

Tensor DSL总结
source link: https://zhen8838.github.io/2023/12/20/tensordsl/
Go to the source link to view the article. You can view the picture content, updated content and better typesetting reading experience. If the link is broken, please click the button below to view the snapshot at that time.
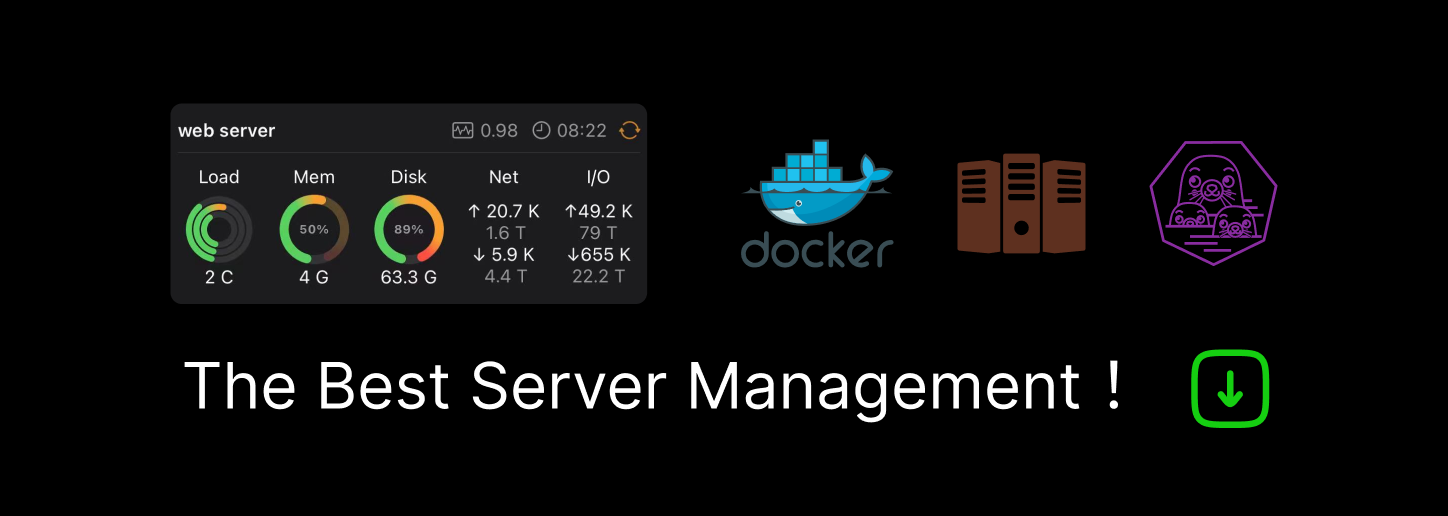
Tensor DSL总结
本文旨在总结一些张量优化的DSL是如何设计的, 尝试从其中发现一些共同点.
接下来我将统一使用Matmul(Transpose(Conv(lhs)),rhs)
的例子在不同的框架中进行测试.
1. Jittor
1.1 DSL语法
首先结合论文中的例子讲一下reindex
的原理:
def conv(x, p):
N,C,H,W = x.shape
o,i,h,w = p.shape
xx = x.reindex(shape=(N,o,H,W,i,h,w),
indexes=("i0", "i4", "i2-i5", "i3-i6") )
pp = p.broadcast(xx.shape, dims=(0,2,3))
yy = xx*pp
y = yy.sum(dims=(4,5,6))
return y
这里其实是把shape
看作为循环层级,
这里的reindex
相当于在7层循环的最内层中做类似xx[N,o,H,W,i,h,w] = x[N,i,H-h,W-w]
的索引.
然后再把weights
也通过boradcast
扩展到同样的循环层级pp[N,o,H,W,i,h,w] = p[o,i,h,w]
,
在7层循环内部执行xx[N,o,H,W,i,h,w]*pp[N,o,H,W,i,h,w]
的操作,
等价于执行x[N,i,H-h,W-w] * p[o,i,h,w]
,
然后对i,h,w
三层循环做求和.
可以说通过reindex
+broadcast
操作,
完成了类似于polyhedral
中2d+1表示中的loop dimension align
和修改access relation
(由indexes
指定).
Jittor
这里并没有考虑让开发者自行调度算子,
后续的优化都交给编译器自动化.
