

Go与神经网络:张量运算
source link: https://tonybai.com/2023/05/21/go-and-nn-part1-tensor-operations/
Go to the source link to view the article. You can view the picture content, updated content and better typesetting reading experience. If the link is broken, please click the button below to view the snapshot at that time.
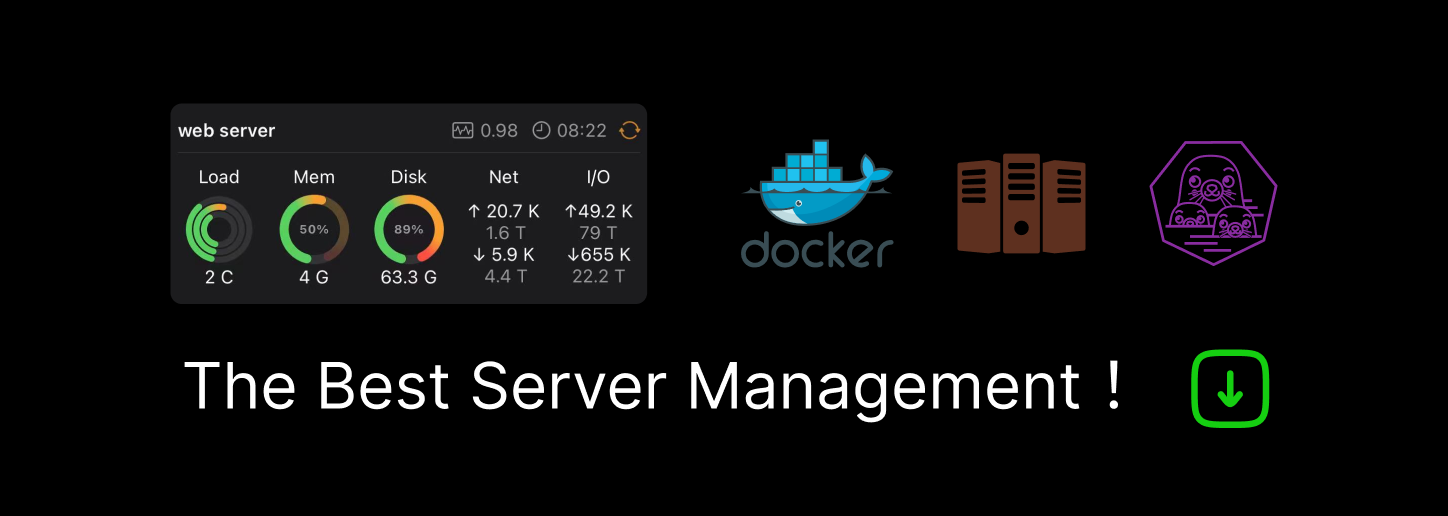
Go与神经网络:张量运算

本文永久链接 – https://tonybai.com/2023/05/21/go-and-nn-part1-tensor-operations
2023年年初,我们很可能是见证了一次新工业革命的起点,也可能是见证了AGI(Artificial general intelligence,通用人工智能)孕育的开始。ChatGPT应用以及后续GPT-4大模型的出现,其震撼程度远超当年AlphaGo战胜人类顶尖围棋选手。相对于AlphaGo在一个狭窄领域的建树,ChatGPT则是以摧枯拉朽之势横扫几乎所有脑力劳动行业。
如今大家更多将ChatGPT及相关应用当做生产力工具,作为程序员,自然会首当其冲的加入到借助AI提升生产力的阵营。但对于程序员来说,如果对一个计算机科学方面的技术没有基本工作原理认知或是完全看不懂,那么就会有一种深深的危机感。
什么是深度学习、什么是神经网络、什么是大模型、上千亿的参数究竟指的是什么、什么是大模型的量化等都是萦绕在头脑中的未知但又急切想知道的东西。
有人会说,深度学习发展都十多年了,现在学还来得及么?其实大多数人不是从事机器学习的,普通程序员只需要了解机器学习、深度学习(神经网络)的基本运作原理即可。此外,有了ChatGPT相关工具后,获取和理解知识的效率可以大幅提升,以前需要以年来计算学习新知识技能,现在可能仅需以月来计算,甚至更短。
作为程序员,了解深度学习,了解神经网络,其实也是去学习一种新的、完全不同于以往的编程范式。以前我们的编程范式是这样的: 人类学习规则,然后通过手工编码将规则内置到系统中,系统运行后,根据明确的规则对输入数据做处理并给出答案(如下图所示):

这个大编程范式通常又细分为下面几类,大家根据自己的喜好和工作要求选择不同的编程范式以及编程语言:
- 命令式编程范式(C、Go等);
- 面向对象编程范式(Java、Ruby);
- 函数式编程范式(Haskell、Lisp、Clojure等);
- 声明式编程范式(SQL)。
这类范式都归属于符号主义人工智能(symbolic AI),即都是用来手工编写明确的规则的。符号主义人工智能适合用来解决定义明确的逻辑问题,比如下国际象棋,但它难以给出明确规则来解决更复杂、更模糊的问题,比如图像分类、语音识别或自然语言翻译。
而机器学习或者说机器学习的结果人工神经网络则是另外一种范式,如下图所示:

在这个范式中,程序员无需再学习什么规则,因为规则是模型自己通过数据学习来的。程序员只需准备好高质量的训练数据以及对应的答案(标注),然后建立初始模型(初始神经网络)即可,之后的事情就交给机器了(机器学习并非在数学方面做出什么理论突破,而是“蛮力出奇迹”一个生动案例)。模型通过数据进行自动训练(学习)并生成包含规则的目标模型,而目标模型即程序。
了解两类截然不同的范式之后,我再来澄清几个问题:
- Go与神经网络系列文章的目的?不是教你如何自己搞出一个大模型,而是将经典机器学习、深度学习(与建立人工神经网络)的来龙去脉搞清楚。
- Why Go? 帮助Go程序员学习机器学习。虽然Python代码看起来很容易理解,代码量也会少很多(像Keras这样的框架,甚至将training dataset都集成在框架中了)。
注:通过阅读Python的机器学习/深度学习代码,我觉得不会有什么语言可以代替Python作为AI主力了。用Python做数据准备、训练模型简直简单的不要不要的了。
- 从何处开始?张量以及相关运算。
张量在深度学习中扮演着非常重要的角色,因为它们是存储和处理数据的基本单位。张量可以看作是一个“容器”,可以表示向量、矩阵和更高维度的数据结构。深度学习中的神经网络模型使用张量来表示输入数据、模型参数和输出结果,以及在计算过程中的各种中间变量。通过对张量进行数学运算和优化,深度学习模型能够从大量的数据中学习到特征和规律,并用于分类、回归、聚类等任务。因此,张量是深度学习中必不可少的概念之一。最流行的深度学习框架tensorflow都以tensor命名。我们也将从张量(tensor)出发进入机器学习和神经网络世界。
不过大家要区分数学领域与机器学习领域张量在含义上的不同。在数学领域,张量是一个多维数组,而在机器学习领域,张量是一种数据结构,用于表示多维数组和高维矩阵。两者的相同点在于都是多维数组,但不同点在于它们的应用场景和具体实现方式不同。如上一段描述那样,在机器学习中,张量通常用于表示数据集、神经网络的输入和输出等。
下面我们就来了解一下张量与张量的运算,包括如何创建张量、执行基本和高级张量操作,以及张量广播(broadcast)与重塑(reshape)操作。
1. 理解张量
