

Some Neural Networks Learn Language Like Humans
source link: https://www.quantamagazine.org/some-neural-networks-learn-language-like-humans-20230522/
Go to the source link to view the article. You can view the picture content, updated content and better typesetting reading experience. If the link is broken, please click the button below to view the snapshot at that time.
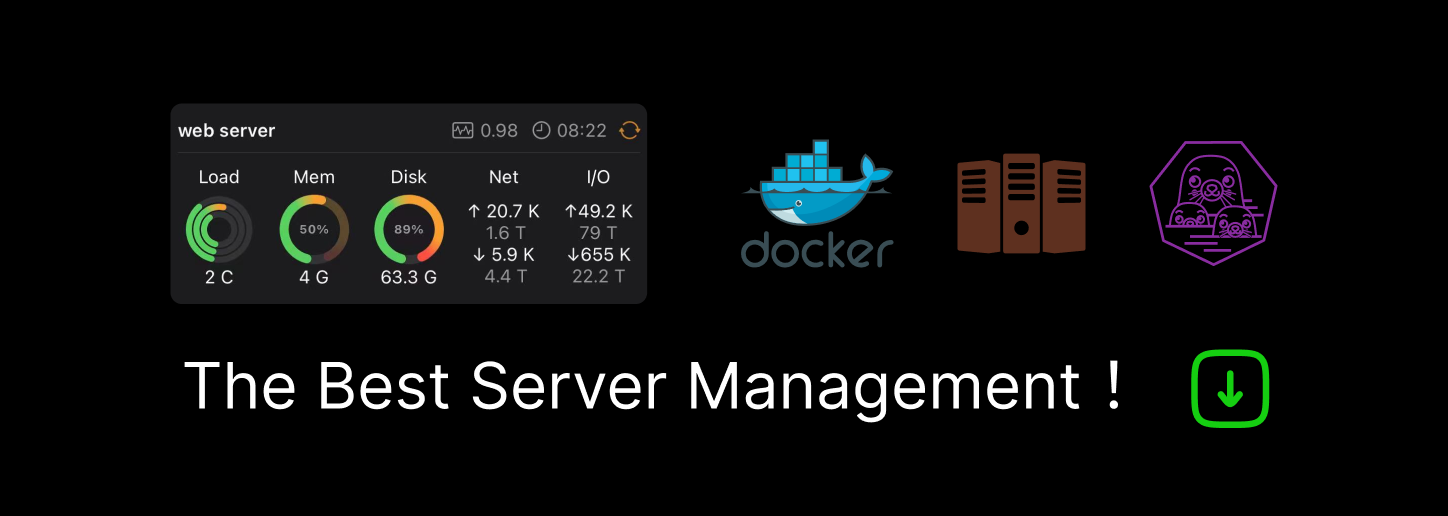
Introduction
How do brains learn? It’s a mystery, one that applies both to the spongy organs in our skulls and to their digital counterparts in our machines. Even though artificial neural networks (ANNs) are built from elaborate webs of artificial neurons, ostensibly mimicking the way our brains process information, we don’t know if they process input in similar ways.
“There’s been a long-standing debate as to whether neural networks learn in the same way that humans do,” said Vsevolod Kapatsinski, a linguist at the University of Oregon.
Now, a study published last month suggests that natural and artificial networks learn in similar ways, at least when it comes to language. The researchers — led by Gašper Beguš, a computational linguist at the University of California, Berkeley — compared the brain waves of humans listening to a simple sound to the signal produced by a neural network analyzing the same sound. The results were uncannily alike. “To our knowledge,” Beguš and his colleagues wrote, the observed responses to the same stimulus “are the most similar brain and ANN signals reported thus far.”
Most significantly, the researchers tested networks made up of general-purpose neurons that are suitable for a variety of tasks. “They show that even very, very general networks, which don’t have any evolved biases for speech or any other sounds, nevertheless show a correspondence to human neural coding,” said Gary Lupyan, a psychologist at the University of Wisconsin, Madison who was not involved in the work. The results not only help demystify how ANNs learn, but also suggest that human brains may not come already equipped with hardware and software specially designed for language.
To establish a baseline for the human side of the comparison, the researchers played a single syllable — “bah” — repeatedly in two eight-minute blocks for 14 English speakers and 15 Spanish speakers. While it played, the researchers recorded fluctuations in the average electrical activity of neurons in each listener’s brainstem — the part of the brain where sounds are first processed.
In addition, the researchers fed the same “bah” sounds to two different sets of neural networks — one trained on English sounds, the other on Spanish. The researchers then recorded the processing activity of the neural network, focusing on the artificial neurons in the layer of the network where sounds are first analyzed (to mirror the brainstem readings). It was these signals that closely matched the human brain waves.

Merrill Sherman/Quanta Magazine
The researchers chose a kind of neural network architecture known as a generative adversarial network (GAN), originally invented in 2014 to generate images. A GAN is composed of two neural networks — a discriminator and a generator — that compete against each other. The generator creates a sample, which could be an image or a sound. The discriminator determines how close it is to a training sample and offers feedback, resulting in another try from the generator, and so on until the GAN can deliver the desired output.
In this study, the discriminator was initially trained on a collection of either English or Spanish sounds. Then the generator — which never heard those sounds — had to find a way of producing them. It started out by making random sounds, but after some 40,000 rounds of interactions with the discriminator, the generator got better, eventually producing the proper sounds. As a result of this training, the discriminator also got better at distinguishing between real and generated ones.

It was at this point, after the discriminator was fully trained, that the researchers played it the “bah” sounds. The team measured the fluctuations in the average activity levels of the discriminator’s artificial neurons, which produced the signal so similar to the human brain waves.
This likeness between human and machine activity levels suggested that the two systems are engaging in similar activities. “Just as research has shown that feedback from caregivers shapes infant productions of sounds, feedback from the discriminator network shapes the sound productions of the generator network,” said Kapatsinski, who did not take part in the study.
The experiment also revealed another interesting parallel between humans and machines. The brain waves showed that the English- and Spanish-speaking participants heard the “bah” sound differently (Spanish speakers heard more of a “pah”), and the GAN’s signals also showed that the English-trained network processed the sounds somewhat differently than the Spanish-trained one.
“And those differences work in the same direction,” explained Beguš. The brainstem of English speakers responds to the “bah” sound slightly earlier than the brainstem of Spanish speakers, and the GAN trained in English responded to that same sound slightly earlier than the Spanish-trained model. In both humans and machines, the difference in timing was almost identical, roughly a thousandth of a second. This provided additional evidence, Beguš said, that humans and artificial networks are “likely processing things in a similar fashion.”
Recommend
About Joyk
Aggregate valuable and interesting links.
Joyk means Joy of geeK