

What Neeva's quiet exit tells us about the future of AI startups
source link: https://www.supervised.news/p/what-neevas-quiet-exit-tells-us-about
Go to the source link to view the article. You can view the picture content, updated content and better typesetting reading experience. If the link is broken, please click the button below to view the snapshot at that time.
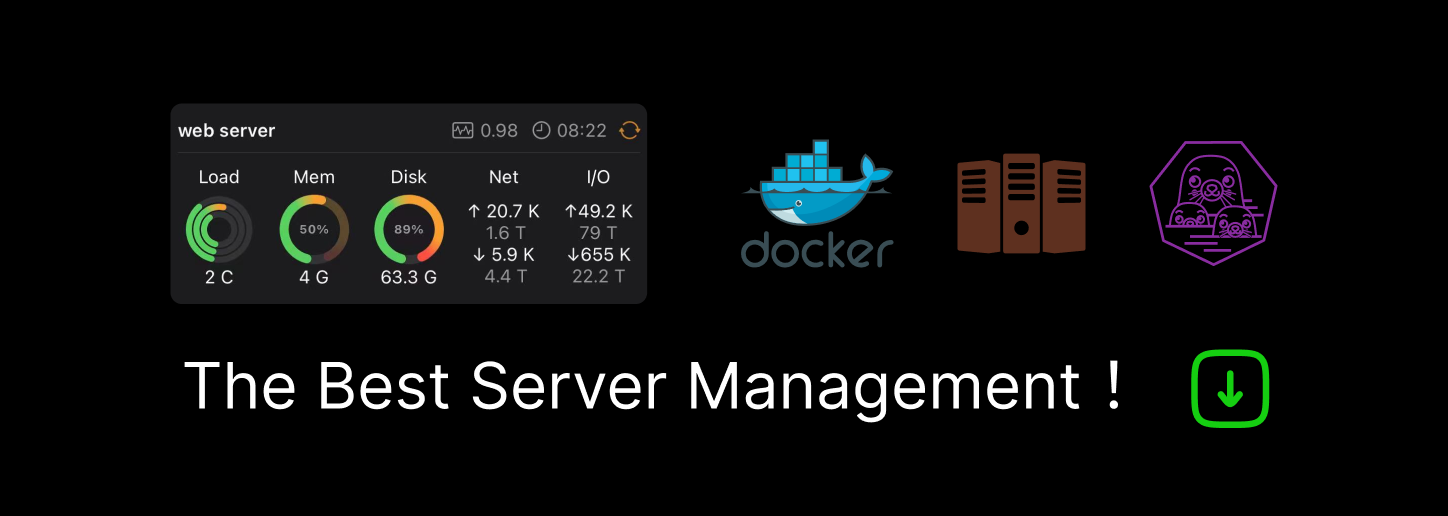
What Neeva's quiet exit tells us about the future of AI startups
Snowflake picked up serious star power in its acquihire of Neeva as it looks to break into machine learning. We'll see a lot more of this.
Author’s note: while today’s issue is focused on Snowflake’s acquisition of Neeva, I wanted to touch on the Nvidia stock market jump briefly—so it’ll be structured a little differently today.
In addition, next week will be the final week where Supervised is a completely free product. Beginning in June I will be moving one issue per week behind a paywall, in addition to all posts that are more than two weeks old. You can read more about it below.
Snowflake, synonymous with the data warehouse, has been trying to crack into machine learning and data science for a few years through a variety of strategies like supporting Python and acquiring machine learning platform Streamlit for an eye-popping $800 million.
This week it acquired another well-known generative AI startup, Neeva, founded by the former Google advertising lead Sridhar Ramaswamy. But Snowflake is not buying an AI-powered search engine with Neeva.
Neeva’s acquisition—or acquihire, as most people consider it—feels like the current nature of the AI industry summed up: technology in service to an ambiguous end-user (consumer vs. enterprise), star power draw, immense opportunity, and enormous vulnerability to the largest incumbents.
The startup, from what I’ve heard, was acquired for around $150 million—though there’s always some nuance when it comes to retention bonuses and such in acquisitions like this. And most I talk to consider getting Ramaswamy in the door as one of the dominant driving factors of the deal. Forbes reported Neeva’s valuation was $300 million in a funding round in March 2021.
Neeva tried to make a paid version of a search engine that would avoid getting stuffed full of ads like Google did and offer better privacy. In the process, Neeva raised around $77.5 million from investors including Greylock and Sequoia.
Neeva launched NeevaAI in January this year to compete with a future that was going to be more dominated with AI-generated search results in Google and Bing. That didn’t pan out, and earlier this week Ramaswamy said the company would be focusing on enterprise LLMs.
