

Stop asking UX researchers to defend their sample size
source link: https://uxdesign.cc/stop-asking-ux-researchers-to-defend-their-sample-size-65aa5c2305d2
Go to the source link to view the article. You can view the picture content, updated content and better typesetting reading experience. If the link is broken, please click the button below to view the snapshot at that time.
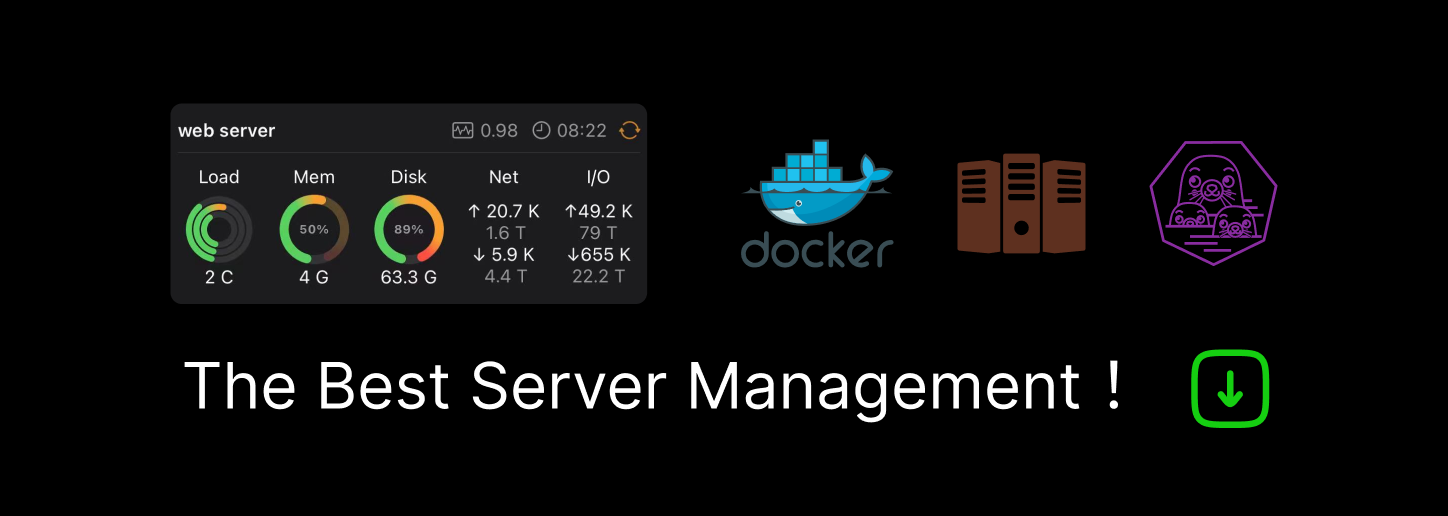
Stop asking UX researchers to defend their sample size
n = 3 reasons to trust your UXR and let them work in peace
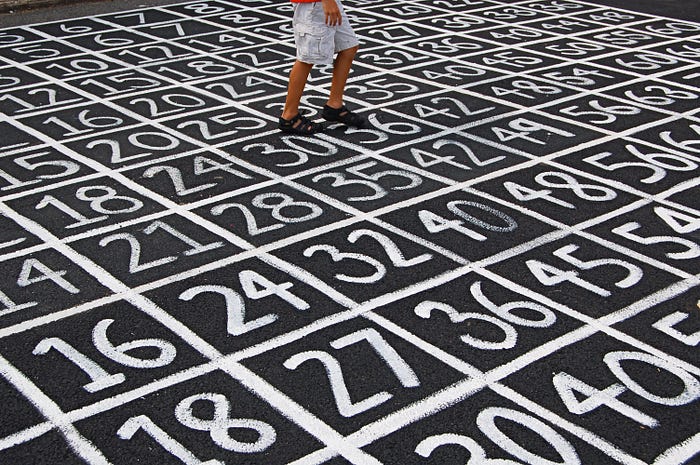
Photo by Markus Krisetya on Unsplash
There’s a lot of things that grind my gears. But one annoyance that I’m certain I share with other UX researchers is being questioned about my sample size.
“You spoke to just X users? Oh.”
“Are you sure surveying Y users is enough to get a representative group?”
Please stop.
If you’re not a researcher, this post will walk through three reasons why your questions and concerns about sample size may sometimes be a little off the mark.
And if you are a researcher, hopefully this will give you some ideas on how to respond next time you’re confronted by The Sample Size Question.
Reason #1: Generalizability is a function not of sample size, but sampling strategy
When people ask for and ask about “representative” samples, usually their underlying concern is over generalizability: To what extent can research findings from a given sample apply to a broader population?
This is a fine and important question to ask, but the important thing to remember is that generalizability doesn’t come from how manypeople are in your sample, but how you selectedthe people to be in your sample.
A really simple thought exercise can illustrate this point well. Imagine that you’ve contracted me to estimate popular support for Prime Minister Justin Trudeau. Which approach would produce a result that you trust more:
(A) 200 people randomly selected from an (imaginary) list of all Canadian adults
(B) 1000 people I found at a mall in downtown Toronto