张量是目前所有机器学习系统都使用的基本数据结构。张量这一概念的核心在于,它是一个数据容器。它包含的数据通常是同类型的数值数据,因此它是一个同构的数字容器。
前面提到过,张量可以表示数字、向量、矩阵甚至更高维度的数据。很多语言采用多维数组来实现张量,不过也有采用平坦数组(flat array)来实现的,比如:gorgonia/tensor。
无论实现方式是怎样的,从逻辑上看,张量的表现是一致的,即张量是一个有如下属性的同构数据类型。
1.1 阶数(ndim)
张量的维度通常叫作轴(axis),张量轴的个数也叫作阶(rank)。下面是从0阶张量到4阶张量的示意图:

仅包含一个数字的张量,也被称为标量(scalar),也叫标量张量。0阶张量有0个轴。
1阶张量,也称为向量(vector),有一个轴。这个向量可以是n维向量,与张量的阶数没有关系。比如在上面图中的一阶张量表示的就是一个4维向量。所谓维度即沿着某个轴上的元素的个数。这个图中一阶张量表示的向量中有4个元素,因此是一个4维向量。
2阶张量,也称为矩阵(matrix),有2个轴。在2阶张量中,这两个轴也称为矩阵的行(axis-0)和列(axis-1),每个轴上的向量都有自己的维度。例如上图中的2阶张量的axis-0轴上有3个元素(每个元素又都是一个向量),因此是axis-0的维度为3,由此类推,axis-1轴的维度为4。
注:张量的轴的下标从0开始,如axis-0、axis-1、…、axis-n。
2阶张量也可以看成是1阶张量的数组。
- 3阶或更高阶张量
3阶张量有3个轴,如上图中的3阶张量,可以看成是多个2阶张量组成的数组。
以此类推,扩展至N阶张量,可以看成是N-1阶张量的数组。
1.2 形状(shape)。
张量有一个属性为shape,shape由张量每个轴上的维度(轴上元素的个数)组成。以上图中的3阶张量为例,其axis-0轴上有2个元素,axis-1轴上有3个元素,axis-2轴上有4个元素,因此该3阶张量的shape为(2, 3, 4)。axis-0轴也被称为样本轴,下图是按照每一级张量的样本轴对张量做拆解的示意图:

我们首先对3阶张量(shape(2,3,4))沿着其样本轴方向进行拆解,我们将其拆解2个2阶张量(shape(3,4))。接下来,我们对得到的2阶张量进行拆解,同样沿着其样本轴方向拆解为3个1阶张量(shape(4,))。我们看到,每个1阶张量是一个4维向量,可拆解为4个0阶张量。
1.3 元素数据类型dtype
张量是同构数据类型,无论是几阶张量,最终都是由一个个标量组合而成,标量的类型就是张量的元素数据类型(dtype),在上图中,我们的张量的dtype为float32。浮点数与整型数是机器学习中张量最常用的元素数据类型。
了解了张量的概念与属性后,我们就来看看在Go中如何创建张量。
2. 在Go中创建张量
Go提供了几个机器学习库,可以用来创建和操作张量。在Go中执行张量操作的两个流行库是Tensorflow和Gorgonia。
不过Tensorflow官方团队已经不再对go binding API提供维护支持了(由Go社区第三方负责维护),并且该binding需要依赖cgo调用tensorflow的库,因此在本文中,我们使用gorgonia来定义张量以及进行张量运算。
Gorgonia提供了tensor包用来定义tensor并提供基于tensor的基本运算函数。下面的例子使用tensor包定义了对应上面图中1阶到3阶的三个张量:
// https://github.com/bigwhite/experiments/blob/master/go-and-nn/tensor-operations/tensor.go
package main
import (
"fmt"
"gorgonia.org/tensor"
)
func main() {
// define an one-rank tensor
oneRankTensor := tensor.New(tensor.WithBacking([]float32{1.7, 2.6, 1.3, 3.2}), tensor.WithShape(4))
fmt.Println("\none-rank tensor:")
fmt.Println(oneRankTensor)
fmt.Println("ndim:", oneRankTensor.Dims())
fmt.Println("shape:", oneRankTensor.Shape())
fmt.Println("dtype", oneRankTensor.Dtype())
// define an two-rank tensor
twoRankTensor := tensor.New(tensor.WithBacking([]float32{1.7, 2.6, 1.3, 3.2,
2.7, 2.8, 1.5, 2.9,
3.7, 2.4, 1.7, 3.1}), tensor.WithShape(3, 4))
fmt.Println("\ntwo-rank tensor:")
fmt.Println(twoRankTensor)
fmt.Println("ndim:", twoRankTensor.Dims())
fmt.Println("shape:", twoRankTensor.Shape())
fmt.Println("dtype", twoRankTensor.Dtype())