“Many of the techniques we have pioneered with small models, size reduction, latency reduction, and inexpensive deployment are the elements that enterprises really want, and need, today,” he wrote in a blog post announcing the shutdown of the consumer product.
His announcement shutting down the product came around the same time The Information reported that Snowflake was in advanced talks to acquire it. Neeva was also in conversations with other potential buyers at the time, according to several sources I spoke with.
In his note shutting down Neeva’s search product, essentially pointed to the cognitive switching costs of moving to a different search engine as a primary culprit for its failure to gain traction. Regardless of whether Google or Bing’s generative AI search experience would be a hit with users (which is an enormous TBD) didn’t really matter given the size of the market they’d already accumulated.
“Convincing users to pay for a better experience was actually a less difficult problem compared to getting them to try a new search engine in the first place,” he wrote.
The Takeaway
In the end, we have an enterprise data warehousing company, which is trying to build a machine learning development platform, acquiring an AI-powered consumer search engine that shut down due to the the sustained inertia built up by incumbents. The tools Neeva built for consumers were uniquely suited for enterprise needs, and Neeva couldn’t beat Google and Microsoft with a better product experience.
It’s the type of story we’re going to see a lot more going forward as the industry continues to develop.
Snowflake, meanwhile, sits in a unique position in that it’s already claimed an enormous part of the data stack for modern companies (particularly startups), and all that data will inevitably be used for training and fine-tuning models at some point. Bringing in a team like Neeva’s gives it some on-the-ground experience building and deploying models to figure out how that workflow will work.
Where the Neeva team fits into Snowflake from an organizational standpoint remains an open question. From what I’ve heard, Streamlit founder Adrien Treuille has taken on more leadership in Snowflake’s machine learning organization beyond just Streamlit. It’s also unclear what tools it’s carrying over and integrating from Neeva.
But it’s also netting a very well-known and well-respected figure in the industry that can serve as a steward as it continues to move into machine learning products. Particularly as Snowflake faces an uphill battle convincing a now critical community that Databricks has already served for years. Databricks, too, has tapped the OSS zeitgeist with its own large language model, Dolly 2.0.
The kind of crossover experience you see from the 50-person Neeva team is going to continue to gain value as time goes on. And non-intuitive enterprise companies—particularly platform plays like Snowflake playing in a highly competitive market—will probably end up being landing places for a lot of the “consumer” AI startups that fail to gain substantial traction.
Everyone is building on top of the same technology, and the skill sets of building and running an AI platform will soon grow well beyond standard machine learning engineering. The question is which of these teams will be able to build lasting products, and which will end up with soft landings at companies scrambling to build out AI-focused teams.
Now on to Nvidia, a company that some seem to have discovered makes AI hardware as of this week.
Nvidia’s earnings report Wednesday came out about as glowing as they could have hoped for following announcements from Meta and Google that they were building superclusters based off Nvidia’s hardware. Following some very optimistic projections in growth for its data center business—its chips that power machine training and inference—Nvidia’s stock went up something like 20+% overnight.
Nvidia is just shy of being one of a number of trillion-dollar companies thanks to its emergence as the standard for AI hardware with no clear challenger on the horizon for machine training with its A100 and H100 series chips. Many startups launched to try to challenge Nvidia for machine training, but so far none have made significant progress in unseating it from foundation model development.
Not to be the obnoxious hipster here who first liked GPUs when they were on vinyl, but the writing has been on the wall for Nvidia’s AI business to go ballistic for years now. Its early growth period just happened to coincide with a time where everyone was obsessed with crypto mining rigs rather than the developments coming out of Google, FAIR, OpenAI, and others.
The sudden surge in its stock this week has really not that much to do with Nvidia’s AI business and more with ChatGPT’s ongoing march into the mainstream, this time infiltrating the magic Wall Street algorithms that oversee whatever Calvinball rules the stock market has today.
The stock market is one of those things that, as a journalist (and probably most people), you feel terrible talking/writing about every time because it’s so often exists in an alternate plane of reality. More than anything else at a given moment, it’s a measurement of some combination of sentiment and zeitgeist.
One of Nvidia’s fastest-growing businesses now just dovetails onto a more broader hype-y mainstream sentiment, which depending on the day is some flavor of what AI will create, displace, revolutionize, destroy, undo, re-do, un-re-do, re-revolutionize after un-revolutionizing, and also paperclips.
