

Data Modeling for Mere Mortals — Part 3: All We Need Is a Data Lakehouse?!
source link: https://datamozart.medium.com/data-modeling-for-mere-mortals-part-3-all-we-need-is-a-data-lakehouse-1d6cd26bfd67
Go to the source link to view the article. You can view the picture content, updated content and better typesetting reading experience. If the link is broken, please click the button below to view the snapshot at that time.
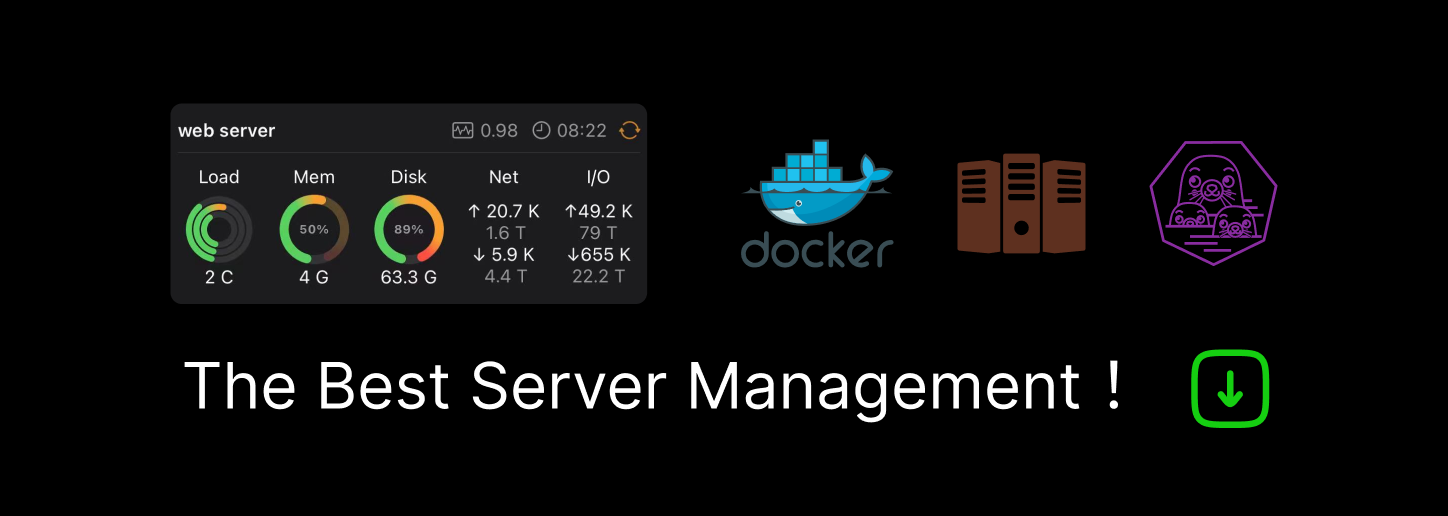
Data Modeling for Mere Mortals — Part 3: All We Need Is a Data Lakehouse?!
Is data lakehouse the concept to rule them all? Do we all need to implement it now? This article will provide you with the answers to all these questions

Image by author
TL; DR: No, data lakehouse is not all we need! But, it’s an extremely important concept to understand, especially in the modern data landscape…
In the previous parts of the Data Modeling for mere mortals series, we examined traditional approaches to data modeling, with a focus on dimensional modeling and Star schema importance for business intelligence scenarios. Now, it’s time to introduce the concept of the modern data platform.
As usual, let’s take a more tool-agnostic approach and learn about some of the key characteristics of the modern data estate. Please, don’t mind if I use some of the latest buzzwords related to this topic, but I promise to reduce their usage as much as possible.
History lessons (again)…
Ok, let’s start with some history lessons and introduce a short history of data architectures.
We’ll kick it off with Data warehouses. Data warehouses, as you’ve already learned in the previous article, have a long history in decision support and business intelligence systems, being around for decades. They represent a mature and well-established architecture for handling huge amounts of structured data. And, this is a typical workflow in traditional enterprise data warehousing architecture:
So, we connect to the structured data stored in various source systems — let’s say transactional databases, then we perform some transformations on this data to shape it in a form that is suitable for analytic workloads — doing things like data cleaning, filtering, and so on — before we load it into a central repository, which is an enterprise data warehouse.
These enterprise data warehouses were, and still are, the focal point of the architecture — a single source of truth for all your organizational data. From there, we are using…
Recommend
About Joyk
Aggregate valuable and interesting links.
Joyk means Joy of geeK