

Exascale supercomputing for the enterprise – your best bet vs quantum and neurom...
source link: https://diginomica.com/exascale-supercomputing-enterprise-vs-quantum-neuromorphic
Go to the source link to view the article. You can view the picture content, updated content and better typesetting reading experience. If the link is broken, please click the button below to view the snapshot at that time.
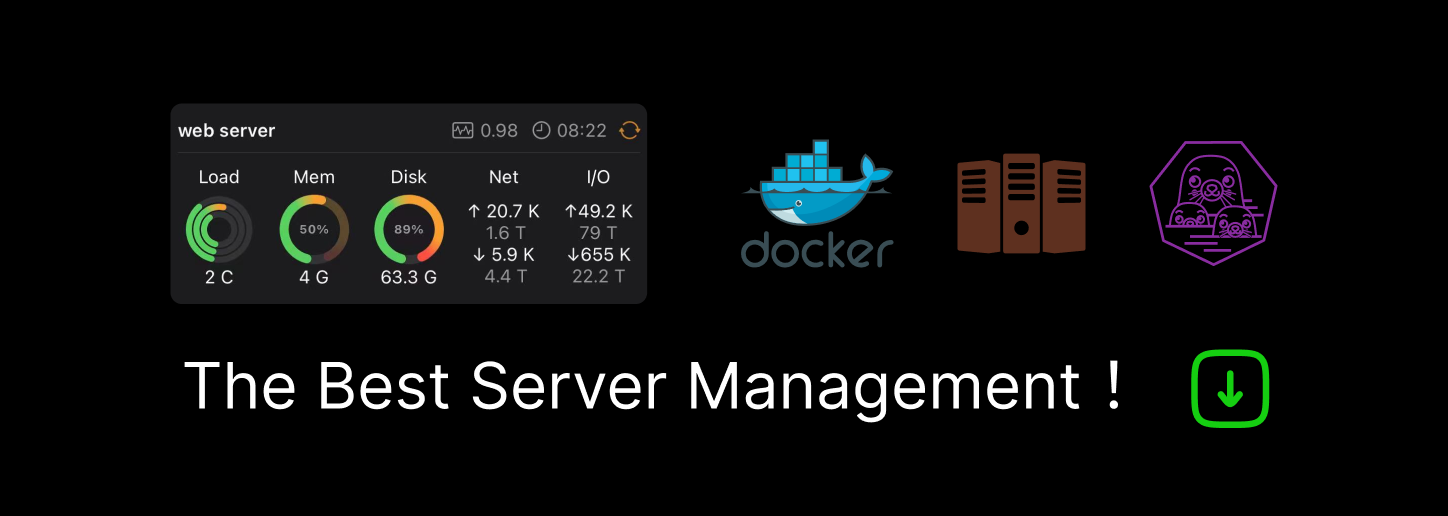
Exascale supercomputing for the enterprise – your best bet vs quantum and neuromorphic rivals

(© casarsaGuru via Canva.com)
While quantum computing emerged as a hot topic in 2023, HPC (High Performance Computing), more colorfully dubbed ‘supercomputing’, broke new ground in two separate developments. First, according to TOP500, an organization that has rated the top 500 supercomputers in the world for twenty-nine years, the first ‘Exascale’ computer is now the fastest in the world. With a new installation now in production. ‘Exascale’ refers to computing systems capable of calculating at least 10^18 IEEE 754 Double Precision (64-bit) operations per second. That’s a quintillion (1,000,000,000,000,000,000) calculations, also known as ‘exaFLOPS’.
In another development, supercomputing has finally broken out of its niche serving scientific research, military and meteorological applications, to find relevance in the enterprise, providing a needed platform for massive Large Language Models (LLM). But traditional supercomputers aren’t the only game in town — new architectures such as quantum and neuromorphic supercomputers hope to overtake their performance, but these for now remain experimental.
Enter the exascale supercomputer
Frontier is the first system with performance exceeding one exaFLOP/second once it passed testing at the Oak Ridge National Laboratory (ORNL) in Tennessee. It achieved 1.194 exaFLOPS/second using 8,699,904 cores. The HPE Cray EX architecture combines 3rd Gen AMD EPYC CPUs optimized for HPC and AI with AMD Instinct 250X accelerators and a Slingshot-11 interconnect.