// define an three-rank tensor
threeRankTensor := tensor.New(tensor.WithBacking([]float32{1.7, 2.6, 1.3, 3.2,
2.7, 2.8, 1.5, 2.9,
3.7, 2.4, 1.7, 3.1,
1.5, 2.7, 1.4, 3.3,
2.5, 2.8, 1.9, 2.9,
3.5, 2.5, 1.7, 3.6}), tensor.WithShape(2, 3, 4))
fmt.Println("\nthree-rank tensor:")
fmt.Println(threeRankTensor)
fmt.Println("ndim:", threeRankTensor.Dims())
fmt.Println("shape:", threeRankTensor.Shape())
fmt.Println("dtype", threeRankTensor.Dtype())
}
tensor.New接受一个变长参数列表,这里我们显式传入了存储张量数据的平坦数组数据以及tensor的shape属性,这样我们便能得到一个满足我们要求的tensor变量。运行上面程序,你将看到下面内容:
$ASSUME_NO_MOVING_GC_UNSAFE_RISK_IT_WITH=go1.20 go run tensor.go
one-rank tensor:
[1.7 2.6 1.3 3.2]
ndim: 1
shape: (4)
dtype float32
two-rank tensor:
⎡1.7 2.6 1.3 3.2⎤
⎢2.7 2.8 1.5 2.9⎥
⎣3.7 2.4 1.7 3.1⎦
ndim: 2
shape: (3, 4)
dtype float32
three-rank tensor:
⎡1.7 2.6 1.3 3.2⎤
⎢2.7 2.8 1.5 2.9⎥
⎣3.7 2.4 1.7 3.1⎦
⎡1.5 2.7 1.4 3.3⎤
⎢2.5 2.8 1.9 2.9⎥
⎣3.5 2.5 1.7 3.6⎦
ndim: 3
shape: (2, 3, 4)
dtype float32
tensor.New返回的*tensor.Dense类型实现了fmt.Stringer接口,可以按shape形式打印出tensor,但是人类肉眼也就识别到3阶tensor吧。3阶以上的tensor输出的格式用人眼识别和理解就有些困难了。
此外,我们看到Gorgonia的tensor包基于平坦的数组来存储tensor数据,tensor包根据shape属性对数组中数据做切分,划分出不同轴上的数据。数组的元素类型可以自定义,如果我们使用float64的切片,那么tensor的dtype就为float64。
3. Go中的基本张量运算
现在我们知道了如何使用Gorgonia/tensor创建张量了,让我们来探索Go中的一些基本张量运算。
3.1. 加法和减法
将两个相同形状(shape)的张量相加或相减是机器学习算法中的一个常见操作。在Go中,我们可以使用Gorgonia/tensor提供的Add和Sub函数进行加减操作。下面是一个使用tensor包进行加减运算的示例代码片断:
// https://github.com/bigwhite/experiments/blob/master/go-and-nn/tensor-operations/tensor_add_sub.go
func main() {
// define two two-rank tensor
ta := tensor.New(tensor.WithBacking([]float32{1.7, 2.6, 1.3, 3.2,
2.7, 2.8, 1.5, 2.9,
3.7, 2.4, 1.7, 3.1}), tensor.WithShape(3, 4))
fmt.Println("\ntensor a:")
fmt.Println(ta)
tb := tensor.New(tensor.WithBacking([]float32{1.7, 2.6, 1.3, 3.2,
2.7, 2.8, 1.5, 2.9,
3.7, 2.4, 1.7, 3.1}), tensor.WithShape(3, 4))
fmt.Println("\ntensor b:")
fmt.Println(ta)
tc, _ := tensor.Add(ta, tb)
fmt.Println("\ntensor a+b:")
fmt.Println(tc)
td, _ := tensor.Sub(ta, tb)
fmt.Println("\ntensor a-b:")
fmt.Println(td)
// add in-place
tensor.Add(ta, tb, tensor.UseUnsafe())
fmt.Println("\ntensor a+b(in-place):")
fmt.Println(ta)
// tensor add scalar
tg, err := tensor.Add(tb, float32(3.14))
if err != nil {
fmt.Println("add scalar error:", err)
return
}
fmt.Println("\ntensor b+3.14:")
fmt.Println(tg)
// add two tensors of different shape
