

Assessing the value of synthetic data for AI - public policy issues take center...
source link: https://diginomica.com/assessing-value-synthetic-data-ai-public-policy-issues-take-center-stage
Go to the source link to view the article. You can view the picture content, updated content and better typesetting reading experience. If the link is broken, please click the button below to view the snapshot at that time.
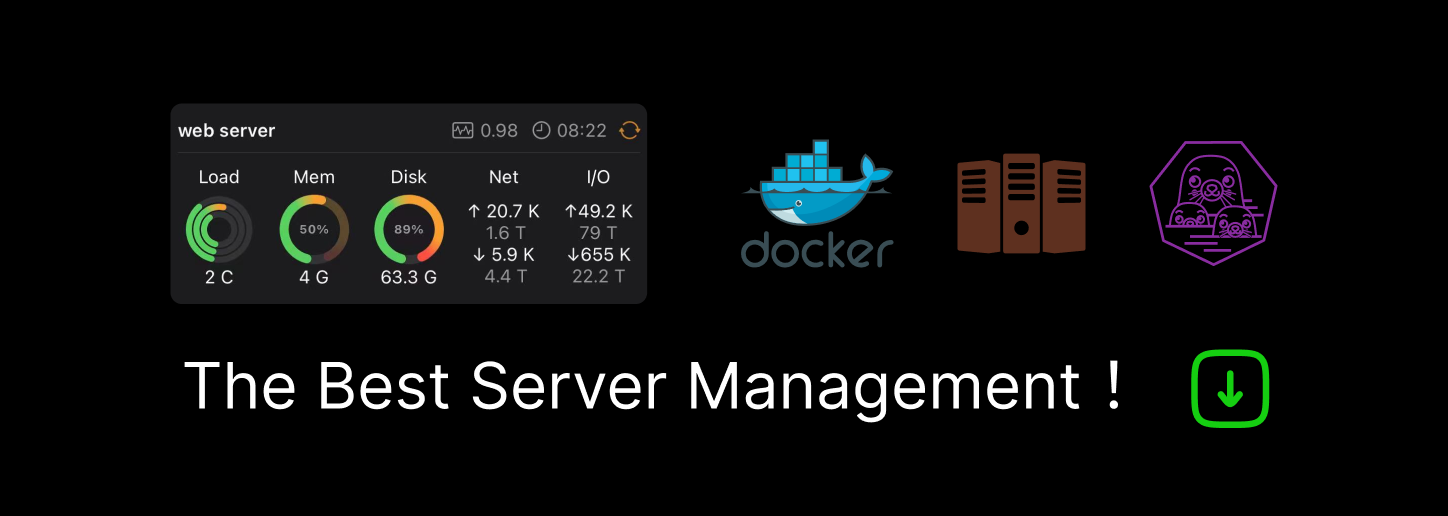
Assessing the value of synthetic data for AI - public policy issues take center stage

(© Rhyzi - Shutterstock)
I've written about synthetic data before, but I recently found an interesting article, Synthetic Data and Public Policy: supporting real-world policymakers with algorithmically generated data.
Two of my primary concerns about this are cited in the paper. I am still waiting to hear a good counter-argument.
A definition of synthetic data is data that has been generated from real data and that has some or all the same statistical properties as the real-world dataset it stands in for. What is problematic about this definition are "real data" and “some or all of the statistical properties.”
What exactly is real data? If the datasets are inadequate for analysis, how real is the data? Part of the problem is the contention that there often needs to be more data to make decent predictions. This claim is derived from a mistaken conception of the truth is in the data. The first sentence of the article says, "Good policy is best developed by drawing on a wide array of high-quality evidence." But not exclusively. Experience, domain knowledge, and hypothesis formation are just as necessary.
“Some or all of the statistical properties.”
The basic idea is simple: you use a model to capture the relationships in the real-world dataset, and then you use the model to generate synthetic data that preserves those relationships. This sounds plausible, but crafting these models is more art than science. Relationships can be spurious. False positives and false negatives can result from including variables that aren’t relevant and excluding others that are.
It is indisputable that selecting the variables for the model injects the modeler’s bias toward the subject matter.
Assuming that the fidelity of your model is high, it begs the question, why not use the model as is? Why generate the synthetic data at all? Doesn’t the model tell you what you need to know? A particularly troubling proposal is to remedy the under-sampling of minority groups. A synthetic dataset based on the census could add a correction to make it more proportional to what the data is expected to contain. Statistically, this only serves to increase the credibility of predictions about those groups that the “real data” doesn’t deserve.
In addition, isn’t the under-representation a crucial analytical fact in its own right? For example, I want to know the number of people surveyed by groups in a survey. If I see 60% vendors, 15% consultants and only 25% CDOs, I will cast a jaundiced eye toward any inferences about CDO input into CDO questions. Synthetically inflating the credibility of certain groups isn't a plausible approach.
