

图书搜索领域重大突破!用Apache SeaTunnel、Milvus和OpenAI提高书名相似度搜索精准度...
source link: https://www.cnblogs.com/seatunnel/p/17506521.html
Go to the source link to view the article. You can view the picture content, updated content and better typesetting reading experience. If the link is broken, please click the button below to view the snapshot at that time.
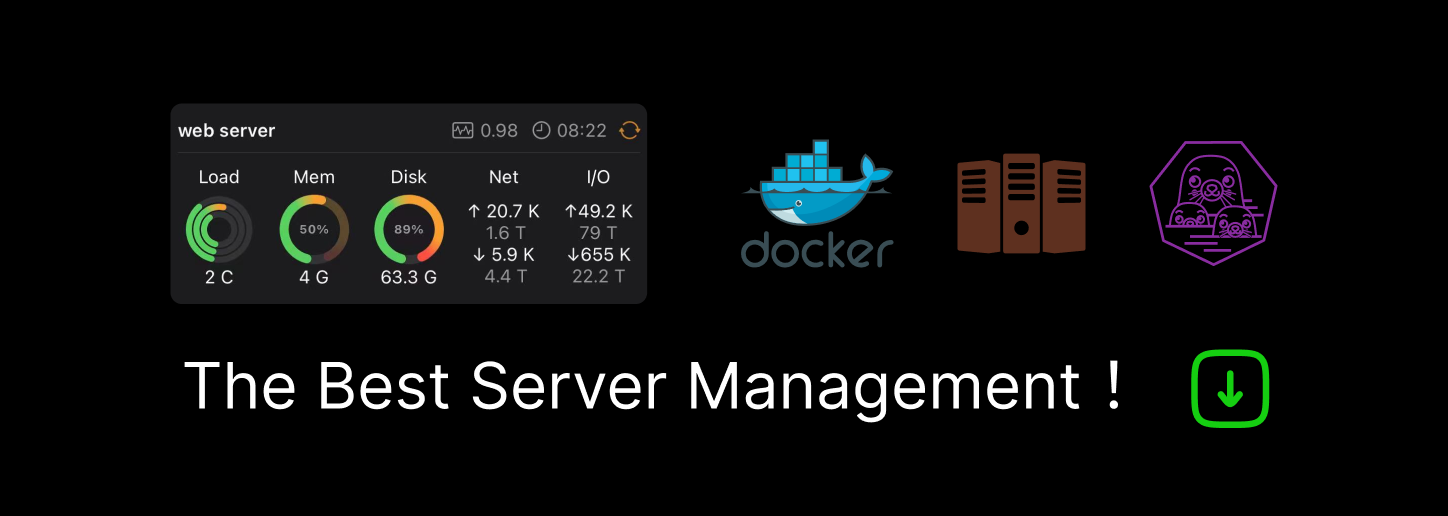
图书搜索领域重大突破!用Apache SeaTunnel、Milvus和OpenAI提高书名相似度搜索精准度和效率
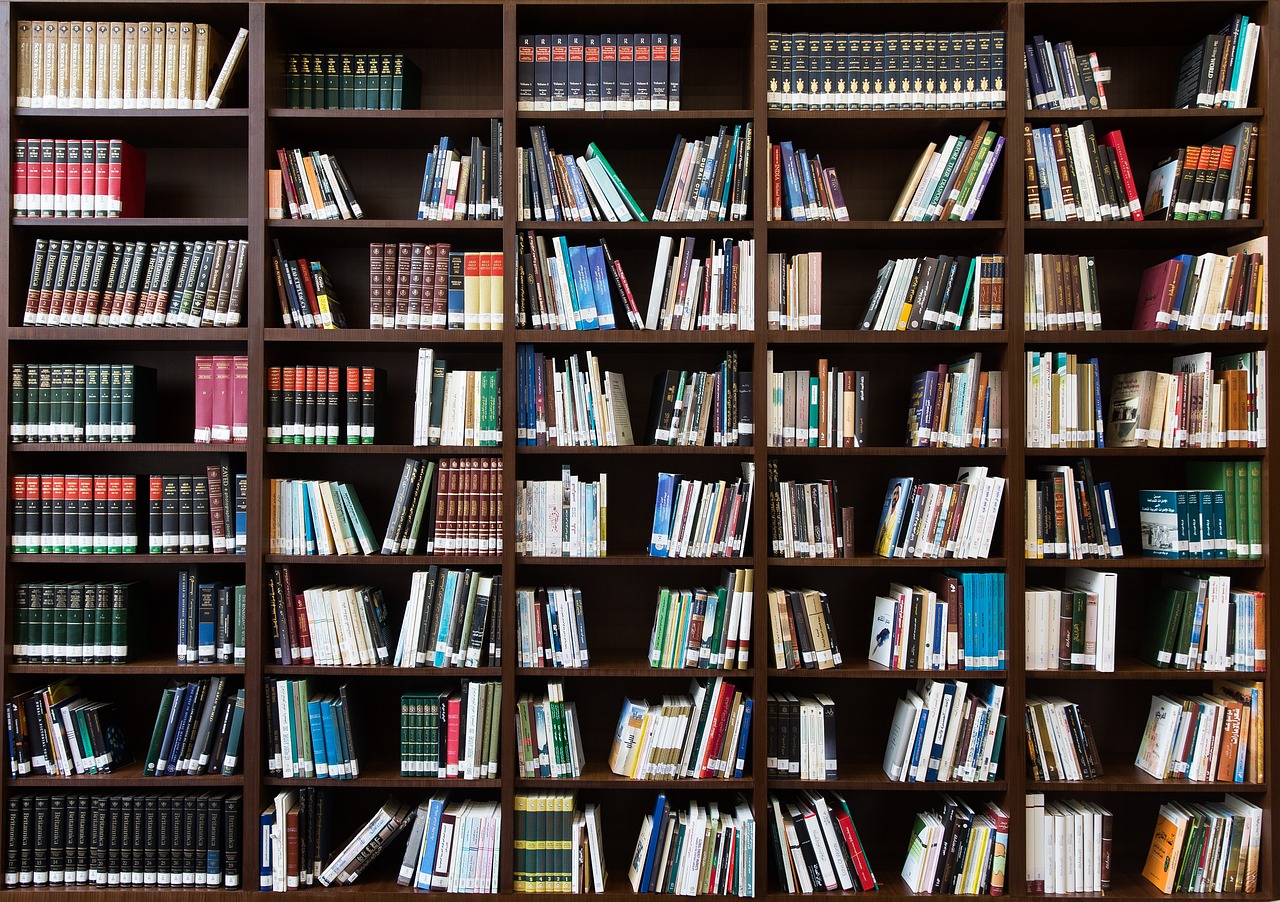
作者 | 刘广东,Apache SeaTunnel Committer
目前,现有的图书搜索解决方案(例如公共图书馆使用的解决方案)十分依赖于关键词匹配,而不是对书名实际内容的语义理解。因此会导致搜索结果并不能很好地满足我们的需求,甚至与我们期待的结果大相径庭。这是因为仅仅依靠关键词匹配是不够的,因为它无法实现语义理解,也就无法理解搜索者真正的意图。
那么,有更好的方法可以让我们更加准确、高效地进行图书搜索吗?答案是有!本文中,我将介绍如何结合使用Apache SeaTunnel、Milvus和OpenAI进行相似度搜索,实现对整个书名的语义理解,从而让搜索结果更加精准。
使用训练有素的模型来表示输入数据被称为语义搜索,这种方法可以扩展到各种不同的基于文本的用例,包括异常检测和文档搜索。因此,本文所介绍的技术可以为图书搜索领域带来重大的突破和影响。
接下来我来简单介绍几个与本文相关的概念和用到的工具/平台,便于大家更好地理解本文。
什么是Apache SeaTunnel
Apache SeaTunnel是一个开源的、高性能的、分布式的数据管理和计算平台。它是由Apache基金会支持的一个顶级项目,能够处理海量数据、提供实时的数据查询和计算,并支持多种数据源和格式。SeaTunnel的目标是提供一个可扩展的、面向企业的数据管理和集成平台,以满足各种大规模数据处理需求。
什么是Milvus
Milvus是一个开源的类似向量搜索引擎,它支持海量向量的存储、检索和相似度搜索,是一个针对大规模向量数据的高性能、低成本的解决方案。Milvus可以在多种场景下使用,如推荐系统、图像搜索、音乐推荐和自然语言处理等。
什么是OpenAI
