

What have we learned about AI ethics? It's time for risk-based regulations
source link: https://diginomica.com/what-have-we-learned-about-ai-ethics-its-time-risk-based-regulations
Go to the source link to view the article. You can view the picture content, updated content and better typesetting reading experience. If the link is broken, please click the button below to view the snapshot at that time.
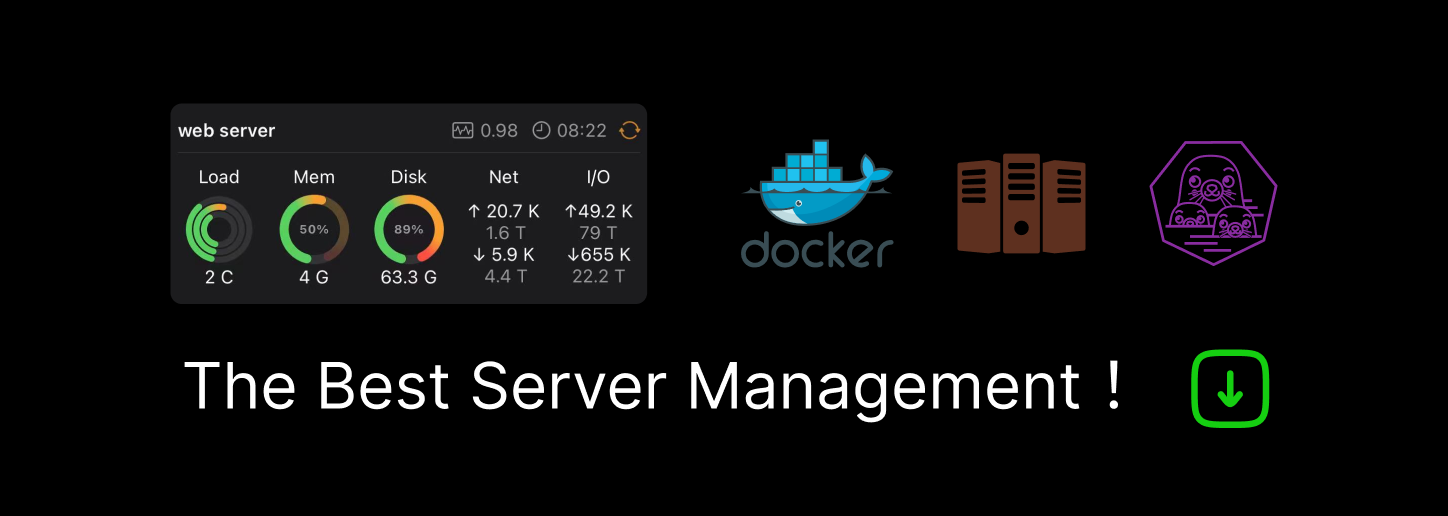
What have we learned about AI ethics? It's time for risk-based regulations

In the period from roughly 2015 until today, the focus on AI "ethics" centered on two issues:
- Will AI cause the extinction of human civilization, if not human existence entirely?
- Will the application of AI only exacerbate the problems of bias, discrimination, division, and unfairness, via automated “black box” systems?
AI technology has proceeded so quickly that it's time to review these concerns in light of what we know today. The first, while still a long-term concern, is less pressing than the second, but it has morphed into a broader issue:
- We learned that lecturing organizations about ethics has only limited effect in convincing people to be more ethical in their AI work, especially the organizations that invent new technology and applications in AI.
- We also learned that focusing on risk instead of ethics is a more powerful and effective method to motivate organizations to use AI for good, not evil.
Countries within the Group of Seven political forum have signed a declaration agreeing on the need for "risk-based" AI regulations. Top technology officials from Britain, Canada, the EU, France, Germany, Italy, Japan and the United States signed the joint statement to establish parameters for how major countries govern the technology. Most notably, the G7, NIST, and the FTC have all released bold statements about the risk to organizations. As per the G7 statement:
We also reassert that AI policies and regulations should be risk-based and forward-looking to preserve an open and enabling environment for AI development and deployment that maximizes the benefit of the technology for people and the planet while mitigating its risks.
While earlier releases of proposed principles and guidelines focused primarily on the danger of disenfranchisement of "marginalized" populations, they were effectively "all carrot, no stick." Today, we see even the Federal Trade Commission (FTC) aggressively pursuing a policy of using their existing power and statutes. In Aiming for truth, fairness, and equity in your company's use of AI, Elisa Jillson of the FTC, in an atypical fashion, put U.S. organizations on notice that the FTC would use its various powers to investigate AI abuses, and presumably take action: "Hold yourself accountable - or be ready for the FTC to do it for you."
It is encouraging to see the FTC take steps to make companies accountable for AI abuses.
The NIST (National Institute of Standards and Technology) AI RMF (Risk Management Framework) is a set of high-level voluntary guidelines and recommendations that organizations can follow to assess and manage risks stemming from using AI (the framework is still under development; NIST has posted the latest iterations. It is not a statute, regulation or law.
The AI RMF aims to support organizations of all sizes to "prevent, detect, mitigate and manage AI risks." It is intended for any organization developing, commissioning or deploying AI systems and is designed to be non-prescriptive, industry and use-case agnostic.