If there is anything to come of all this at least, the public market tends to be a leading indicator of how startup valuations will go. And now there are suddenly many, many, many more eyes on the total addressable market that is AI model training and inference hardware. And it’s likely many more mainstream LPs interested in another trillion-dollar slot in the S&P 500 are eyeing the same opportunity.
How that materializes given the historical track record of machine training chip startups is still to be determined, especially since even AMD hasn’t made a true dent in Nvidia.
But I do, however, know of a very large number of standing open offers among tech executives that are more than ready to try out a different kind of hardware than what Nvidia offers if a truly competitive one ever actually materializes.
ICYMI
OpenAI warns over split with Europe as regulation advances (Financial Times): Sam Altman comes in to once again say he hopes to comply with regulations, but if OpenAI can’t, it will pull out of Europe. Given how often Europe is a vanguard in tech regulation, I’m increasingly feeling that this stance is less about OpenAI wanting to dodge regulation and more about OpenAI’s inexperience with how to manage the (quite new) meta-discussion around regulation here.
Why Fake Drake Is Here to Stay (Wired): Lauren Goode and Gideon Lichfield have a wonderful interview with Puja Patel, the editor in chief of Pitchfork, about the future of generative AI in the music industry. Puja oversees one of the most important publications in the music industry and has a great view of the cultural impact voice transcription and synthesis models will have on music in general.
OpenAI Closes $175 Million Startup Fund (The Information): OpenAI’s debut fund for seeding startups powered on ChatGPT comes in way ahead of target, according to a filing spotted by Kate Clark. Of course, this isn’t like Slack where you need to build a fund to prod and coax companies into building on your platform—so we’ll see in what way OpenAI puts this money to work.
Former GitHub CTO Jason Warner Raises $26 Million for Foundation Model Code Startup (Newcomer): Eric Newcomer talks to Warner and co-founder Eiso Kant about their new startup targeting language models focused on code. It might seem like a hefty seed funding round, but training an actual foundation model from the ground up is quite costly even with the work being done by MosaicML and others.
Model evaluation for extreme risks (ArXiv): A new paper out of DeepMind this week offers some guidelines on how to evaluate models for extreme risk, which it lays out multiple examples and tries to build some codification around it. It’s loaded with great, much more explicit definitions, and might serve as a good catalog going forward for what risk we’re monitoring and how we evaluate them.
Also: building a real business
I've been overwhelmed by the level of support everyone has given for Supervised in its first month of launch. Things have gone about as smoothly as I could have hoped. So now for the next step: turning this into sustainable operation.
Starting in June, one issue per week will be going behind a paywall, while two will remain free. The archived stories for Supervised will also begin going behind a paywall two weeks after publication starting in June.
This decision is based on a lot of conversations with existing and new readers, as well as the invaluable mentors that have shared their time and suggestions. I am still absorbing your feedback on the format, the length, and other ways the newsletter could improve, so please continue send me your thoughts!
Thank you to everyone for all your help, suggestions, and patience as I get this thing off the ground. And, of course, thank you for reading!
Supervised is a reader-supported publication. To receive new posts and support my work, consider becoming a free or paid subscriber.
Still on my radar
What does a startup built around AutoGPT look like?
What progress is Character AI making on foundation models?
How do feature stores like Tecton fit into the future of vector databases?
Which funds are investing in startups working on vector embedding models?
If you have any tips (or answers to any of the above), please send me a note at [email protected] or contact me directly on Signal at +1-415-690-7086. As always, please send any and all feedback my way.
Recommend
-
3
Ad-free Search on Neeva with Darin Fisher By SE Daily Podcast Tuesday, January 25 2022 ...
-
14
Say hello to CHAI CHAI, a short news product by nextbigwhat is meant for ambitious professionals like you who prefer more signals vs. noise. Read less....
-
4
Neeva is Shutting Down Its Privacy-First, Ad-Free $4.95-a-Month Search Engine
-
6
Neeva, the would-be Google competitor, is shutting down its search engine / “We’ve discovered that it is one thing to build a search engine, and an entirely different thing to convince regular users of the need to swi...
-
3
Google search competitor Neeva shuts down to focus on enterprise
-
4
Google search rival Neeva is shutting down its search engine to work on AI products...
-
6
TechNeeva, co-founded by a former Google exec, to shut down its consumer search prod...
-
8
Neeva beat Google and Microsoft in launching generative AI search with citations. But the ad-free search engine didn't attract enough users.
-
4
Next Steps for Neeva
-
5
Snowflake deal appears imminent as Neeva shuts down search, pivots to enterprise LLMs
About Joyk
Aggregate valuable and interesting links.
Joyk means Joy of geeK