te := tensor.New(tensor.WithBacking([]float32{1.7, 2.6, 1.3,
3.2, 2.7, 2.8}), tensor.WithShape(2, 3))
fmt.Println("\ntensor e:")
fmt.Println(te)
tf, err := tensor.Add(ta, te)
fmt.Println("\ntensor a+e:")
if err != nil {
fmt.Println("add error:", err)
return
}
fmt.Println(tf)
}
运行该示例:
$ASSUME_NO_MOVING_GC_UNSAFE_RISK_IT_WITH=go1.20 go run tensor_add_sub.go
tensor a:
⎡1.7 2.6 1.3 3.2⎤
⎢2.7 2.8 1.5 2.9⎥
⎣3.7 2.4 1.7 3.1⎦
tensor b:
⎡1.7 2.6 1.3 3.2⎤
⎢2.7 2.8 1.5 2.9⎥
⎣3.7 2.4 1.7 3.1⎦
tensor a+b:
⎡3.4 5.2 2.6 6.4⎤
⎢5.4 5.6 3 5.8⎥
⎣7.4 4.8 3.4 6.2⎦
tensor a-b:
⎡0 0 0 0⎤
⎢0 0 0 0⎥
⎣0 0 0 0⎦
tensor a+b(in-place):
⎡3.4 5.2 2.6 6.4⎤
⎢5.4 5.6 3 5.8⎥
⎣7.4 4.8 3.4 6.2⎦
tensor b+3.14:
⎡ 4.84 5.74 4.44 6.34⎤
⎢ 5.84 5.94 4.6400003 6.04⎥
⎣ 6.84 5.54 4.84 6.24⎦
tensor e:
⎡1.7 2.6 1.3⎤
⎣3.2 2.7 2.8⎦
tensor a+e:
add error: Add failed: Shape mismatch. Expected (2, 3). Got (3, 4)
我们看到:tensor加减法是一个逐元素(element-wise)进行的操作,要求参与张量运算的张量必须有相同的shape,同位置的两个元素相加,否则会像示例中最后的a+e那样报错;tensor加法支持tensor与一个scalar(标量)进行加减,原理就是tensor中每个元素都与这个标量相加减;此外若传入tensor.Unsafe这个option后,参与加减法操作的第一个tensor的值会被结果重写掉(override)。
3.2. 乘法和除法
两个张量的相乘或相除是机器学习算法中另一个常见的操作。在Go中,我们可以使用Gorgonia/tensor提供的Mul和Div函数进行乘除运算。下面是一个使用Gorgonia/tensor进行乘法和除法运算的示例代码:
// https://github.com/bigwhite/experiments/blob/master/go-and-nn/tensor-operations/tensor_mul_div.go
func main() {
// define two two-rank tensor
ta := tensor.New(tensor.WithBacking([]float32{1.7, 2.6, 1.3, 3.2,
2.7, 2.8, 1.5, 2.9,
3.7, 2.4, 1.7, 3.1}), tensor.WithShape(3, 4))
fmt.Println("\ntensor a:")
fmt.Println(ta)
tb := tensor.New(tensor.WithBacking([]float32{1.7, 2.6, 1.3, 3.2,
2.7, 2.8, 1.5, 2.9,
3.7, 2.4, 1.7, 3.1}), tensor.WithShape(3, 4))
fmt.Println("\ntensor b:")
fmt.Println(tb)
tc, err := tensor.Mul(ta, tb)
if err != nil {
fmt.Println("multiply error:", err)
return
}
fmt.Println("\ntensor a x b:")
fmt.Println(tc)
// multiple tensor and a scalar
td, err := tensor.Mul(ta, float32(3.14))
if err != nil {
fmt.Println("multiply error:", err)
return
}
fmt.Println("\ntensor ta x 3.14:")
fmt.Println(td)
// divide two tensors
td, err = tensor.Div(ta, tb)
if err != nil {
fmt.Println("divide error:", err)
return
}
fmt.Println("\ntensor ta / tb:")
fmt.Println(td)
// multiply two tensors of different shape
te := tensor.New(tensor.WithBacking([]float32{1.7, 2.6, 1.3,
3.2, 2.7, 2.8}), tensor.WithShape(2, 3))
fmt.Println("\ntensor e:")