While the NIST AI RMF can prioritize risk, it does not prescribe risk tolerance. Risk tolerance refers to the organization's or AI actor's readiness to bear the risk to achieve its objectives. Risk tolerance and the risk acceptable to organizations or society are highly contextual, application, and use-case specific. Risk tolerances can be influenced by policies and norms established by AI system owners, organizations, industries, communities, or policymakers. Risk tolerances will change as AI systems, policies, and standards evolve.
Organizations may have varied risk tolerances due to their organizational priorities and resource considerations. Emerging knowledge and methods to better inform harm/cost-benefit tradeoffs will continue to be developed and debated by businesses, governments, academia, and civil society. To the extent that challenges for specifying AI risk tolerances still need to be solved, there may be contexts where a risk management framework still needs to be readily applicable for mitigating adverse AI risks. The NIST Framework is intended to be flexible and augment existing risk practices that align with applicable laws, regulations, and norms.
There is no question that AI is now essential to our lives, improving capabilities in societal challenges in many sectors, from healthcare to agriculture, finance, and law enforcement. Although AI has the potential to transform society positively, it also poses potential legal, ethical, and societal risks that regulators and policymakers must address.
The European Commission's proposal for the Artificial Intelligence Act, the first globally of its kind, aimed to address AI regulation in a risk-based approach, categorizing AI applications based on perceived levels of risk. It proposed an outright ban on specific AI applications, stringent requirements for high-risk AI systems, and a limited set of transparency requirements for lower-risk AI applications. The European Parliament is currently debating the proposal, and lengthy negotiations have resulted in an expanded list of high-risk AI systems in Annex III, deviating from its original objectives.
Regulating AI is crucial to protect fundamental rights while laying the foundation for innovation.
However, categorizing AI based on levels of risk and excluding or including applications that pose a risk, even if they do not fit the profile, can lead to an exercise that becomes quickly outdated, stifling future innovation. Furthermore, a focus on risk categorization may fail to regulate or over-regulate AI systems and hinder smaller players' development due to extensive conformity assessments.
The EU approach to risk is still aimed at the risk to people affected by the application of AI. To be effective, the focus should shift to the risk organizations face to their own harm. This comes in the form of damage to reputation and trust, loss of customer loyalty, and even litigation and fines. A risk-based approach focusing on crucial AI issues and organizational accountability requirements can address these concerns. A future-proof AI regulation should allow for the evolution of AI, enabling organizations to adapt to new developments and use cases, a constantly changing risk taxonomy, and a seemingly endless range of applications. Regulatory sandboxes can mitigate the challenge faced by smaller organizations, which may find the extensive conformity assessment required for high-risk AI challenging without extensive resources or a frame of reference.
Large Language Models (LLM) are one such application that demonstrates the importance of focusing on the application and associated risks. LLMs are trained on broad data sets and can be fine-tuned for a wide range of downstream tasks, acting as the foundation for hundreds of other applied models. The seemingly limitless capacity of LLMs may pose regulatory challenges that require careful consideration to develop an appropriate regulatory framework.
The clear and present danger of LLMs is their foundation in language. Language expresses - and even defines - culture. The unbridled expansion of language-based applications poses the risk of defining culture, blurring the line between what is real and what is artificial by socializing the idea that organizations face real and significant risks from relying on LLMs without sufficiently understanding their behavior. Even developers of LLMs point out the limitations of deep learning that can lead to "hallucinations" and "gradient diminishment," leading to misinformation, and even bizarre behavior.
My take
A risk-based approach that focuses on the application and associated risk, combined with organizational accountability requirements, can address legal, ethical, and societal risks while providing a foundation for innovation. It is essential to strike a balance between regulation and innovation while allowing for the evolution of AI.
Recommend
-
4
Romance & Polyamory I guess the biggest deal for me is discovering that polyamory 1) exists 2) is something I’ve been missing a lot in my interpersonal relations. It’s the big one because it most directly affected me, and...
-
4
Thing I Have Learned About Software Testing 2011-08-29 by qntm Firstly, it's a thing. I...
-
4
Arbitrage of cryptocurrencies: What I have learned from my experiences
-
10
Executive InsiderWhat Leaders Have Learned A Year into a Global PandemicYou have watched the Hero’s Journey play out in every Disney movie since you were a c...
-
5
Sponsored by WSO2 Need to improve developer productivity and enable collaboration between low-code and pro-code users? Use Choreo - an integration platform as a service that offers low-code cloud-native engineering for profess...
-
4
Have Rights Owners Learned from the Mistakes of the Failed ESL?Skip to content
-
3
The state of AI ethics: The principles, the tools, the regulations Image Credit: Getty Images Join today's leading executives onl...
-
11
Ten years of page bloat: What have we learned?
-
6
AI - where do ethics stop, and regulations begin? By Chris Middleton
-
4
Should There Be Enforceable Ethics Regulations on Generative AI?Private sector, voluntary guidelines on the ethical use of generative AI are emerging, yet no US government body regulates the technology currently.
About Joyk
Aggregate valuable and interesting links.
Joyk means Joy of geeK