
14

使用 wechaty langchain 部署私有 chatgpt
source link: https://wechaty.js.org/2023/07/07/wechaty-chat-with-langchain/
Go to the source link to view the article. You can view the picture content, updated content and better typesetting reading experience. If the link is broken, please click the button below to view the snapshot at that time.
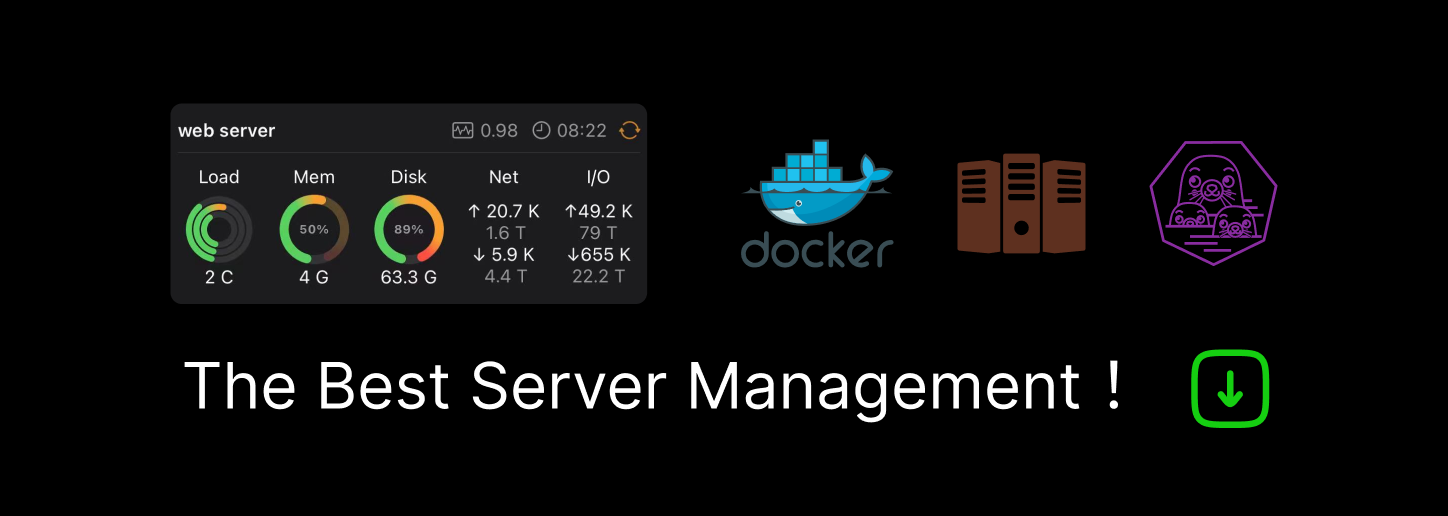
WeChaty 是一个基于 Node.js 的开源微信机器人框架,而 LangChain 是一个用于部署私有化 GPT 模型的工具。通过结合 WeChaty 和 LangChain,你可以创建一个私有化的 GPT 机器人,使其在微信平台上运行。
Setup:
我们使用 wechaty-puppet-wechat4u
package.json:
"wechaty": "^1.20.2",
"wechaty-puppet-wechat4u": "1.14.1"
"langchain": "^0.0.102",
"@pinecone-database/pinecone": "^0.1.6",
"pdf-parse": "^1.1.1", // 篇幅原因这里只演示 pdf
import { WechatyBuilder} from 'wechaty'
const wechaty = WechatyBuilder.build({
name: 'wechaty-chatgpt',
puppet: 'wechaty-puppet-wechat4u',
puppetOptions: {
uos: true,
},
});
设置 pinecone ,openai
PROMPTLAYER_API_KEY=pl_... # PROMPTLAYER 是一个用于记录 api 调用时 prompt 与 response 的工具
PINECONE_API_KEY=89e...
PINECONE_ENVIRONMENT=us-west4-gcp-free
PINECONE_INDEX=...