fmt.Println(te)
tf, err := tensor.Mul(ta, te)
fmt.Println("\ntensor a x e:")
if err != nil {
fmt.Println("mul error:", err)
return
}
fmt.Println(tf)
}
运行该示例,我们可以看到如下结果:
$ASSUME_NO_MOVING_GC_UNSAFE_RISK_IT_WITH=go1.20 go run tensor_mul_div.go
tensor a:
⎡1.7 2.6 1.3 3.2⎤
⎢2.7 2.8 1.5 2.9⎥
⎣3.7 2.4 1.7 3.1⎦
tensor b:
⎡1.7 2.6 1.3 3.2⎤
⎢2.7 2.8 1.5 2.9⎥
⎣3.7 2.4 1.7 3.1⎦
tensor a x b:
⎡ 2.89 6.7599993 1.6899998 10.240001⎤
⎢7.2900004 7.8399997 2.25 8.410001⎥
⎣13.690001 5.76 2.89 9.61⎦
tensor ta x 3.14:
⎡5.3380003 8.164 4.082 10.048⎤
⎢ 8.478001 8.792 4.71 9.106001⎥
⎣11.618001 7.5360007 5.3380003 9.734⎦
tensor ta / tb:
⎡1 1 1 1⎤
⎢1 1 1 1⎥
⎣1 1 1 1⎦
tensor e:
⎡1.7 2.6 1.3⎤
⎣3.2 2.7 2.8⎦
tensor a x e:
mul error: Mul failed: Shape mismatch. Expected (2, 3). Got (3, 4)
我们看到,和加减法一样,tensor的乘除法也是逐元素进行的,同时也支持与scalar的乘除。但对于shape不同的两个tensor,Mul和Div会报错。
了解了加减、乘除等基本操作后,下面我们再探索一写更高级的张量操作。
4. Go中的高级张量操作
除了基本的张量操作外,Go还提供了一些高级的张量操作,用于复杂的机器学习算法中。让我们来探讨一下Go中的一些高级张量操作。
4.1. 点积
点积运算,也叫张量积(tensor product,不要与上面的逐元素的乘积弄混),是线性代数和机器学习算法中的一个作最常见也最有用的张量运算。与逐元素的运算不同,它将输入张量的元素合并在一起。
它涉及到将两个张量元素相乘,然后将结果相加。这里借用鱼书中的图来直观的看一下二阶tensor计算过程:

图中是两个shape为(2, 2)的tensor的点积。
下面是更一般的两个二阶tensor t1和t2:
tensor t1: shape(a, b)
tensor t2: shape(c, d)
t1和t2可以做点积的前提是b == c,即第一个tensor t1的shape[1] == 第二个tensor t2的shape[0]。
在Go中,我们可以Dot函数来实现点积操作。下面是使用Gorgonia/tensor进行点积操作的例子:
// https://github.com/bigwhite/experiments/blob/master/go-and-nn/tensor-operations/tensor_dot.go
func main() {
// define two two-rank tensor
ta := tensor.New(tensor.WithBacking([]float32{1, 2, 3, 4}), tensor.WithShape(2, 2))
fmt.Println("\ntensor a:")
fmt.Println(ta)
tb := tensor.New(tensor.WithBacking([]float32{5, 6, 7, 8}), tensor.WithShape(2, 2))
fmt.Println("\ntensor b:")
fmt.Println(tb)
tc, err := tensor.Dot(ta, tb)
if err != nil {
fmt.Println("dot error:", err)
return
}
fmt.Println("\ntensor a dot b:")
fmt.Println(tc)
td := tensor.New(tensor.WithBacking([]float32{5, 6, 7, 8, 9, 10}), tensor.WithShape(2, 3))
fmt.Println("\ntensor d:")
fmt.Println(td)
te, err := tensor.Dot(ta, td)
if err != nil {
fmt.Println("dot error:", err)
return
}
fmt.Println("\ntensor a dot d:")
fmt.Println(te)
// three-rank tensor dot two-rank tensor
tf := tensor.New(tensor.WithBacking([]float32{23: 12}), tensor.WithShape(2, 3, 4))
fmt.Println("\ntensor f:")
fmt.Println(tf)
