
3

Extract only birds sound from audio
source link: https://donghao.org/2023/06/09/extract-only-birds-sound-from-audio/
Go to the source link to view the article. You can view the picture content, updated content and better typesetting reading experience. If the link is broken, please click the button below to view the snapshot at that time.
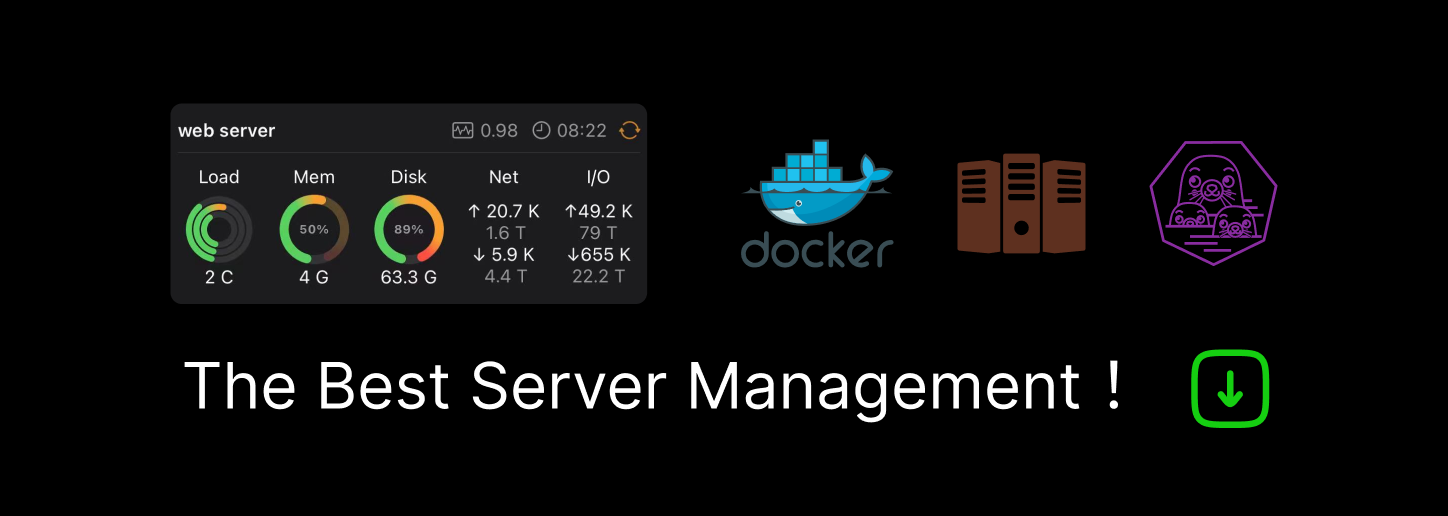
Extract only birds sound from audio
This paper introduced a method to extract only segments with bird sound from an audio file. Since the paper didn’t give any code, I started to write it by myself.
