

使用vLLM和ChatGLM3-6b批量推理 - 扫地升
source link: https://www.cnblogs.com/shengshengwang/p/17923666.html
Go to the source link to view the article. You can view the picture content, updated content and better typesetting reading experience. If the link is broken, please click the button below to view the snapshot at that time.
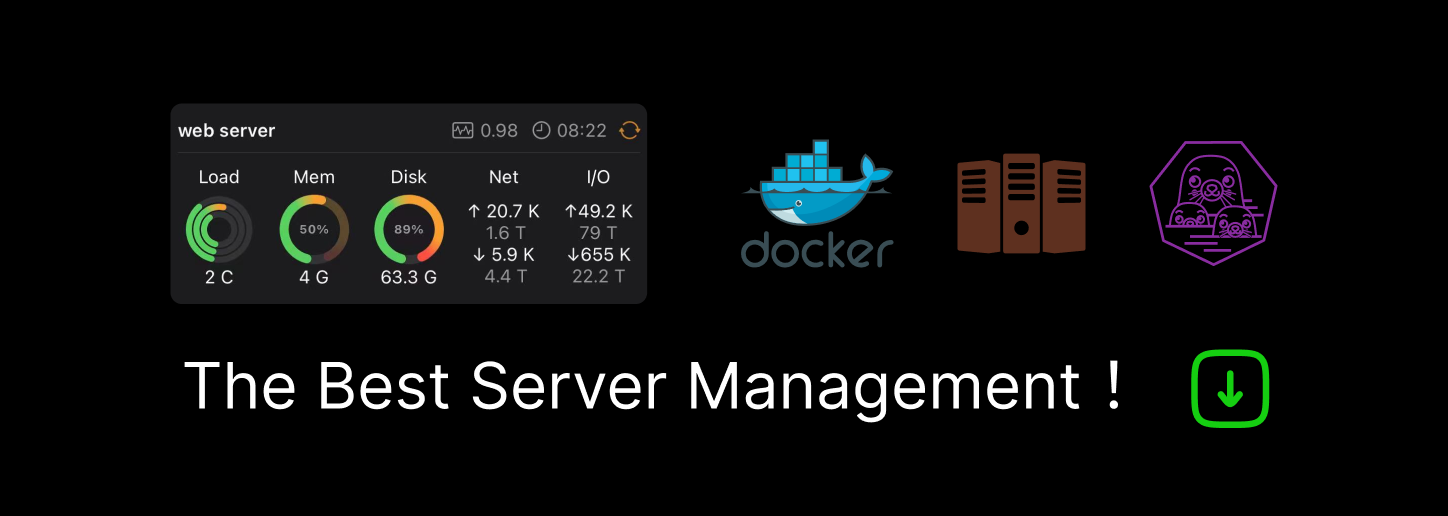
当数据量大的时候,比如百万级别,使用 ChatGLM3-6b 推理的速度是很慢的。发现使用 vLLM 和 ChatGLM3-6b 批量推理极大的提高了推理效率。本文主要通过一个简单的例子进行实践。
1.安装 vLLM 和PyTorch [2]
除了 Python(本文使用 3.11)、CUDA(本文使用 11.8)外,还要安装 vllm、pytorch、xformers 等库,特别注意版本要一致。官方提供类库版本主要是针对 CUDA 12.1 版。如下所示:
# 用CUDA 11.8安装vLLM
# 指定vLLM版本
export VLLM_VERSION=0.2.6
# 指定Python版本
export PYTHON_VERSION=311
# 在线安装whl文件
pip install https://github.com/vllm-project/vllm/releases/download/v${VLLM_VERSION}/vllm-${VLLM_VERSION}+cu118-cp${PYTHON_VERSION}-cp${PYTHON_VERSION}-manylinux1_x86_64.whl
# 用CUDA 11.8重新安装PyTorch
pip uninstall torch -y
pip install torch --upgrade --index-url https://download.pytorch.org/whl/cu118
# 安装xformers库
pip3 install -U xformers --index-url https://download.pytorch.org/whl/cu118
2.离线批量推理例子 [3]
例子实现是比较简单的,主要是参数配置细节,如下所示:
from vllm import LLM, SamplingParams
# 定义批量数据
prompts = [
"宪法规定的公民法律义务有",
"属于专门人民法院的是",
"无效婚姻的种类包括",
"刑事案件定义",
"税收法律制度",
]
sampling_params = SamplingParams(temperature=0.1, top_p=0.5, max_tokens=4096)
path = '/data/ssw/llm_model/chatglm3-6b'
llm = LLM(model=path, trust_remote_code=True, tokenizer_mode="auto", tensor_parallel_size=2, dtype="auto")
outputs = llm.generate(prompts, sampling_params)
# 输出结果
for output in outputs:
prompt = output.prompt
generated_text = output.outputs[0].text
print(f"Prompt: {prompt!r}, Generated text: {generated_text!r}")
(1)temperature=0.1 [5]
控制采样随机性的浮点数。较低的值使模型更具确定性,而较高的值使模型更随机。0 意味着贪婪采样。
(2)top_p=0.5 [5]
控制要考虑的 top tokens 的累积概率的浮点数。必须在(0,1]之间。设置为 1 以考虑所有 token。
(3)max_tokens=4096 [5]
每个输出序列生成的最大 token 数。
(4)trust_remote_code=True [4]
当下载 model 和 tokenizer 时,信任远程代码(比如,来自 HuggingFace)。
(5)tokenizer_mode="auto" [4]
"auto"模式会在可用时使用快速分词器,而"slow"模式则始终使用慢分词器。
(6)tensor_parallel_size=2 [4]
用于张量并行的分布式执行的 GPU 数量。
(7)dtype="auto" [4]
模型权重和激活的数据类型。当前支持 float32,float16 和 bfloat16。如果设置为 auto,将使用模型配置文件中指定的 torch_dtype 属性。然而,如果配置中的 torch_dtype 是 float32,将使用 float16。
[1] https://github.com/vllm-project/vllm/releases
[2] https://docs.vllm.ai/en/latest/
[3] https://github.com/vllm-project/vllm/blob/main/examples/offline_inference.py
[4] https://github.com/vllm-project/vllm/blob/main/vllm/entrypoints/llm.py
[5] https://github.com/vllm-project/vllm/blob/main/vllm/sampling_params.py
[6] 使用vLLM和ChatGLM3-6b批量推理(代码):https://github.com/ai408/nlp-engineering/blob/main/知识工程-大语言模型/公众号文章/使用vLLM和ChatGLM3-6b批量推理/chatglm3_6b_vllm.py
NLP工程化
1.本公众号以对话系统为中心,专注于Python/C++/CUDA、ML/DL/RL和NLP/KG/DS/LLM领域的技术分享。
2.本公众号Roadmap可查看飞书文档:https://z0yrmerhgi8.feishu.cn/wiki/Zpewwe2T2iCQfwkSyMOcgwdInhf
NLP工程化
飞书文档
Recommend
About Joyk
Aggregate valuable and interesting links.
Joyk means Joy of geeK