tg := tensor.New(tensor.WithBacking([]float32{11: 12}), tensor.WithShape(4, 3))
fmt.Println("\ntensor g:")
fmt.Println(tg)
th, err := tensor.Dot(tf, tg)
if err != nil {
fmt.Println("dot error:", err)
return
}
fmt.Println("\ntensor f dot g:")
fmt.Println(th)
}
运行该示例,我们可以看到如下结果:
$ASSUME_NO_MOVING_GC_UNSAFE_RISK_IT_WITH=go1.20 go run tensor_dot.go
tensor a:
⎡1 2⎤
⎣3 4⎦
tensor b:
⎡5 6⎤
⎣7 8⎦
tensor a dot b:
⎡19 22⎤
⎣43 50⎦
tensor d:
⎡ 5 6 7⎤
⎣ 8 9 10⎦
tensor a dot d:
⎡21 24 27⎤
⎣47 54 61⎦
tensor f:
⎡ 0 0 0 0⎤
⎢ 0 0 0 0⎥
⎣ 0 0 0 0⎦
⎡ 0 0 0 0⎤
⎢ 0 0 0 0⎥
⎣ 0 0 0 12⎦
tensor g:
⎡ 0 0 0⎤
⎢ 0 0 0⎥
⎢ 0 0 0⎥
⎣ 0 0 12⎦
tensor f dot g:
⎡ 0 0 0⎤
⎢ 0 0 0⎥
⎣ 0 0 0⎦
⎡ 0 0 0⎤
⎢ 0 0 0⎥
⎣ 0 0 144⎦
我们看到大于2阶的高阶tensor也可以做点积,只要其形状匹配遵循与前面2阶张量相同的原则:
(a, b, c, d) . (d,) -> (a, b, c)
(a, b, c, d) . (d, e) -> (a, b, c, e)
4.2. 转置
转置张量包括翻转其行和列。这是机器学习算法中的一个常见操作,广泛应用在图像处理和自然语言处理等领域。在Go中,我们可以使用tensor包提供的Transpose函数对tensor进行转置:
// https://github.com/bigwhite/experiments/blob/master/go-and-nn/tensor-operations/tensor_transpose.go
func main() {
// define two-rank tensor
ta := tensor.New(tensor.WithBacking([]float32{1, 2, 3, 4, 5, 6}), tensor.WithShape(3, 2))
fmt.Println("\ntensor a:")
fmt.Println(ta)
tb, err := tensor.Transpose(ta)
if err != nil {
fmt.Println("transpose error:", err)
return
}
fmt.Println("\ntensor a transpose:")
fmt.Println(tb)
// define three-rank tensor
tc := tensor.New(tensor.WithBacking([]float32{1, 2, 3, 4, 5, 6,
7, 8, 9, 10, 11, 12,
13, 14, 15, 16, 17, 18,
19, 20, 21, 22, 23, 24}), tensor.WithShape(2, 3, 4))
fmt.Println("\ntensor c:")
fmt.Println(tc)
fmt.Println("tc shape:", tc.Shape())
td, err := tensor.Transpose(tc)
if err != nil {
fmt.Println("transpose error:", err)
return
}
fmt.Println("\ntensor c transpose:")
fmt.Println(td)
fmt.Println("td shape:", td.Shape())
}
运行上面示例:
$ASSUME_NO_MOVING_GC_UNSAFE_RISK_IT_WITH=go1.20 go run tensor_transpose.go
tensor a:
⎡1 2⎤
⎢3 4⎥
⎣5 6⎦
tensor a transpose:
⎡1 3 5⎤
⎣2 4 6⎦
tensor c:
⎡ 1 2 3 4⎤
⎢ 5 6 7 8⎥
⎣ 9 10 11 12⎦
⎡13 14 15 16⎤
⎢17 18 19 20⎥
⎣21 22 23 24⎦
tc shape: (2, 3, 4)
tensor c transpose:
⎡ 1 13⎤
⎢ 5 17⎥
⎣ 9 21⎦
⎡ 2 14⎤
⎢ 6 18⎥
⎣10 22⎦
⎡ 3 15⎤
⎢ 7 19⎥
⎣11 23⎦
⎡ 4 16⎤
⎢ 8 20⎥
⎣12 24⎦
td shape: (4, 3, 2)
接下来,我们再来探讨两个张量的高级操作:重塑(也叫变形)与广播。
5. 在Go中重塑与广播张量
在机器学习算法中,经常需要对张量进行重塑和广播,使其与不同的操作兼容。Go提供了几个函数来重塑和广播张量。让我们来探讨如何在Go中重塑和广播张量。
5.1. 重塑张量
重塑一个张量涉及到改变它的尺寸到一个新的形状。在Go中,我们可以使用Gorgonia/tensor提供的Dense类型的Reshape方法来重塑张量自身。
