

IBM team builds low-power analog AI processor
source link: https://arstechnica.com/science/2023/08/ibm-team-builds-low-power-analog-ai-processor/
Go to the source link to view the article. You can view the picture content, updated content and better typesetting reading experience. If the link is broken, please click the button below to view the snapshot at that time.
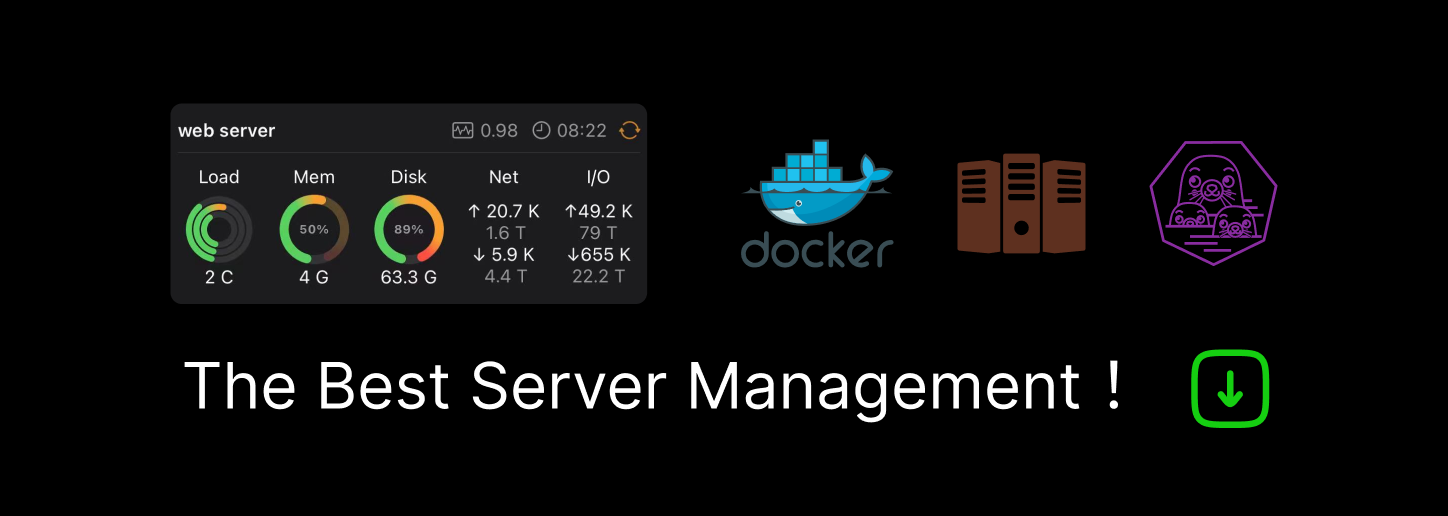
Power down —
IBM team builds low-power analog AI processor
Huge arrays of phase-change material perform in-memory processing.
John Timmer - 8/23/2023, 7:23 PM
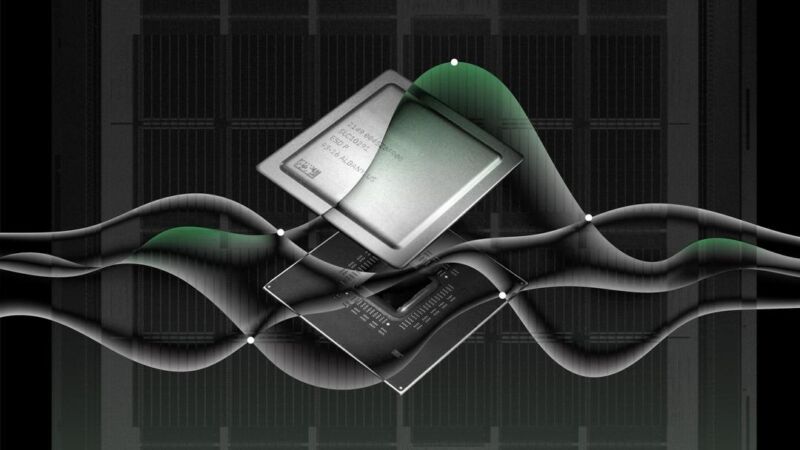
Large language models, the AI tech behind things like Chat GPT, are just what their name implies: big. They often have billions of individual computational nodes and huge numbers of connections among them. All of that means lots of trips back and forth to memory and a whole lot of power use to make that happen. And the problem is likely to get worse.
One way to potentially avoid this is to mix memory and processing. Both IBM and Intel have made chips that equip individual neurons with all the memory they need to perform their functions. An alternative is to perform operations in memory, an approach that has been demonstrated with phase-change memory.
Now, IBM has followed up on its earlier demonstration by building a phase-change chip that's much closer to a functional AI processor. In a paper released on Wednesday by Nature, the company shows that its hardware can perform speech recognition with reasonable accuracy and a much lower energy footprint.
In phase
Phase-change memory has been under development for a while. It offers the persistence of flash memory but with performance that's much closer to existing volatile RAM. It operates by heating a small patch of material and then controlling how quickly it cools. Cool it slowly, and the material forms an orderly crystal that conducts electricity reasonably well. Cool it quickly, and it forms a disordered mess that has much higher resistance. The difference between these two states can store a bit that will remain stored until enough voltage is applied to melt the material again.
AdvertisementThis behavior also turns out to be a great match for neural networks. In neural networks, each node receives an input and, based on its state, determines how much of that signal to forward to further nodes. Typically, this is viewed as representing the strength of the connections between individual neurons in the network. Thanks to the behavior of phase-change memory, that strength can also be represented by an individual bit of memory operating in an analog mode.
When storing digital bits, the difference between the on and off states of phase-change memory is maximized to limit errors. But it's entirely possible to set the resistance of a bit to values anywhere in between its on and off states, allowing analog behavior. This smooth gradient of potential values can be used to represent the strength of connections between nodes—you can get the equivalent of a neural network node's behavior simply by passing current through a bit of phase-change memory.
As mentioned above, IBM has already shown this can work. The chip described today, however, is much closer to a functional processor, containing all the hardware needed to connect individual nodes. And it has done so at a scale much closer to that needed to handle large language models.
Page:
Recommend
About Joyk
Aggregate valuable and interesting links.
Joyk means Joy of geeK