1.2 测试例子
import jittor as jt
def conv(x, p):
N,C,H,W = x.shape
o,i,h,w = p.shape
xx = x.reindex(shape=(N,o,H,W,i,h,w),
indexes=("i0", "i4", "i2-i5", "i3-i6") )
pp = p.broadcast(xx.shape, dims=(0,2,3))
yy = xx*pp
y = yy.sum(dims=(4,5,6))
return y
def matmul(a,b):
bc, c, m,k = a.shape
_, _, _,n = b.shape
shape = [bc, c, m, k, n]
a = a.broadcast(shape, [-1]) # [m,k, ] -> [m,k,n]
b = b.broadcast(shape, [-3]) # [ ,k,n] -> [m,k,n]
return (a*b).sum(-2)
lhs = jt.randn(8,3,32,32)
kernel = jt.randn(16,3,3,3)
rhs = jt.randn(8,32,16,64)
jt.flags.compile_options={"compile_shapes":1}
with jt.profile_scope() as report:
output = matmul(jt.transpose(conv(lhs, kernel), [0,2,3,1]), rhs).fetch_sync()
jt.flags.compile_options={}
编译后得到:
Profile result, sorted by TotalTime
('it/s' represent number of iterations per sec)
Name FileName Count TotalTime %,cum% AvgTime MinTime MaxTime Input Output InOut Compute
Total time: 12.8ms
Total Memory Access: 6.19MB
[opkey0:broadcast_to[Tx:float32][DIM=7][BCAST=d][JIT:1][JIT_cpu:1][index_t:int32]][opkey1:reindex[Tx:float32][XDIM=4][YDIM=7][OVERFLOW:itof(0x0)][INDEX0:i0][INDEX1:i4][INDEX2:i2-i5][INDEX3:i3-i6][OSIZE=0][ESIZE=0][JIT:1][JIT_cpu:1][index_t:int32]][opkey2:binary[Tx:float32][Ty:float32][Tz:float32][OP:multiply][JIT:1][JIT_cpu:1][index_t:int32]][opkey3:reduce[Tx:float32][Ty:float32][Tz:float32][OP:add][DIM=7][REDUCE=70][JIT:1][JIT_cpu:1][index_t:int32]][JIT:1][JIT_cpu:1][graph:040000,062010,010020,000021,020030,][var_info::041704171724][shapes:[10,3,3,3,],[8,10,20,20,3,3,3,],[8,3,20,20,],[8,10,20,20,3,3,3,],[8,10,20,20,3,3,3,],[8,10,20,20,],][choices:compile_shapes:1,]
/root/.cache/jittor/jt1.3.1/g++10.5.0/py3.8.18/Linux-5.4.0-42xae/AMDEPYC7T8364-x8f_debug/default/jit/_opkey0_broadcast_to_Tx_float32__DIM_7__BCAST_d__JIT_1__JIT_cpu_1__index_t_int32___opkey1____hash_a2d65b1fd1c3f3d0_op.cc
1 8.12ms(63.3%,63.3%) 8.12ms 8.12ms 8.12ms 11.7MB/s 61.6MB/s 73.3MB/s 436Mit/s
random[T:float32][R:normal][JIT:1][JIT_cpu:1][index_t:int32]
/root/.cache/jittor/jt1.3.1/g++10.5.0/py3.8.18/Linux-5.4.0-42xae/AMDEPYC7T8364-x8f_debug/default/jit/random_T_float32__R_normal__JIT_1__JIT_cpu_1__index_t_int32__hash_c27874d0aacc5d25_op.cc
3 3.68ms(28.7%,91.9%) 1.23ms 5.58us 3.37ms 0 B/s 298MB/s 298MB/s 78Mit/s
[opkey0:broadcast_to[Tx:float32][DIM=5][BCAST=4][JIT:1][JIT_cpu:1][index_t:int32]][opkey1:broadcast_to[Tx:float32][DIM=5][BCAST=10][JIT:1][JIT_cpu:1][index_t:int32]][opkey2:binary[Tx:float32][Ty:float32][Tz:float32][OP:multiply][JIT:1][JIT_cpu:1][index_t:int32]][opkey3:reduce[Tx:float32][Ty:float32][Tz:float32][OP:add][DIM=5][REDUCE=8][JIT:1][JIT_cpu:1][index_t:int32]][JIT:1][JIT_cpu:1][graph:040000,062010,010020,000021,020030,][var_info::041504151524][shapes:[8,20,10,40,],[8,20,20,10,40,],[8,20,20,10,],[8,20,20,10,40,],[8,20,20,10,40,],[8,20,20,40,],][choices:compile_shapes:1,]
/root/.cache/jittor/jt1.3.1/g++10.5.0/py3.8.18/Linux-5.4.0-42xae/AMDEPYC7T8364-x8f_debug/default/jit/_opkey0_broadcast_to_Tx_float32__DIM_5__BCAST_4__JIT_1__JIT_cpu_1__index_t_int32___opkey1____hash_ef35f9063cf2acdf_op.cc
1 828us(6.45%,98.4%) 828us 828us 828us 1.77GB/s 2.36GB/s 4.13GB/s 10.1Git/s
transpose[Tx:float32][DIM=4][AXES0=0][AXES2=1][AXES3=2][AXES1=3][JIT:1][JIT_cpu:1][index_t:int32]
/root/.cache/jittor/jt1.3.1/g++10.5.0/py3.8.18/Linux-5.4.0-42xae/AMDEPYC7T8364-x8f_debug/default/jit/transpose_Tx_float32__DIM_4__AXES0_0__AXES2_1__AXES3_2__AXES1_3__JIT_1__JIT_cpu_1__index_t_int32__hash_998b34c8052fe15_op.cc
1 208us(1.62%,100%) 208us 208us 208us 2.35GB/s 2.35GB/s 4.71GB/s 632Mit/s
最终我检查他的输出, 发现是分成了三个部分,
_opkey0_broadcast_to_Tx_float32__DIM_7__BCAST_d__JIT_1__JIT_cpu_1__index_t_int32___opkey1____hash_a2d65b1fd1c3f3d0_op
为卷积实现,
_opkey0_broadcast_to_Tx_float32__DIM_5__BCAST_4__JIT_1__JIT_cpu_1__index_t_int32___opkey1____hash_ef35f9063cf2acdf_op
为矩阵乘,
transpose_Tx_float32__DIM_4__AXES0_0__AXES2_1__AXES3_2__AXES1_3__JIT_1__JIT_cpu_1__index_t_int32__hash_998b34c8052fe15_op
为转置.