下面是一个使用Gorgonia重塑张量的示例代码:
// https://github.com/bigwhite/experiments/blob/master/go-and-nn/tensor-operations/tensor_reshape.go
func main() {
// define two-rank tensor
ta := tensor.New(tensor.WithBacking([]float32{1, 2, 3, 4, 5, 6}), tensor.WithShape(3, 2))
fmt.Println("\ntensor a:")
fmt.Println(ta)
fmt.Println("ta shape:", ta.Shape())
err := ta.Reshape(2, 3)
if err != nil {
fmt.Println("reshape error:", err)
return
}
fmt.Println("\ntensor a reshape(2,3):")
fmt.Println(ta)
fmt.Println("ta shape:", ta.Shape())
err = ta.Reshape(1, 6)
if err != nil {
fmt.Println("reshape error:", err)
return
}
fmt.Println("\ntensor a reshape(1, 6):")
fmt.Println(ta)
fmt.Println("ta shape:", ta.Shape())
err = ta.Reshape(2, 1, 3)
if err != nil {
fmt.Println("reshape error:", err)
return
}
fmt.Println("\ntensor a reshape(2, 1, 3):")
fmt.Println(ta)
fmt.Println("ta shape:", ta.Shape())
}
运行上述代码,我们将看到:
$ASSUME_NO_MOVING_GC_UNSAFE_RISK_IT_WITH=go1.20 go run tensor_reshape.go
tensor a:
⎡1 2⎤
⎢3 4⎥
⎣5 6⎦
ta shape: (3, 2)
tensor a reshape(2,3):
⎡1 2 3⎤
⎣4 5 6⎦
ta shape: (2, 3)
tensor a reshape(1, 6):
R[1 2 3 4 5 6]
ta shape: (1, 6)
tensor a reshape(2, 1, 3):
⎡1 2 3⎤
⎡4 5 6⎤
ta shape: (2, 1, 3)
由此看来,张量转置其实是张量重塑的一个特例,只是将将轴对调。
5.2. 广播张量
广播张量涉及到扩展其维度以使其与其他操作兼容。下面是鱼书中关于广播(broadcast)的图解:

我们看到图中这个标量(Scalar)扩展维度后与第一个张量做乘法操作,与我们前面说到的张量与标量(scalar)相乘是一样的。如上图中这种标量10被扩展成了2 × 2的形状后再与矩阵A进行乘法运算,这个的功能就称为广播(broadcast)。
在鱼书中还提到了“借助这个广播功能,不同形状的张量之间也可以顺利地进行运算”以及下面图中这个示例:

但Gorgonia/tensor包目前并不支持除标量之外的“广播”。
张量操作在机器学习和数据科学中是必不可少的,它允许我们有效地操纵多维数组。在这篇文章中,我们探讨了如何使用Go创建和执行基本和高级张量操作。我们还学习了广播和重塑张量,使它们与不同的机器学习模型兼容。
我希望这篇文章能为后续继续探究深度学习与神经网络奠定一个基础,让你开始探索Go中的张量操作,并使用它们来解决现实世界的问题。
注:说实话,Go在机器学习领域的应用并不广泛,前景也不明朗,零星的几个开源库似乎也不是很活跃。这里也仅是基于Go去学习理解机器学习的概念和操作,真正为生产编写和训练的机器学习模型与程序还是要使用Python。
本文涉及的源码可以在这里下载 – https://github.com/bigwhite/experiments/blob/master/go-and-nn/tensor-operations
7. 参考资料
- 《Python深度学习(第二版)》 – https://book.douban.com/subject/36078304/
- 鱼书《深度学习入门:基于Python的理论与实现》 – https://book.douban.com/subject/30270959/
- 苹果书《深入浅出神经网络与深度学习》 – https://book.douban.com/subject/35128111/
- 《机器学习:Go语言实现》 – https://book.douban.com/subject/30457083/
“Gopher部落”知识星球旨在打造一个精品Go学习和进阶社群!高品质首发Go技术文章,“三天”首发阅读权,每年两期Go语言发展现状分析,每天提前1小时阅读到新鲜的Gopher日报,网课、技术专栏、图书内容前瞻,六小时内必答保证等满足你关于Go语言生态的所有需求!2023年,Gopher部落将进一步聚焦于如何编写雅、地道、可读、可测试的Go代码,关注代码质量并深入理解Go核心技术,并继续加强与星友的互动。欢迎大家加入!
