

Don’t build trust with AI, calibrate it
source link: https://uxdesign.cc/dont-build-trust-with-ai-calibrate-it-2889a5740e16
Go to the source link to view the article. You can view the picture content, updated content and better typesetting reading experience. If the link is broken, please click the button below to view the snapshot at that time.
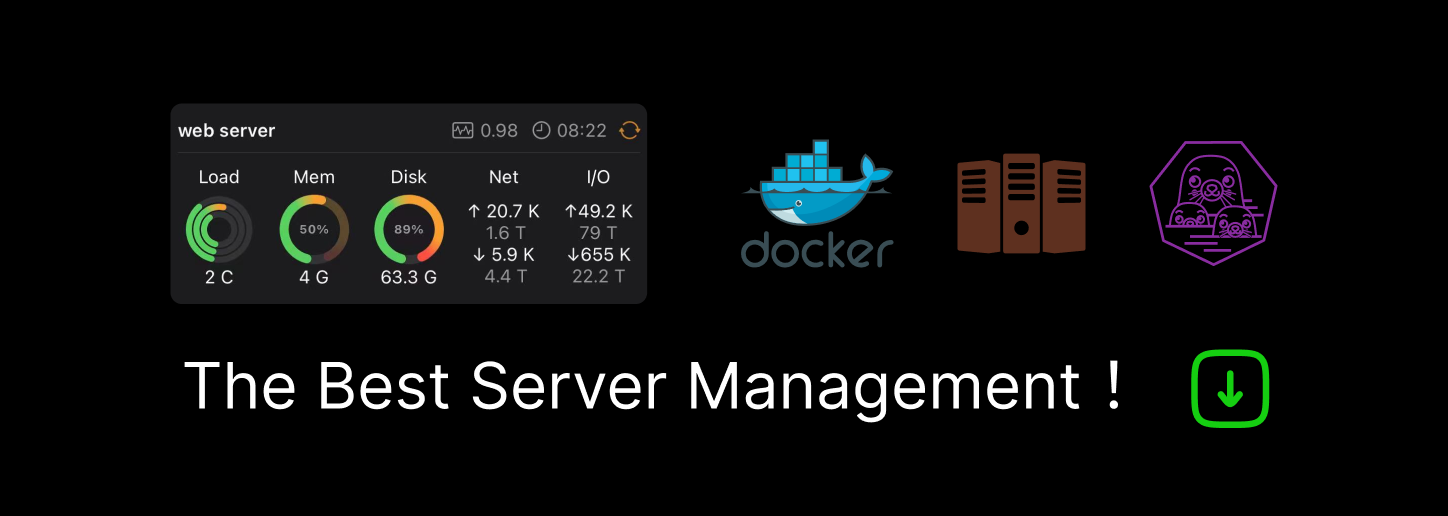
Don’t build trust with AI, calibrate it
Designing AI systems with the right level of trust
AI operates on probabilities and uncertainties. Whether it’s object recognition, price prediction, or a Large Language Model (LLM), AI can make mistakes.
The right amount of trust is a key ingredient in a successful AI-empowered system. Users should trust the AI enough to extract value from the tech, but not so much that they’re blinded to its potential errors.
When designing for AI, we should carefully calibrate trust instead of making users rely on the system blindly.

How people trust AI systems
According to Harvard researchers’ meta-analysis, people often place too much trust in AI systems when presented with final predictions. And just providing explanations for AI predictions doesn’t solve the problem. It serves as a signal for AI competence rather than drawing attention to AI mistakes.
Sure, a few wrong turns won’t ruin your journey if you’re just seeking movie or music recommendations. But in critical decision-making situations, this approach can make users “co-dependent on AI and susceptible to AI mistakes.”
The trust issue becomes even more critical with LLM chatbots. These interactions naturally create more trust and even emotional connection.
Evaluate risk
Every person involved in the creation of AI at any step is responsible for its impact. The first thing that AI designers have to do is to understand the risk of their solution.
How critical is it if AI makes a mistake, and how likely is it to happen?

If the available training data is biased, messy, subjective, or discriminatory it can almost certainly lead to harmful results. The team's first priority, in that case, should be to prevent this.
For example, a recidivism risk assessment algorithm used in some US states is biased against black people. It predicts a score of the likelihood of committing a future crime and often rates black…
Recommend
-
22
Modern applications don’t crash; they hang. One of the main reasons for it is the assumption that the network is reliable. It isn’t. When you make a network call without setting a timeout, you are telling your code...
-
9
Don't Trust the DOM 03 Jun 2014 A long-known maxim is "Don't trust the client". Web browsers, being clients, are no exception. Usually they'll r...
-
10
Don't trust those survey numbers Let's say you have a company with a bunch of employees. Business is "super super awesome", and everyone is really happy with how you are running things. Some magazine comes along and polls a subse...
-
9
New Samsung TVs will calibrate HDR10+ movies in response to ambient light By Henry St Leger 4 hours ago New Samsung TVs get one of LG and Panasonic's best fe...
-
4
How to easily color calibrate your Apple TV with an iPhoneYou don't even need the newest A...
-
4
By:
-
1
Powerful campaign analytics platform for marketersA powerful campaign analytics platform for marketers to level up their analytics. Connecting up to 5 premium marketing platforms (LinkedIn, Facebook, Google Ads, Google Analytics,...
-
3
How to calibrate an Apple Watch for accurate fitness tracking
-
7
Calibrate Used Zoom to Announce Layoffs Then Wiped Laptops US Markets Loading......
-
4
Can't calibrate new XDR MacBook Pro display - missing 'Color' button, calibration option. ...
About Joyk
Aggregate valuable and interesting links.
Joyk means Joy of geeK