2. Halide
2.1 DSL语法
import halide as hl
inputLhs = hl.ImageParam(hl.Float(32), 2, "inputLhs")
inputRhs = hl.ImageParam(hl.Float(32), 2, "inputRhs")
output = hl.Func("output")
(m, n) = hl.Var("m"), hl.Var("n")
k = hl.RDom([hl.Range(0, self.K)], "k")
output[n, m] = 0.0
output[n, m] += inputLhs[k.x, m] * inputRhs[n, k.x]
Halide
使用Var
来表示循环,对于规约的循环需要用RDom
来标识(并且如果定义了规约循环,那么还需要为数据设定初值).
循环层级也是由Var
来确定,
他这里默认应该都会把规约的循环放到最内层.
使用Var
对张量inputLhs[k.x, m]
进行索引操作用于建立access relation
.
提前声明的循环变量的缺点在于需要开发者手动管理好所有的循环变量,
书写起来较为复杂; 优点在于可以确定上下游操作循环之间的关系,
可以轻易的做到自动fusion
上下两层算子.
2.2 测试例子
import halide as hl
input = hl.ImageParam(hl.Float(32), 4, "input")
weight = hl.ImageParam(hl.Float(32), 4, "weight")
act = hl.ImageParam(hl.Float(32), 2, "act")
pad_w_before = 0 # hl.Param(hl.Int(32), "pad_w_before")
pad_h_before = 0 # hl.Param(hl.Int(32), "pad_h_before")
stride_w = 1 # hl.Param(hl.Int(32), "stride_w")
stride_h = 1 # hl.Param(hl.Int(32), "stride_h")
WO, HO, CI, B, CO = hl.Var("WO"), hl.Var("HO"), hl.Var("CI"), hl.Var("B"), hl.Var("CO")
Padding, Paded, Conv, Acted, Clamped, Psumed = hl.Func("Padding"), hl.Func(
"Paded"), hl.Func("Conv"), hl.Func("Acted"), hl.Func("Clamped"), hl.Func("Psumed")
r = hl.RDom([hl.Range(0, weight.width()), hl.Range(0, weight.height()),
hl.Range(0, weight.dim(2).extent())]) # w,h,ic
Padding = hl.BoundaryConditions.constant_exterior(
input, 0, [hl.Range(0, input.width()), hl.Range(0, input.height())])
in_channels = input.dim(2).extent()
out_channels = weight.dim(3).extent()
Paded[WO, HO, CI, B] = Padding[WO - pad_w_before, HO - pad_h_before, CI, B]
Conv[WO, HO, CO, B] = 0.0
Conv[WO, HO, CO, B] += weight[r[0], r[1], r[2], CO] * Paded[WO * stride_w + r[0], HO * stride_h + r[1], r[2], B] # use float to sum
Acted[WO, HO, CO, B] = hl.select(
Conv[WO, HO, CO, B] < act[0, CO],
Conv[WO, HO, CO, B] * act[1, CO] + act[2, CO],
Conv[WO, HO, CO, B] * act[3, CO] + act[4, CO]) # float
Transpose = hl.Func("Transpose")
Transpose[CO, WO, HO, B] = Acted[WO, HO, CO, B]
rhs = hl.ImageParam(hl.Float(32), 4, "rhs") # [x,x,K,N]
N = hl.Var("N")
kdom = hl.RDom([hl.Range(0, rhs.dim(2).extent())], "k")
Matmul = hl.Func("Matmul")
Matmul[N, WO, HO, B] = 0.0
Matmul[N, WO, HO, B] += Transpose[kdom.x, WO, HO, B] * rhs[N, kdom.x, HO, B]
Matmul.print_loop_nest()
得到的循环嵌套如下:
produce Matmul:
for B:
for HO:
for WO:
for N:
Matmul(...) = ...
for B:
for HO:
for WO:
for N:
for k:
produce Conv:
Conv(...) = ...
for r14:
for r14:
for r14:
Conv(...) = ...
consume Conv:
Matmul(...) = ...
矩阵层的初始化他默认放到root
层级,
下面是自动把Transpose
的操作inline
了,
也自动把矩阵乘和卷积进行了fusion
.
3. TVM
TVM
中脱胎于Halide
,
他提供了一套Tensor Expression
的DSL
来协助我们定义算子计算逻辑.