ChatGPT是一种基于GPT(Generative Pre-trained Transformer)模型的对话生成系统,是由OpenAI开发的。该系统主要使用了自然语言处理、深度学习等技术,可以生成与人类对话相似的自然语言文本。ChatGPT的应用范围很广,可以用于开发智能客服、聊天机器人、智能助手等应用程序,也可以用于语言模型的研究和开发。近年来,ChatGPT已经成为了自然语言处理领域的研究热点之一。
什么是LLM(Large Language Model)
大语言模型(Large Language Model)是一种基于深度学习技术的自然语言处理模型,它可以对一段给定的文本进行分析和理解,并生成与之相关的文本内容。大语言模型通常使用深度神经网络来学习自然语言的语法和语义规则,并将文本数据转换为连续向量空间中的向量表示。在训练过程中,大语言模型利用大量的文本数据来学习语言模式和统计规律,从而可以生成高质量的文本内容,如文章、新闻、对话等。大语言模型的应用领域非常广泛,包括机器翻译、文本生成、问答系统、语音识别等。目前,许多开放源代码深度学习框架都提供了大语言模型的实现,如TensorFlow、PyTorch等。
重点来了!我将展示如何将Apache SeaTunnel、OpenAI的Embedding API与我们的矢量数据库结合使用,来通过语义搜索整个书名。
在实验之前,我们需要去官网获取一个OpenAI的token,然后在去部署一个Milvus的实验环境。我们还需要准备好将用于这个例子的数据。你可以从这里下载数据。
通过SeaTunnel将数据导入Milvus
首先,将book.csv放到/tmp/milvus_test/book下,然后配置任务配置为milvus.conf并放到config下。请参考快速使用指南。
env {
# You can set engine configuration here
execution.parallelism = 1
job.mode = "BATCH"
checkpoint.interval = 5000
#execution.checkpoint.data-uri = "hdfs://localhost:9000/checkpoint"
}
source {
# This is a example source plugin **only for test and demonstrate the feature source plugin**
LocalFile {
schema {
fields {
bookID = string
title_1 = string
title_2 = string
}
}
path = "/tmp/milvus_test/book"
file_format_type = "csv"
}
}
transform {
}
sink {
Milvus {
milvus_host = localhost
milvus_port = 19530
username = root
password = Milvus
collection_name = title_db