According to Top500, Frontier is 7x faster and has 4x more information in memory than its predecessors. Nearly 10,000 CPUs perform instructionsfor the computer and are made of integrated circuits — and almost 38,000 GPUs especially useful for grinding out calculations in parallel. Inside Frontier, GPUs do repetitive algebraic math in parallel, which frees the CPUs to direct tasks faster and more efficiently. An article in Scientific American explains some of the applications for this massive computing power:
Frontier is dedicated to fundamental research, which is hoped to illuminate core truths in various fields — such as learning about how energy is produced, how elements are made and how the dark parts of the universe spur its evolution — all through almost-true-to-life simulations in ways that wouldn't have been possible even with the nothing-to-sniff-at supercomputers of a few years ago. In meteorology, it could make hurricane forecasts more precise and manageable. It could experiment with different molecular configurations in chemistry to see which might make great superconductors or pharmaceutical compounds. In medicine, it has already analyzed all the genetic mutations of SARS-CoV-2, the virus that causes COVID — cutting the time that calculation takes from a week to a day — to understand how those tweaks affect the virus's contagiousness. That saved time allows scientists to perform ultrafast iterations, quickly altering their ideas and conducting new digital experiments.
Frontier’s green credentials are also notable, although supercomputing’s carbon footprint still gives some cause for concern. It is ranked as the world’s most energy-efficient supercomputer on the Green500 list, which rates energy use and efficiency by commercially available supercomputing systems, with Frontier delivering 52.592 gigaFLOPS per watt. At full capacity, it nevertheless consumes 22.7MW, which is enough to power a small city for a year. In comparison, though, Aurora, which we’ll come to in a moment and currently #2 on the Top500 list, consumes 24.7MW but only at half of its projected completion in 2024.
Supercomputing for the enterprise
A new system installed in the Microsoft Azure Cloud, called Eagle, has taken the #3 spot, the highest rank a cloud system has ever achieved on the TOP500. We can expect Eagle to be preoccupied with training with LLMs and other AI applications at scale. Only two years ago, a previous Azure system was the first cloud system ever to enter the TOP10 at spot #10. This Microsoft NDv5 system has an HPL score of 561.2 petaFLOPS/s (roughly 0.5 exaFLOPS). It is based on Intel Xeon Platinum 8480C processors and NVIDIA H100 accelerator processors.
In another example of HPC entering the realm of enterprise IT, enterprise technology giant Hewlett Packard Enterprise (HPE) has added an AI cloud option to its GreenLake hybrid cloud portfolio. According to Antonio Neri, the company’s president and CEO:
HPE is making AI, once the domain of well-funded government labs and the global cloud giants, accessible to all by delivering a range of AI applications, starting with large language models, that run on HPE's proven, sustainable supercomputers. Now, organizations can embrace AI to drive innovation, disrupt markets, and achieve breakthroughs with an on-demand cloud service that trains, tunes, and deploys models at scale and responsibly.
The first GreenLake for LLMs will be hosted in the Q01 QScale data center, for which HPE became the anchor tenant in May 2023. This will enable the supercomputer to run on almost 100% renewable energy. The service will also give users access to a pre-trained LLM called Luminous, which Aleph Alpha developed. Luminous is available in multiple languages and can be used with the customer's data to create a customized model.
More exascale contenders
Frontier will soon have better competition (or peers) from incoming exa-machines such as El Capitan, housed at Lawrence Livermore National Laboratory (LLNL), and Aurora, in the #2 spot with a score of 585 petaFLOP/s.
The Aurora system is installed at the Argonne Leadership Computing Facility in Illinois, which is also operated by the Department of Energy (DOE). With roughly half of Frontier's score, it should be noted that it was measured on only half of the system. Once installed, Aurora will roar past Frontier and achieve 2.4 exaFLOPS. This new Intel system is based on HPE Cray EX Intel Exascale Compute Blades and uses Intel Xeon CPU Max Series, Intel Data Center GPU Max Series accelerators, and a Slingshot-11 interconnect.
The US Department of Energy announced a contract with Hewlett Packard Enterprise and AMD to build the El Capitan supercomputer for US$600 million, to be installed at LLNL. It will be used primarily (but not exclusively) for nuclear weapons modeling. El Capitan will be active in early 2024 and will perform two exaFLOPS. It uses AMD and GPUs, with 4 Radeon Instinct GPUs per EPYC Zen 4 CPU, to speed up artificial intelligence tasks. El Capitan should consume around 40MW of electric power. The system will use predictive modeling and simulation to support the nuclear weapons stockpile stewardship program.
Much of the funding for supercomputer research comes from the government. The National Strategic Computing Initiative was created in the US in 2015 by an executive order. The order specified the development of ‘exascale systems’ through funding for research and development of a new class of supercomputers through the National Nuclear Security Administration (NNSA) for placement in national laboratories. The Department of Energy (DOE) oversees these labs and a network of others nationwide. NNSA's role is to manage the nuclear weapons stockpile, relying on exascale computing to maintain the arsenal. Still, part of the original executive order was to ensure supercomputers are applied to solve problems in pure science.