3.1 DSL语法
n = te.var("n")
A = te.placeholder((n,), name="A")
B = te.placeholder((n,), name="B")
C = te.compute(A.shape, lambda i: A[i] + B[i], name="C")
也是使用shape
来表示完美循环,
fcompute
的回调函数的参数映射迭代变量,
同时也会在最内层循环执行它. 同Jittor
类似,
不过使用回调函数的方式更增加了灵活性. 可以使用reduce_axis
,
类似于RDom
, 会自动最内层循环加上规约的循环,
他这里默认的初始化会放到规约循环外面.
3.2 测试例子
import tvm
from tvm import te
from tvm import tir
batch_size = 8
in_channel = 3
out_channel = 16
in_height = 32
in_width = 32
kernel_height = 3
kernel_width = 3
N = 64
Input = te.placeholder(
(batch_size, in_channel, in_height, in_width), name='Input')
Kernel = te.placeholder(
(out_channel, in_channel, kernel_height, kernel_width), name='Kernel')
rc = te.reduce_axis((0, in_channel), name='rc')
ry = te.reduce_axis((0, kernel_height), name='ry')
rx = te.reduce_axis((0, kernel_width), name='rx')
Conv = te.compute(
(batch_size, out_channel, in_height -
kernel_height + 1, in_width - kernel_width + 1),
lambda n, f, y, x: te.sum(
Input[n, rc, y + ry, x + rx] * Kernel[f, rc, ry, rx],
axis=[rc, ry, rx]
),
name='Conv'
) # (b,oc,oh,ow) -> (b,oh,ow,oc)
oh, ow = 30, 30
rhs = te.placeholder((batch_size, oh, out_channel, N), name='rhs')
Trans = te.compute(
(batch_size, oh, ow, out_channel),
lambda i0, i1, i2, i3: Conv[i0, i3, i1, i2])
rk = te.reduce_axis((0, out_channel), name='rk')
MatMul = te.compute(
(batch_size, oh, ow, N),
lambda i0, i1, i2, i3: te.sum(
Trans[i0, i1, i2, rk] * rhs[i0, i1, rk, i3], axis=[rk]),
name='MatMul'
)
s: te.Schedule = te.create_schedule([Conv.op, MatMul.op])
ir = tvm.lower(s, [Input, Kernel, rhs])
ir.show()
@I.ir_module
class Module:
@T.prim_func
def main(Input: T.Buffer((8, 3, 32, 32), "float32"), Kernel: T.Buffer((16, 3, 3, 3), "float32"), rhs: T.Buffer((8, 30, 16, 64), "float32")):
T.func_attr({"from_legacy_te_schedule": T.bool(True), "tir.noalias": T.bool(True)})
Conv = T.allocate([460800], "float32", "global")
compute = T.allocate([115200], "float32", "global")
Conv_1 = T.Buffer((115200,), data=Conv)
for n, f, y, x in T.grid(8, 16, 30, 30):
Conv_1[n * 14400 + f * 900 + y * 30 + x] = T.float32(0)
for rc, ry, rx in T.grid(3, 3, 3):
cse_var_1: T.int32 = n * 14400 + f * 900 + y * 30 + x
Input_1 = T.Buffer((24576,), data=Input.data)
Kernel_1 = T.Buffer((432,), data=Kernel.data)
Conv_1[cse_var_1] = Conv_1[cse_var_1] + Input_1[n * 3072 + rc * 1024 + y * 32 + ry * 32 + x + rx] * Kernel_1[f * 27 + rc * 9 + ry * 3 + rx]
compute_1 = T.Buffer((115200,), data=compute)
for i0, i1, i2, i3 in T.grid(8, 30, 30, 16):
cse_var_2: T.int32 = i0 * 14400
compute_1[cse_var_2 + i1 * 480 + i2 * 16 + i3] = Conv_1[cse_var_2 + i3 * 900 + i1 * 30 + i2]
for i0, i1, i2, i3 in T.grid(8, 30, 30, 64):
Conv_2 = T.Buffer((460800,), data=Conv)
Conv_2[i0 * 57600 + i1 * 1920 + i2 * 64 + i3] = T.float32(0)
for rk in range(16):
cse_var_3: T.int32 = i0 * 57600 + i1 * 1920 + i2 * 64 + i3
rhs_1 = T.Buffer((245760,), data=rhs.data)
Conv_2[cse_var_3] = Conv_2[cse_var_3] + compute_1[i0 * 14400 + i1 * 480 + i2 * 16 + rk] * rhs_1[i0 * 30720 + i1 * 1024 + rk * 64 + i3]
他这里不像Halide
一样需要提前定义好循环变量,
但可以从输出中获取axis
然后使用类似Halide
的调度,
也可以在lower
到tir
之后使用基于tensor ir
的调度.
这里进行lower
依据默认的优化流程后,
并无法自动fusion
.
4. Mlir
Mlir
基于linalg
dialect中的linalg.generic
op提供了一套OpDSL
.
