecommerce sites like Amazon are natural places for personalized product recommendations that improve customer satisfaction and retention
- Media companies. Highlighted by Netflix’s success with on-demand movie and TV show recommendations, media subscription sites are a natural place for AI suggestions
- Social media sites strive to engage people through giving them relevant content, so on-point smart recommendations are a big help
- In healthcare, recommendation engines help providers suggest appropriate medication and courses of treatment
Recommended for you
Getting interested in harnessing AI-aided recommendations to take your business to the next level?
As part of your decision-making, consider this: Algolia AI Recommendations, our state-of-the-art API’s dynamic recommendations feature, enables near-real-time product discovery with scalability.
Implemented strategically, it enhances customer engagement, which we’ve calculated leads to a 150% increase in order rate, 13% increase in overall site conversion, and 24% lower bounce rate.
Intrigued by these metrics? Contact us and let’s get you started with recommendations optimization and higher conversion.
Recommend
About Joyk
Aggregate valuable and interesting links.
Joyk means Joy of geeK