The Eaton Centre in Toronto — a city which, despite what we Torontonians may think sometimes, is not representative of Canada as a whole politically (Canmenwalker on Wikipedia)
This example is tongue-in-cheek, but it makes my point: If a sampling strategy is flawed having a bigger sample size doesn’t help make the sample more representative.
In a similar vein, the way to make a sample more representative isn’t necessarily to recruit more people but to change or adjust the sampling strategy.
So if your concern is “would the results from this sample still hold for the wider population,” ask your UXR about how they’re recruiting and sampling users, not on whether they think they have enough people or if they can talk to a couple more folks.
Reason #2: Generalizability is not the goal of qualitative research
This point may seem provocative, so let me be blunt: There is no perfect research method. Different methods and approaches each have their strengths and weaknesses. It’s why I love being a mixed-methods researcher.
In the social sciences, the strength of qualitative approaches is in generating deep nuanced understanding of beliefs behind behaviours; in being able to follow and trace processes over time; or in generating leads and ideas when working in a new problem space for the first time. But the cost of that ‘in-depth-ness’ is the inability (or, I suppose, inefficiency) to generate that level of nuance and richness at scale.
As an analogy, consider a telescope. The bigger the aperture of a telescope, the farther you can see with it. At the same time, the bigger the telescope, the narrower its field of view. There’s a direct tradeoff between how wide of the night sky you can see and how far you can see into it, and this is just as true for amateur telescopes (of which I was recently in the market for) as for those used by astrophysicists.
Qualitative methods tend to have big research apertures — you can peer deep and far with it, but that comes at the cost of narrowing your field of view. Quantitative methods lets you look at a wider slice of your population, but as a result you’re not peering as far or as deep into your sample as you could qualitatively.
(One final point to close the loop on this analogy: No one mocks the James Webb Space Telescope for peering deeply into a sliver of spacetime instead of widely surveying a big swath of the night sky — in fact, we celebrate it — so why do we give qualitative researchers a hard time for doing the same?)

The latest gem from NASA’s JWST (https://www.flickr.com/photos/nasawebbtelescope/53003114489/in/album-72177720305127361/)
None of this is to say that qualitative researchers don’t pay attention to generalizability as a general factor or concern. When designing qualitative studies, researchers are thinking about their sampling strategy and whether their resulting sample differs in significant and important ways from the population at large. But there’s a difference between thinking about generalizability and making it the end-all-be-all. If generalizing is the most important goal you have for a study, consider a quantitative approach instead. Just keep in mind the drawbacks.
I’ll repeat: There is no perfect research method.
Reason #3: In qual research, the appropriate sample size is determined by the principle of saturation
So if qualitative sample sizes are not being determined by generalizablity concerns, how do qualitative researchers arrive at a sample size?
Let’s say your UXR is doing an interview study and they begin talking to users. At some point, they’ll reach a stage where they’re largely hearing the same thing from each additional user and are no longer learning new information that’s helpful for answering the research questions. That stage is what UXRs call saturation.
There are two important implications for using the principle of saturation for determining sample sizes.
One: You can’t really know when you’ll have enough data until you start collecting that data. You can make educated guesses of course, but UXRs can and do adjust their data collection as they begin working with and through the collected data. Expect that kind of adjusting-as-we-go from your UXR.
Two: How many users are needed to reach saturation will depend a lot on how many research questions there are; how complicated or nuanced those questions are; how much your team already knows about the problem space; and how many sampling criteria there are for the study, just to name a few.
To be a little less vague by drawing on personal experience: I’ve found the oft-repeated claim of “you only need five users for usability testing” largely true when testing something relatively straightforward and when you really only care about a handful of things. A majority of my usability studies have five to 10 users. Interviews can vary a little more because of the wider variety of interview studies, but most of my own have had sample sizes of seven to 15.
(My record for interviews comes from my time working at a non-profit, where an emancipatory interview study with an unstructured protocol ended up with 27 participants. That was a pretty unique case — I would not recommend trying at home).
Lessons on sample size
So, what did we learn about qualitative sample sizes today?
- Qualitative researchers determine their sample size through the principle of saturation
- If your curiosity about sample size is because you want to generalize findings more broadly, consider asking your UXR to complement a qualitative study with a quant one, or ask them about their sampling strategy (not sample size)
- Qualitative researchers are attentive to concerns about generalizability, but it’s not the goal or the strength of qualitative approaches
Recommend
About Joyk
Aggregate valuable and interesting links.
Joyk means Joy of geeK