4.1 DSL语法
@linalg_structured_op
def conv_2d_nhwc_hwcf(
I=TensorDef(T1, S.N, S.OH * S.SH + S.KH * S.DH, S.OW * S.SW + S.KW * S.DW, S.C),
K=TensorDef(T2, S.KH, S.KW, S.C, S.F),
O=TensorDef(U, S.N, S.OH, S.OW, S.F, output=True),
strides=IndexAttrDef(S.SH, S.SW, default=[1, 1]),
dilations=IndexAttrDef(S.DH, S.DW, default=[1, 1]),
):
"""Performs 2-D convolution.
Layout:
* Input: NHWC.
* Kernel: HWCF.
Numeric casting is performed on the operands to the inner multiply, promoting
them to the same data type as the accumulator/output.
"""
implements(ConvolutionOpInterface)
domain(D.n, D.oh, D.ow, D.f, D.kh, D.kw, D.c)
O[D.n, D.oh, D.ow, D.f] += TypeFn.cast_signed(
U, I[D.n, D.oh * S.SH + D.kh * S.DH, D.ow * S.SW + D.kw * S.DW, D.c]
) * TypeFn.cast_signed(U, K[D.kh, D.kw, D.c, D.f])
Mlir
这里不使用shape
,
使用和polyhedral
更加贴近的称呼domain
来表示嵌套循环.
我觉得这里更加激进的一点就是完全抛弃循环中的初始化,
也就是忠实的翻译这个OpDSL
所描述的内容.
4.2 测试例子
from mlir.dialects import arith, builtin, func, linalg, tensor, memref
from mlir.dialects.linalg.opdsl.lang import *
from mlir.ir import *
@linalg_structured_op
def transpose_nchw_nhwc(
I=TensorDef(TV.T1, S.d0, S.d1, S.d2, S.d3),
O=TensorDef(TV.T1, S.d0, S.d2, S.d3, S.d1, output=True)
):
domain(D.d0, D.d1, D.d2, D.d3)
implements(ContractionOpInterface)
O[D.d0, D.d2, D.d3, D.d1] = I[D.d0, D.d1, D.d2, D.d3]
@linalg_structured_op
def matmul_4d(
A=TensorDef(TV.T1, S.d0, S.d1, S.M, S.K),
B=TensorDef(TV.T1, S.d0, S.d1, S.K, S.N),
C=TensorDef(TV.T1, S.d0, S.d2, S.M, S.N, output=True)
):
domain(D.d0, D.d1, D.m, D.n, D.k)
implements(ContractionOpInterface)
C[D.d0, D.d1, D.m, D.n] += A[D.d0, D.d1, D.m, D.k] * B[D.d0, D.d1, D.k, D.n]
def testOpResultFromOtherOp():
with Context(), Location.unknown():
module = Module.create()
f32 = F32Type.get()
index_type = IndexType.get()
with InsertionPoint(module.body):
batch_size = 8
in_channel = 3
out_channel = 16
in_height = 32
out_height = 30
in_width = 32
out_width = 30
kernel_height = 3
kernel_width = 3
N = 64
@func.FuncOp.from_py_func(
MemRefType.get(
(batch_size, in_channel, in_height, in_width), f32),
MemRefType.get(
(out_channel, in_channel, kernel_height, kernel_width), f32),
MemRefType.get((batch_size, out_height, out_channel, N), f32),
)
def main(lhs, weight, rhs):
# conv = tensor.EmptyOp([batch_size, out_channel, out_height, out_width], f32)
zero = arith.ConstantOp(F32Type.get(), 0.0)
# CHECK: %[[LHS:.*]] = linalg.fill
conv = memref.AllocOp(MemRefType.get(
[batch_size, out_channel, out_height, out_width], f32), [], [])
linalg.fill(zero, outs=[conv])
linalg.conv_2d_nchw_fchw(lhs, weight, outs=[conv])
trans = memref.AllocOp(MemRefType.get(
[batch_size, out_height, out_width, out_channel], f32), [], [])
transpose_nchw_nhwc(conv, outs=[trans])
matmul = memref.AllocOp(MemRefType.get(
[batch_size, out_height, out_width, N], f32), [], [])
matmul_4d(trans, rhs, outs=[matmul])
return matmul
print(module)
testOpResultFromOtherOp()
得到convmatmul.mlir
:
#map = affine_map<(d0, d1, d2, d3) -> (d0, d1, d2, d3)>
#map1 = affine_map<(d0, d1, d2, d3) -> (d0, d2, d3, d1)>
#map2 = affine_map<(d0, d1, d2, d3, d4) -> (d0, d1, d2, d4)>
#map3 = affine_map<(d0, d1, d2, d3, d4) -> (d0, d1, d4, d3)>
#map4 = affine_map<(d0, d1, d2, d3, d4) -> (d0, d1, d2, d3)>
module {
func.func @main(%arg0: memref<8x3x32x32xf32>, %arg1: memref<16x3x3x3xf32>, %arg2: memref<8x30x16x64xf32>) -> memref<8x30x30x64xf32> {
%cst = arith.constant 0.000000e+00 : f32
%alloc = memref.alloc() : memref<8x16x30x30xf32>
linalg.fill ins(%cst : f32) outs(%alloc : memref<8x16x30x30xf32>)
linalg.conv_2d_nchw_fchw ins(%arg0, %arg1 : memref<8x3x32x32xf32>, memref<16x3x3x3xf32>) outs(%alloc : memref<8x16x30x30xf32>)
%alloc_0 = memref.alloc() : memref<8x30x30x16xf32>