It's not just about FLOPS
With a similar acronym to the LLNL, Los Alamos National Labs (LANL) has long had supercomputing facilities, some of the first. The organization is not focused on exaFLOPS, and its current developments are outside the TOP10. According to LANL, the requirements of simulating stockpile stewardship require a computer cluster that is not only large and fast but prioritizes memory size and speed. The Los Alamos Reporter elaborates in its report, Welcome to Crossroads, LANL's Newest Supercomputer
’Given the hoopla in the press about the “fastest computer in the world,” one might think we should buy computers with the most FLOPS,’ said Gary Grider, the Lab's HPC division leader. ‘Every class of problem requires a different balance of FLOPS, memory size, and memory access. For the problems we are working on, the time it takes to get a result is mainly determined by memory size and memory access, not FLOPS.’ In other words, the application is the thing that dictates the needs of the system. Suppose it's an embarrassingly parallel problem; a flat network of boxes with no exceptional connectivity will suffice. If you're doing fluid dynamics, you would need a highly connected computer and backend storage with loads of memory.
The complexity of some of the Lab's big Multiphysics codes must be balanced, relying heavily on the developers of those codes to help us understand their performance characteristics and identify optimal porting strategies. There still needs to be more certainty about the models used at Exascale. Still, there's certainty about the requirements required: a large number of threads, reduced communication and synchronization, tolerance for and variance in execution time. Even with current programming models, new codes must be written to satisfy these requirements so that future ports don't require algorithm changes. This is particularly important for newly written OpenMP code, as it's natural to write such code without regard to locality and with fine-grain synchronization. Good programming practices are more critical than good programming models.
In another initiative, the Pacific Northwest National Lab (PNNL) will lead a multi-lab exascale project focusing on graph analytics. Researchers to implement new combinatorial algorithms for smart grids, optimizing the grid's response to many events in addition to computational chemistry, computational biology, and climatoloigy.
The world's first human-brain-scale neuromorphic supercomputer
In Australia, a group of scientists have taken a completely different tack, building a machine to emulate the human brain. It’s a neuromorphic contraption that can process the same estimated 228 trillion synaptic operations per second that human brains handle.
It is purported to run in a fraction of the energy of the current class of exascale systems — but still not nearly so efficiently as our brains, which process a billion-billion mathematical operations every second, while consuming only a meagre 20 watts of energy.
And that's why a team at Western Sydney University is building the DeepSouth neuromorphic supercomputer — the first machine ever made that'll be capable of simulating ‘spiking neural networks’at the scale of the human brain. One of the researchers explains it this way:
In a deep neural network, all the hundreds of millions of neurons are activated during each processing step. That uses quite a bit of resources. In a spiking neural network, only a very small subset of neurons are activated at a time. They have an internal state that accumulates some evidence that something might look like a chair, but they don't give out a 'spike' until that evidence reaches a certain threshold.
Simulating spiking neural networks on standard computers using graphics processing units (GPUs) and multicore central processing units (CPUs) must be faster and more power-intensive. The secret sauce uses Intel's research-grade Loihi 2 neuromorphic chip.
DeepSouth is expected to go online in April 2024, and it’s promised to be much smaller and use far less energy than the exascale behemoths described above. And, unlike some other mad science, it doesn’t use any human brain tissue.
My take
I have some doubts about neuromorphic computing. The massive deep-learning neural networks perform many amazing feats, but they are not in any sense intelligent. The flaw is the model isbased on a simplistic and primitive notion of neurons and synapses.The brain is far more complicated than that. Spiking neural networks seems to me to more like a computational optimization rather than a breakthrough in machine intelligence.
As for quantum computing, it is still an open question. IDM’s Osprey computer contains 433 qubits, but is projected to will break the 1000 qubit mark in 2024. Quantum computers face four significant hurdles: 1) error correction, 2) decoherence, 3) cooling, and 4) scalability. However, a recent article in Politico describes some progress on the error correction problem:
Researchers made a potentially major breakthrough in quantum computing last week,nudging the technology ever-so-gradually toward the concrete from the conceptual.
A team of scientists announced a major advancement in the development of ‘error correction’, the process of fighting the subatomic deterioration that makes most quantum computers today unhelpful for more than research purposes. The commercial quantum company QuEra Computing said it achieved a ‘significant leap’, in cooperation with Harvard University, the Massachusetts Institute of Technology and a joint program between the National Institute of Standards and Technology and the University of Maryland.
That’s progress, but there’s still a long way to go before quantum fulfils its promise. Exascale computers are huge, costly and consume tremendous amounts of energy, but for now they are your best bet for training massive foundational models at supercomputing scale.
Recommend
About Joyk
Aggregate valuable and interesting links.
Joyk means Joy of geeK