A commenter on LinkedIn made a clear argument against the data augmentation process:
One of the valuable components of analysis is quantifying the uncertainty of your conclusions due to the volume of data you have. Couldn't introducing synthetic data artificially inflate the confidence of an analysis?
There are some hyperbolic opinions about the impact of synthetic data. In the article, for example:
Rob Toews believes this new technology is approaching a critical inflection point regarding real-world implications. It is poised to upend the entire value chain and technology stack for artificial intelligence, with immense economic implications' (Toews, 2022).
Immense economic implications in the AI technology stack result from thousands of small to large innovations.
Privacy use case for synthetic data has some potential
Proponents of synthetic data also propose its usefulness for protecting privacy, an entirely separate use case. In correspondence with Alexandra Ebert, Chief Trust Officer of the Austrian company MOSTLY AI, she said:
One of the main reasons for the hype around synthetic data is that it resolves the privacy-utility-trade of legacy anonymization techniques.
She refers to masking, encrypting or deleting PII (Personally Indefinable Information) "…and thus doesn't come with the well-known drawbacks."
Which are that they don't work. It is too easy for a bad actor to defeat the scheme or a reasonable actor to expose protected information inadvertently. Masking or anonymizing data proved to be inadequate. From the article:
Using synthetic data addresses several different kinds and levels of privacy risks: 'singling out the possibility of distinguishing and identifying individual people; ‘linkability’ – the ability to link two or more data points concerning the same data subject within one or more datasets; and ‘inference’ – the possibility of deducing, with significant probability, the value given to other attributes within the dataset.
When solving human problems, it helps to have human data, not clumps of them. Consider medical research. Masking or deleting any PII weakens the dataset by removing features relevant to the investigations.
However, changing a dataset to immunize it against unlawful disclosure of personal information is not new. In fact, in some cases, the lack of the PII can render a dataset useless. A researcher may need to have that for their experiments.
A curious element in the paper was confusing some privacy protection techniques with synthetic data, quoting from Differential Privacy and the 2020 US Census:
For the 2020 US census, the Census Bureau decided to release high-fidelity synthetic data that incorporated a form of 'differential privacy.' This is an advanced technique to further reduce the risk of an individual being identified, basically through adding random values – 'noise' – to the dataset at controlled levels. Notably, differential privacy allows government census agencies to precisely quantify the probability of an individual being identified through the synthetic dataset.
Differential Privacy, which I wrote about here, which uses randomized perturbation has nothing to do with generating synthetic data. It preserves the sensitive data by introducing noise resolved through a probabilistic model.
Earlier attempts to comply with privacy regulations involved more sophisticated methods such as homomorphic encryption, a cryptographic approach make it mathematically impossible crack the data and identify people. Unfortunately, these techniques have one problem: as soon as you try to use them for a complex project, there are efficiency, utility, and scalability problems.
My take
I can see in principle how synthetic data can solve some disclosure problems, and I'm beginning to see a plausible use case for dealing with PII. There is a ton of material online about synthetic data, most of which is from vendors and journalists, and very little raising the questions I've raised.
The article raises some issues worth considering and, like all academic papers, has a boatload of references and citations worth reviewing to get a more comprehensive set of opinions than my own. If you want to use data to get at the "truth," elaborating on what is already suspected is not a good solution.
Recommend
-
62
除非特别声明,此文章内容采用知识共享署名 3.0许可,代码示例采用Apache 2.0许可。更多细节请查看我们的服务条款。
-
2
Biden's Economic Policy Centers Electric Vehicles and BatteriesResponses (7)
-
10
PPC Google updates AdSense Policy center look, adds new feature updates for advertisers The announcement also indicates that upco...
-
5
Navigating in Gridworld using Policy and Value Iteration Learn how to use policy evaluation, policy iteration, and value iteration to find the shortest path in gridworld.
-
4
Public preview: Synthetic GraphQL We use optional cookies to improve your experience on our websites, such as through social media connections,...
-
3
Press Release Mindtech Launch Part Five in Synthetic Data Series, Assessing Synthetic Data’s Place on the Gartner Hype Cycle
-
5
Center For AI And Digital Policy Files Complaint To Slow Down AI Development ...
-
4
Center for AI and Digital Policy asks FTC to suspend commercial deployments of OpenAI's LLMs...
-
4
In addition to its Automated Content policy, Google clarified its existing Merchant Center policies and enforcement behavior.
-
7
GenAI quick wins focus on potential productivity improvements These will likely come from productivity assistants, such as Microsoft...
About Joyk
Aggregate valuable and interesting links.
Joyk means Joy of geeK