linalg.generic {indexing_maps = [#map, #map1], iterator_types = ["parallel", "parallel", "parallel", "parallel"]} ins(%alloc : memref<8x16x30x30xf32>) outs(%alloc_0 : memref<8x30x30x16xf32>) {
^bb0(%in: f32, %out: f32):
linalg.yield %in : f32
}
%alloc_1 = memref.alloc() : memref<8x30x30x64xf32>
linalg.generic {indexing_maps = [#map2, #map3, #map4], iterator_types = ["parallel", "parallel", "parallel", "parallel", "reduction"]} ins(%alloc_0, %arg2 : memref<8x30x30x16xf32>, memref<8x30x16x64xf32>) outs(%alloc_1 : memref<8x30x30x64xf32>) {
^bb0(%in: f32, %in_2: f32, %out: f32):
%0 = arith.mulf %in, %in_2 : f32
%1 = arith.addf %out, %0 : f32
linalg.yield %1 : f32
}
return %alloc_1 : memref<8x30x30x64xf32>
}
}
使用mlir-opt
进行fusion
:
mlir-opt -allow-unregistered-dialect convmatmul.mlir --convert-linalg-to-affine-loops -o convmatmul1.mlir
mlir-opt -allow-unregistered-dialect convmatmul1.mlir -pass-pipeline='builtin.module(func.func(affine-loop-fusion))' -o convmatmul2.mlir
#map = affine_map<(d0, d1) -> (d0 + d1)>
module {
func.func @main(%arg0: memref<8x3x32x32xf32>, %arg1: memref<16x3x3x3xf32>, %arg2: memref<8x30x16x64xf32>) -> memref<8x30x30x64xf32> {
%alloc = memref.alloc() : memref<1x1x1x16xf32>
%alloc_0 = memref.alloc() : memref<1x1x1x1xf32>
%cst = arith.constant 0.000000e+00 : f32
%alloc_1 = memref.alloc() : memref<8x30x30x64xf32>
affine.for %arg3 = 0 to 8 {
affine.for %arg4 = 0 to 30 {
affine.for %arg5 = 0 to 30 {
affine.for %arg6 = 0 to 16 {
affine.store %cst, %alloc_0[0, 0, 0, 0] : memref<1x1x1x1xf32>
affine.for %arg7 = 0 to 3 {
affine.for %arg8 = 0 to 3 {
affine.for %arg9 = 0 to 3 {
%1 = affine.apply #map(%arg4, %arg8)
%2 = affine.apply #map(%arg5, %arg9)
%3 = affine.load %arg0[%arg3, %arg7, %1, %2] : memref<8x3x32x32xf32>
%4 = affine.load %arg1[%arg6, %arg7, %arg8, %arg9] : memref<16x3x3x3xf32>
%5 = affine.load %alloc_0[0, 0, 0, 0] : memref<1x1x1x1xf32>
%6 = arith.mulf %3, %4 : f32
%7 = arith.addf %5, %6 : f32
affine.store %7, %alloc_0[0, 0, 0, 0] : memref<1x1x1x1xf32>
}
}
}
%0 = affine.load %alloc_0[0, 0, 0, 0] : memref<1x1x1x1xf32>
affine.store %0, %alloc[0, 0, 0, %arg6] : memref<1x1x1x16xf32>
}
affine.for %arg6 = 0 to 64 {
affine.for %arg7 = 0 to 16 {
%0 = affine.load %alloc[0, 0, 0, %arg7] : memref<1x1x1x16xf32>
%1 = affine.load %arg2[%arg3, %arg4, %arg7, %arg6] : memref<8x30x16x64xf32>
%2 = affine.load %alloc_1[%arg3, %arg4, %arg5, %arg6] : memref<8x30x30x64xf32>
%3 = arith.mulf %0, %1 : f32
%4 = arith.addf %2, %3 : f32
affine.store %4, %alloc_1[%arg3, %arg4, %arg5, %arg6] : memref<8x30x30x64xf32>
}
}
}
}
}
return %alloc_1 : memref<8x30x30x64xf32>
}
}
经过affine-loop-fusion
之后的ir基本符合我的预期.
5. Tiramisu
5.1 DSL语法
import tiramisu as tm
import shutil
import os
tm.init("matmul")
M = 64
K = 256
N = 128
# Level I: specifies "what" should be computed
A = tm.input("A", ['m', 'k'], [M, K], tm.primitive_t.p_float32)
B = tm.input("B", ['k', 'n'], [K, N], tm.primitive_t.p_float32)
m, k, n = tm.var("m", 0, M), tm.var("k", 0, K), tm.var("n", 0, N)
C_init = tm.computation("C_init", [m, n], tm.expr(0.0))
C = tm.computation("C", [m, n, k], tm.primitive_t.p_float32)
C.set_expression(C[m, n, k - 1] + A[m, k] * B[k, n])
# Level II: level specifies "when" and "where"
# schedule the computation oerder
# Level III: level specifies "stored"
bufA = tm.buffer("bufA", [M, K], tm.primitive_t.p_float32, tm.argument_t.a_input)
bufB = tm.buffer("bufB", [K, N], tm.primitive_t.p_float32, tm.argument_t.a_input)
bufC = tm.buffer("bufC", [M, N], tm.primitive_t.p_float32, tm.argument_t.a_output)
A.store_in(bufA)
B.store_in(bufB)
C_init.store_in(bufC, [m, n])
C.store_in(bufC, [m, n])
f = tm.get_implicit_function()
f.codegen([bufA, bufB, bufC], "matmul.o", 0, False)
f.dump_halide_stmt()
tiramisu
其实是使用更加贴近于polyhedral
的思想,
computation
可以等价于statement
.