Here is the Python implementation:
import cv2 import time import torch import librosa import soundfile as sf import numpy as np from torchlibrosa.stft import LogmelFilterBank, Spectrogram class CFG: n_fft = 2048 hop_length = 512 sample_rate = 32000 n_mels = 64 fmin = 150 fmax = 150000 class SignalExtractor: def __init__(self): self.spectrogram_extractor = Spectrogram( n_fft=CFG.n_fft, hop_length=CFG.hop_length, win_length=CFG.n_fft, window="hann", center=True, pad_mode="reflect", freeze_parameters=True) # Logmel feature extractor self.logmel_extractor = LogmelFilterBank(sr=CFG.sample_rate, n_fft=CFG.n_fft, n_mels=CFG.n_mels, fmin=CFG.fmin, fmax=CFG.fmax, ref=1.0, amin=1e-10, top_db=None, freeze_parameters=True) self.factors = [2.0, 1.8, 1.6, 1.4, 1.2, 1.1] self.kernel_size = 15 self.sn_threshold = 0.2 def extract(self, input): x = torch.from_numpy(input) x = x[None, :].float() x = self.spectrogram_extractor(x) x = self.logmel_extractor(x) x = x.squeeze(0).squeeze(0) x = x.permute(1, 0).numpy() x = x - np.amin(x) for factor in self.factors: sound, sn_ratio = self._factor_extract(input, x, factor) if sn_ratio >= self.sn_threshold: break return sound, sn_ratio def _factor_extract(self, input, x, factor: float): rows, cols = x.shape row_median = np.median(x, axis=1) row_median_matrix = np.tile(row_median, (cols, 1)).T * factor col_median = np.median(x, axis=0) col_median_matrix = np.tile(col_median, (rows, 1)) * factor y = x > row_median_matrix z = x > col_median_matrix res = np.logical_and(y, z) + np.zeros(x.shape) kernel = np.ones((self.kernel_size, self.kernel_size), np.uint8) img = cv2.dilate(res, kernel, iterations=1) indicator = np.sum(img, axis=0) chunk_size = input.shape[0] // indicator.shape[0] sounds = [] for index, chunk in enumerate(indicator): if chunk > 0: sounds.append(input[index*chunk_size:(index+1)*chunk_size]) if len(sounds) <= 0: return None, 0.0 sound = np.concatenate(sounds) return sound, sound.shape[0]/input.shape[0]
Python
import cv2
import time
import torch
import librosa
import soundfile as sf
import numpy as np
from torchlibrosa.stft import LogmelFilterBank, Spectrogram
class CFG:
n_fft = 2048
hop_length = 512
sample_rate = 32000
n_mels = 64
fmin = 150
fmax = 150000
class SignalExtractor:
def __init__(self):
self.spectrogram_extractor = Spectrogram(
n_fft=CFG.n_fft, hop_length=CFG.hop_length, win_length=CFG.n_fft, window="hann",
center=True, pad_mode="reflect", freeze_parameters=True)
# Logmel feature extractor
self.logmel_extractor = LogmelFilterBank(sr=CFG.sample_rate, n_fft=CFG.n_fft,
n_mels=CFG.n_mels, fmin=CFG.fmin, fmax=CFG.fmax, ref=1.0, amin=1e-10, top_db=None, freeze_parameters=True)
self.factors = [2.0, 1.8, 1.6, 1.4, 1.2, 1.1]
self.kernel_size = 15
self.sn_threshold = 0.2
def extract(self, input):
x = torch.from_numpy(input)
x = x[None, :].float()
x = self.spectrogram_extractor(x)
x = self.logmel_extractor(x)
x = x.squeeze(0).squeeze(0)
x = x.permute(1, 0).numpy()
x = x - np.amin(x)
for factor in self.factors:
sound, sn_ratio = self._factor_extract(input, x, factor)
if sn_ratio >= self.sn_threshold:
break
return sound, sn_ratio
def _factor_extract(self, input, x, factor: float):
rows, cols = x.shape
row_median = np.median(x, axis=1)
row_median_matrix = np.tile(row_median, (cols, 1)).T * factor
col_median = np.median(x, axis=0)
col_median_matrix = np.tile(col_median, (rows, 1)) * factor
y = x > row_median_matrix
z = x > col_median_matrix
res = np.logical_and(y, z) + np.zeros(x.shape)
kernel = np.ones((self.kernel_size, self.kernel_size), np.uint8)
img = cv2.dilate(res, kernel, iterations=1)
indicator = np.sum(img, axis=0)
chunk_size = input.shape[0] // indicator.shape[0]
sounds = []
for index, chunk in enumerate(indicator):
if chunk > 0:
sounds.append(input[index*chunk_size:(index+1)*chunk_size])
if len(sounds) <= 0:
return None, 0.0
sound = np.concatenate(sounds)
return sound, sound.shape[0]/input.shape[0]
Recommend
About Joyk
Aggregate valuable and interesting links.
Joyk means Joy of geeK