openai_engine = text-embedding-ada-002
openai_api_key = sk-xxxx
embeddings_fields = title_2
}
}
执行如下命令:
./bin/SeaTunnel.sh --config ./config/milvus.conf -e local
查看数据库中数据,可以看到已经有数据写入进去。
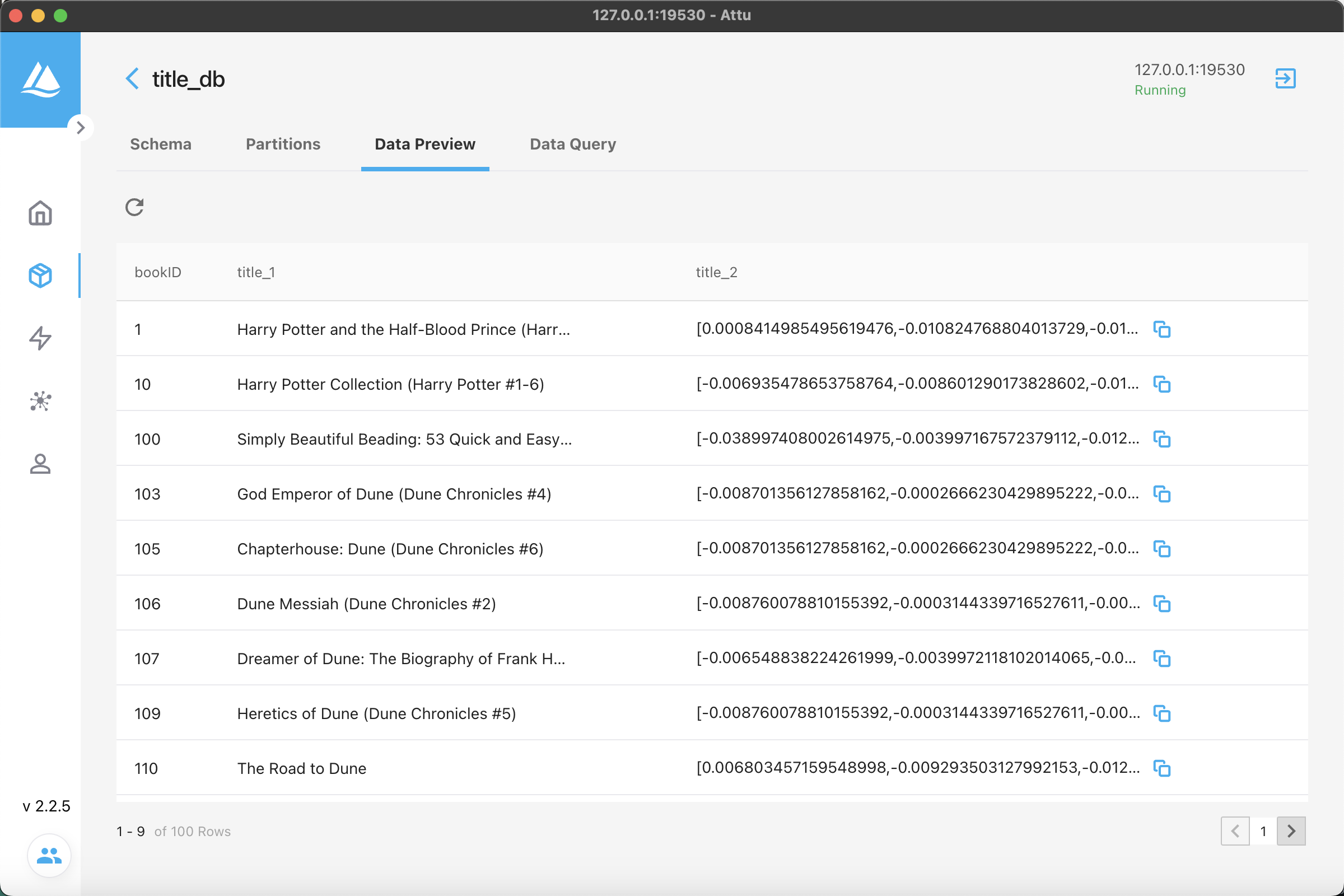
然后使用如下代码通过语义搜索书名:
import json
import random
import openai
import time
from pymilvus import connections, FieldSchema, CollectionSchema, DataType, Collection, utility
COLLECTION_NAME = 'title_db' # Collection name
DIMENSION = 1536 # Embeddings size
COUNT = 100 # How many titles to embed and insert.
MILVUS_HOST = 'localhost' # Milvus server URI
MILVUS_PORT = '19530'
OPENAI_ENGINE = 'text-embedding-ada-002' # Which engine to use
openai.api_key = 'sk-******' # Use your own Open AI API Key here
connections.connect(host=MILVUS_HOST, port=MILVUS_PORT)
collection = Collection(name=COLLECTION_NAME)
collection.load()
def embed(text):
return openai.Embedding.create(
input=text,
engine=OPENAI_ENGINE)["data"][0]["embedding"]
def search(text):
# Search parameters for the index
search_params={
"metric_type": "L2"
}
results=collection.search(
data=[embed(text)], # Embeded search value
anns_field="title_2", # Search across embeddings
param=search_params,
limit=5, # Limit to five results per search
output_fields=['title_1'] # Include title field in result
)
ret=[]
for hit in results[0]:
row=[]
row.extend([hit.id, hit.score, hit.entity.get('title_1')]) # Get the id, distance, and title for the results
ret.append(row)
return ret
search_terms=['self-improvement', 'landscape']
for x in search_terms:
print('Search term:', x)
for result in search(x):
print(result)
print()
搜索结果如下:
Search term: self-improvement
[96, 0.4079835116863251, "The Dance of Intimacy: A Woman's Guide to Courageous Acts of Change in Key Relationships"]
[56, 0.41880303621292114, 'Nicomachean Ethics']
[76, 0.4309804439544678, 'Possession']
[19, 0.43588975071907043, 'Vanity Fair']
[7, 0.4423919916152954, 'Knowledge Is Power (The Amazing Days of Abby Hayes: #15)']
Search term: landscape
[9, 0.3023473024368286, 'The Lay of the Land']
[1, 0.3906732499599457, 'The Angry Hills']
[78, 0.392495334148407, 'Cloud Atlas']
[95, 0.39346450567245483, 'Alien']
[94, 0.399422287940979, 'The Known World']
如果我们按照之前的老方法——关键词搜索,书名中必须包含自我提升、提升等关键词;但是提供大模型进行语义级别的理解,则可以检索到更加符合我们需求的书名。比如在上面的例子中,我们搜索的关键词为self-improvement(自我提升),展示的书名《关系之舞:既亲密又独立的相处艺术》、《尼各马可伦理学》等虽然不包含相关关键词,却很明显更加符合我们的要求。
可见,我们利用Apache SeaTunnel、Milvus和OpenAI,通过大语言模型的方法,可以实现更加精准的书名相似度搜素,为图书搜索领域带来重大的技术突破,同时对于语义理解也提供了有价值的参考,希望可以给大家带来一些启发。
本文由 白鲸开源 提供发布支持!
Recommend
About Joyk
Aggregate valuable and interesting links.
Joyk means Joy of geeK