类似halide
一样使用var
表示循环,
用于指定当前computation
的循环位置.
不过他这里内部都是基于polyhedral
,
定义computation
后直接得到了iteration domain
,
经过loop dimension align
可以得到schedule
.
5.2 测试例子
import tiramisu as tm
import shutil
import os
# f = tm.function("matmul")
tm.init("convmatmul")
B = 8
IC = 3
OC = 16
IH, OH = 32, 30
IW, OW = 32, 30
KH = 3
KW = 3
N = 64
# Level I: specifies "what" should be computed
lhs = tm.input("lhs", ['B', 'IC', 'IH', 'IW'], [
B, IC, IH, IW], tm.primitive_t.p_float64)
rhs = tm.input("rhs", ['B', 'OH', 'OC', 'N'], [
B, OH, OC, N], tm.primitive_t.p_float64)
kernel = tm.input("kernel", ['OC', 'IC', 'KH', 'KW'], [
OC, IC, KH, KW], tm.primitive_t.p_float64)
b, oc, oh, ow, ic, kh, kw = tm.var('b', 0, B), tm.var('oc', 0, OC), tm.var('oh', 0, OH), tm.var(
'ow', 0, OW), tm.var('ic', 0, IC), tm.var('kh', 0, KH), tm.var('kw', 0, KW)
ConvInit = tm.computation("conv_init", [b, oc, oh, ow], tm.expr(0.0))
Conv = tm.computation(
"Conv", [b, oc, oh, ow, ic, kh, kw], tm.primitive_t.p_float64)
Conv.set_expression(Conv[b, oc, oh, ow, ic, kh, kw] +
lhs[b, oc, oh + kh, ow + kw] * kernel[oc, ic, kh, kw])
n = tm.var('n', 0, N)
Transpose = tm.computation("transpose", [b, oh, ow, oc], ConvInit[b, oc, oh, ow])
MatmulInit = tm.computation("matmul_init", [b, oh, ow, n], tm.expr(0.0))
Matmul = tm.computation("Matmul", [b, oh, ow, n], tm.primitive_t.p_float64)
Matmul.set_expression(
MatmulInit[b, oh, ow, n] + Transpose[b, oh, ow, oc] * rhs[b, oh, oc, n])
# Level II: level specifies "when" and "where"
# Level III: level specifies "stored"
buflhs = tm.buffer("buflhs", [B, IC, IH, IW], tm.primitive_t.p_float64, tm.argument_t.a_input)
bufrhs = tm.buffer("bufrhs", [B, OH, OC, N], tm.primitive_t.p_float64, tm.argument_t.a_input)
bufkernel = tm.buffer("bufkernel", [OC, IC, KH, KW], tm.primitive_t.p_float64, tm.argument_t.a_input)
bufconv = tm.buffer("bufconv", [B, OC, OH, OW], tm.primitive_t.p_float64, tm.argument_t.a_temporary)
bufmatmul = tm.buffer("bufmatmul", [B, OH, OW, N], tm.primitive_t.p_float64, tm.argument_t.a_output)
lhs.store_in(buflhs)
rhs.store_in(bufrhs)
kernel.store_in(bufkernel)
ConvInit.store_in(bufconv, [b, oc, oh, ow])
Conv.store_in(bufconv, [b, oc, oh, ow])
Transpose.store_in(bufconv, [b, oh, ow, oc])
MatmulInit.store_in(bufmatmul, [b, oh, ow, n])
Matmul.store_in(bufmatmul, [b, oh, ow, n])
f = tm.get_implicit_function()
f.codegen([buflhs, bufrhs, bufkernel, bufconv, bufmatmul], "matmul.o", 0, False)
f.dump_halide_stmt()
produce {
allocate _transpose_b5[float64 * (16 - 0) * (30 - 0) * (30 - 0) * (8 - 0)] in Heap
allocate _rhs_b1[float64 * (64 - 0) * (16 - 0) * (30 - 0) * (8 - 0)] in Heap
allocate _matmul_init_b6[float64 * (64 - 0) * (30 - 0) * (30 - 0) * (8 - 0)] in Heap
allocate _lhs_b0[float64 * (32 - 0) * (32 - 0) * (3 - 0) * (8 - 0)] in Heap
allocate _kernel_b2[float64 * (3 - 0) * (3 - 0) * (3 - 0) * (16 - 0)] in Heap
