

Expanding Transformer size without losing function or starting from scratch
source link: https://arxiv.org/abs/2308.06103
Go to the source link to view the article. You can view the picture content, updated content and better typesetting reading experience. If the link is broken, please click the button below to view the snapshot at that time.
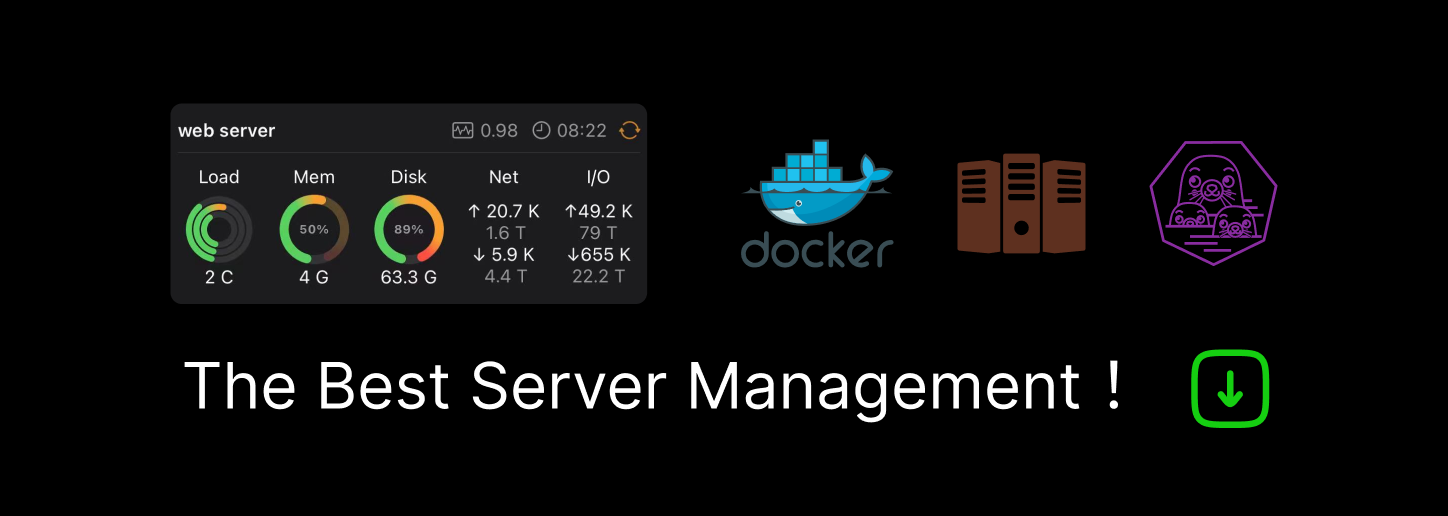
Computer Science > Machine Learning
[Submitted on 11 Aug 2023]
Composable Function-preserving Expansions for Transformer Architectures
Training state-of-the-art neural networks requires a high cost in terms of compute and time. Model scale is recognized to be a critical factor to achieve and improve the state-of-the-art. Increasing the scale of a neural network normally requires restarting from scratch by randomly initializing all the parameters of the model, as this implies a change of architecture's parameters that does not allow for a straightforward transfer of knowledge from smaller size models. In this work, we propose six composable transformations to incrementally increase the size of transformer-based neural networks while preserving functionality, allowing to expand the capacity of the model as needed. We provide proof of exact function preservation under minimal initialization constraints for each transformation. The proposed methods may enable efficient training pipelines for larger and more powerful models by progressively expanding the architecture throughout training.
Recommend
About Joyk
Aggregate valuable and interesting links.
Joyk means Joy of geeK