以下代码为当接收到支持的文件对文件进行向量化成功后返回提示
wechaty.on('message', async message => {
const contact = message.talker();
currentAdminUser = contact.payload.alias === process.env.ADMIN
const receiver = message.listener();
let content = message.text().trim();
const room = message.room();
const target = room || contact;
const isText = message.type() === wechaty.Message.Type.Text;
const isAudio = message.type() === wechaty.Message.Type.Audio;
const isFile = message.type() === wechaty.Message.Type.Attachment;
if (isFile) {
const filebox = await message.toFileBox()
if (supportFileType(filebox.mediaType)) {
await saveFile(filebox)
await loadDocuments()
await send(room || contact, `${filebox.name} Embeddings 成功`)
return
}
}
})
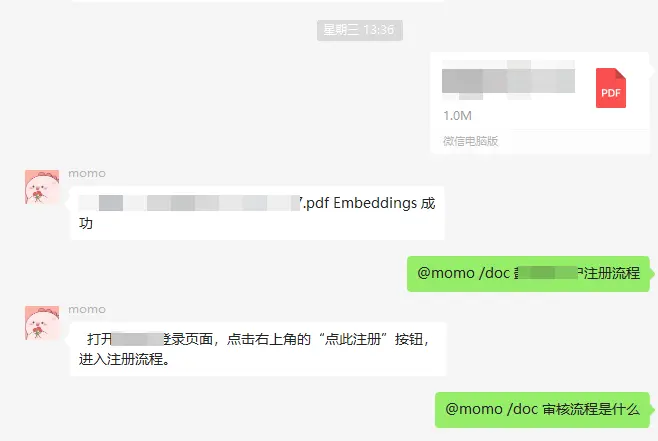
langchain 相关代码
import { PineconeClient } from "@pinecone-database/pinecone";
import dotenv from 'dotenv';
import { VectorDBQAChain } from "langchain/chains";
import { DirectoryLoader } from "langchain/document_loaders";
import { DocxLoader } from "langchain/document_loaders/fs/docx";
import { PDFLoader } from "langchain/document_loaders/fs/pdf";
import { TextLoader } from "langchain/document_loaders/fs/text";
import { OpenAIEmbeddings } from "langchain/embeddings/openai";
import { PromptLayerOpenAI } from "langchain/llms/openai";
import { RecursiveCharacterTextSplitter } from "langchain/text_splitter";
import { PineconeStore } from "langchain/vectorstores";
dotenv.config();
const client = new PineconeClient();
await client.init({
apiKey: process.env.PINECONE_API_KEY,
environment: process.env.PINECONE_ENVIRONMENT,
});
const pineconeIndex = client.Index(process.env.PINECONE_INDEX);
async function loadDocuments(directory = 'resource') {
console.log('loadDocuments...')
const loader = new DirectoryLoader(directory,
{
".pdf": (path) => new PDFLoader(path),
".txt": (path) => new TextLoader(path),
".doc": (path) => new DocxLoader(path),
".docx": (path) => new DocxLoader(path),
});
// 将数据转成 document 对象,每个文件会作为一个 document
const rawDocuments = await loader.load();
console.log(`documents: ${rawDocuments.length}`);
// 初始化加载器
const textSplitter = new RecursiveCharacterTextSplitter({ chunkSize: 500 });
// 切割加载的 document
const splitDocs = await textSplitter.splitDocuments(rawDocuments);
// 持久化数据
// const docsearch = await Chroma.fromDocuments(splitDocs, embeddings, { collectionName: "private_doc" });
// docsearch.persist();
await PineconeStore.fromDocuments(splitDocs, new OpenAIEmbeddings(), {
pineconeIndex,
});
console.log(`send to PineconeStore`);
}
async function askDocument(question) {
const llm = new PromptLayerOpenAI({ plTags: ["langchain-requests", "chatbot"] })
// 初始化 openai 的 embeddings 对象
// 加载数据
const vectorStore = await PineconeStore.fromExistingIndex(
new OpenAIEmbeddings(),
{ pineconeIndex }
);
/* Search the vector DB independently with meta filters */
const chain = VectorDBQAChain.fromLLM(llm, vectorStore, {
k: 1,
returnSourceDocuments: true,
});
const response = await chain.call({ query: question });
console.log(response);
// const response = await vectorStore.similaritySearch(question, 1);
// console.log(response);
return response.text
}
function supportFileType(mediaType) {
const types = ['doc', 'docx', , 'pdf', 'text']
return types.filter(e => mediaType.includes(e)).length > 0
}
export { askDocument, loadDocuments, supportFileType };
Recommend
About Joyk
Aggregate valuable and interesting links.
Joyk means Joy of geeK