allocate _conv_init_b3[float64 * (30 - 0) * (30 - 0) * (16 - 0) * (8 - 0)] in Heap
allocate _Matmul_b7[float64 * (64 - 0) * (30 - 0) * (30 - 0) * (8 - 0)] in Heap
allocate _Conv_b4[float64 * (3 - 0) * (3 - 0) * (3 - 0) * (30 - 0) * (30 - 0) * (16 - 0) * (8 - 0)] in Heap
for (c1, 0, 8 - 0) {
for (c3, 0, 30 - 0) {
for (c5, 0, 30 - 0) {
for (c7, 0, 64 - 0) {
if (c3 >= 16) {
bufmatmul[(((0 + (c7*1)) + (c5*64)) + (c3*1920)) + (c1*57600)] = 0.000000
bufmatmul[(((0 + (c7*1)) + (c5*64)) + (c3*1920)) + (c1*57600)] = (float64)bufmatmul[(((0 + (c7*1)) + (c5*64)) + (c3*1920)) + (c1*57600)] + ((float64)bufconv[(((0 + (oc*1)) + (c5*30)) + (c3*900)) + (c1*14400)]*(float64)bufrhs[(((0 + (c7*1)) + (oc*64)) + (c3*1024)) + (c1*30720)])
if (c7 <= 15) {
bufconv[(((0 + (c7*1)) + (c5*30)) + (c3*900)) + (c1*14400)] = (float64)bufconv[(((0 + (c5*1)) + (c3*30)) + (c7*900)) + (c1*14400)]
}
} else if (c7 >= 30) {
bufmatmul[(((0 + (c7*1)) + (c5*64)) + (c3*1920)) + (c1*57600)] = 0.000000
bufmatmul[(((0 + (c7*1)) + (c5*64)) + (c3*1920)) + (c1*57600)] = (float64)bufmatmul[(((0 + (c7*1)) + (c5*64)) + (c3*1920)) + (c1*57600)] + ((float64)bufconv[(((0 + (oc*1)) + (c5*30)) + (c3*900)) + (c1*14400)]*(float64)bufrhs[(((0 + (c7*1)) + (oc*64)) + (c3*1024)) + (c1*30720)])
} else {
for (c9, 0, 3 - 0) {
for (c11, 0, 3 - 0) {
for (c13, 0, 3 - 0) {
if (((c9 == 0) && (c11 == 0)) && (c13 == 0)) {
bufmatmul[(((0 + (c7*1)) + (c5*64)) + (c3*1920)) + (c1*57600)] = 0.000000
}
bufconv[(((0 + (c7*1)) + (c5*30)) + (c3*900)) + (c1*14400)] = (float64)bufconv[(((0 + (c7*1)) + (c5*30)) + (c3*900)) + (c1*14400)] + ((float64)buflhs[(((0 + ((c7 + c13)*1)) + ((c5 + c11)*32)) + (c3*1024)) + (c1*3072)]*(float64)bufkernel[(((0 + (c13*1)) + (c11*3)) + (c9*9)) + (c3*27)])
if (((c9 == 0) && (c11 == 0)) && (c13 == 0)) {
bufmatmul[(((0 + (c7*1)) + (c5*64)) + (c3*1920)) + (c1*57600)] = (float64)bufmatmul[(((0 + (c7*1)) + (c5*64)) + (c3*1920)) + (c1*57600)] + ((float64)bufconv[(((0 + (oc*1)) + (c5*30)) + (c3*900)) + (c1*14400)]*(float64)bufrhs[(((0 + (c7*1)) + (oc*64)) + (c3*1024)) + (c1*30720)])
if (c7 <= 15) {
bufconv[(((0 + (c7*1)) + (c5*30)) + (c3*900)) + (c1*14400)] = (float64)bufconv[(((0 + (c5*1)) + (c3*30)) + (c7*900)) + (c1*14400)]
}
bufconv[(((0 + (c7*1)) + (c5*30)) + (c3*900)) + (c1*14400)] = 0.000000
}
}
}
}
}
}
}
}
}
}
因为tiramisu
是完全依赖手动调度,
所以这里的fusion
使用的buffer
需要提前手动指定,
对于手工优化算子应该会省事情, 但是集成到编译器并不是很合适.
我认为一个算子的实现取决于compute order
,
tiling
, buffer binding
三部分,
作为编译器或者开发者需要利用尽可能多的信息在这三个design space
进行选择从而优化计算性能.
我们期待Tensor DSL
能带来足够多的信息支持做好这件事.
目前这些DSL
都提供了基本的iteration domain
和access relation
的信息.
但是只有halide
直接记录了循环共享的信息,
mlir
应该是在fusion
的优化中通过affine map
来推导出这个信息的.
在linalg
的基本原理文档中,
mlir
总结了不同编译器实现的经验, 需要提供有灵活调度的能力,
又不能像Polyhedral
那样复杂,
并且还要保持SSA
的形式. 从fusion
pass的优化结果上来看, mlir
所提炼的方案还是比较实用的.
Recommend
About Joyk
Aggregate valuable and interesting links.
Joyk means Joy of geeK