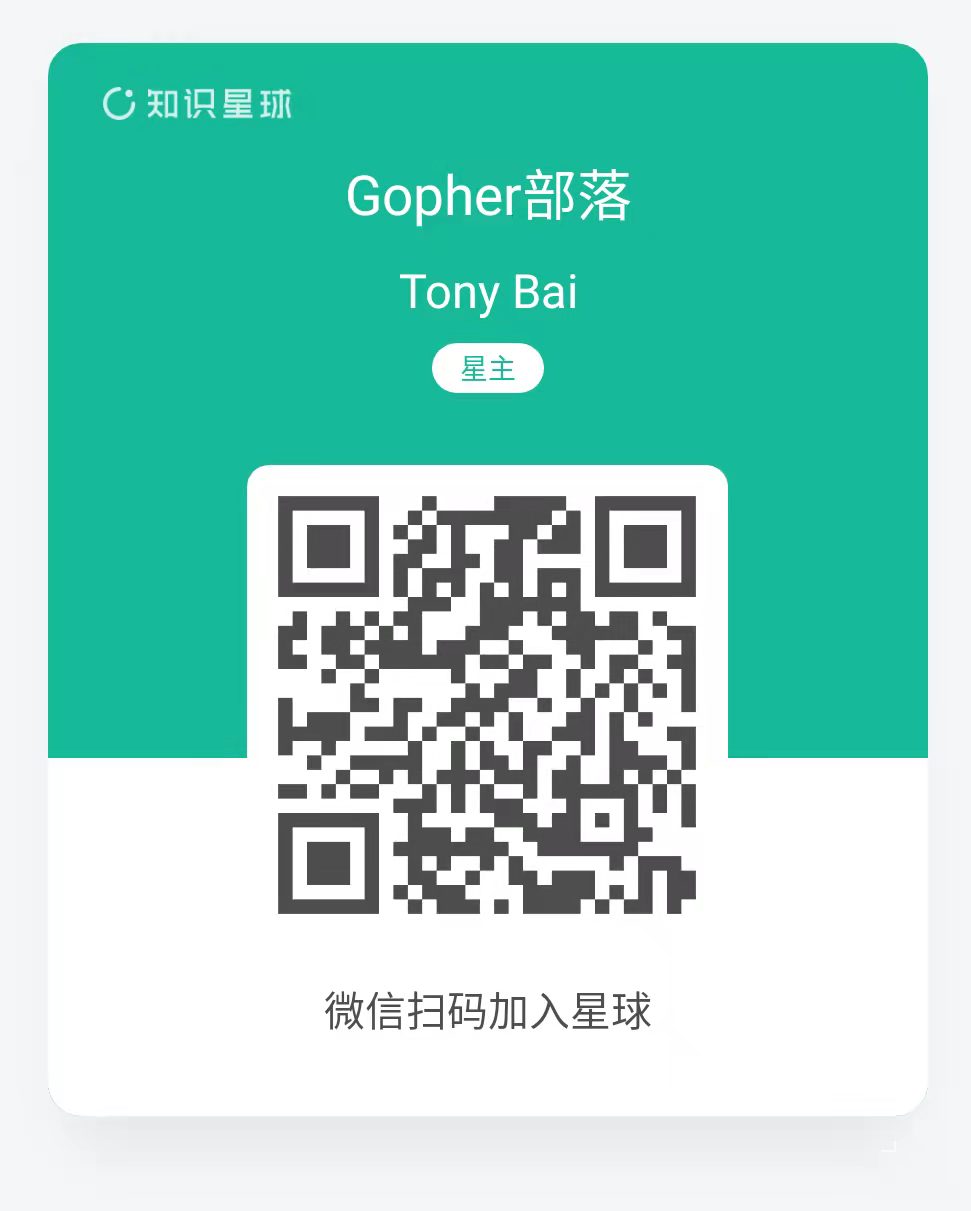
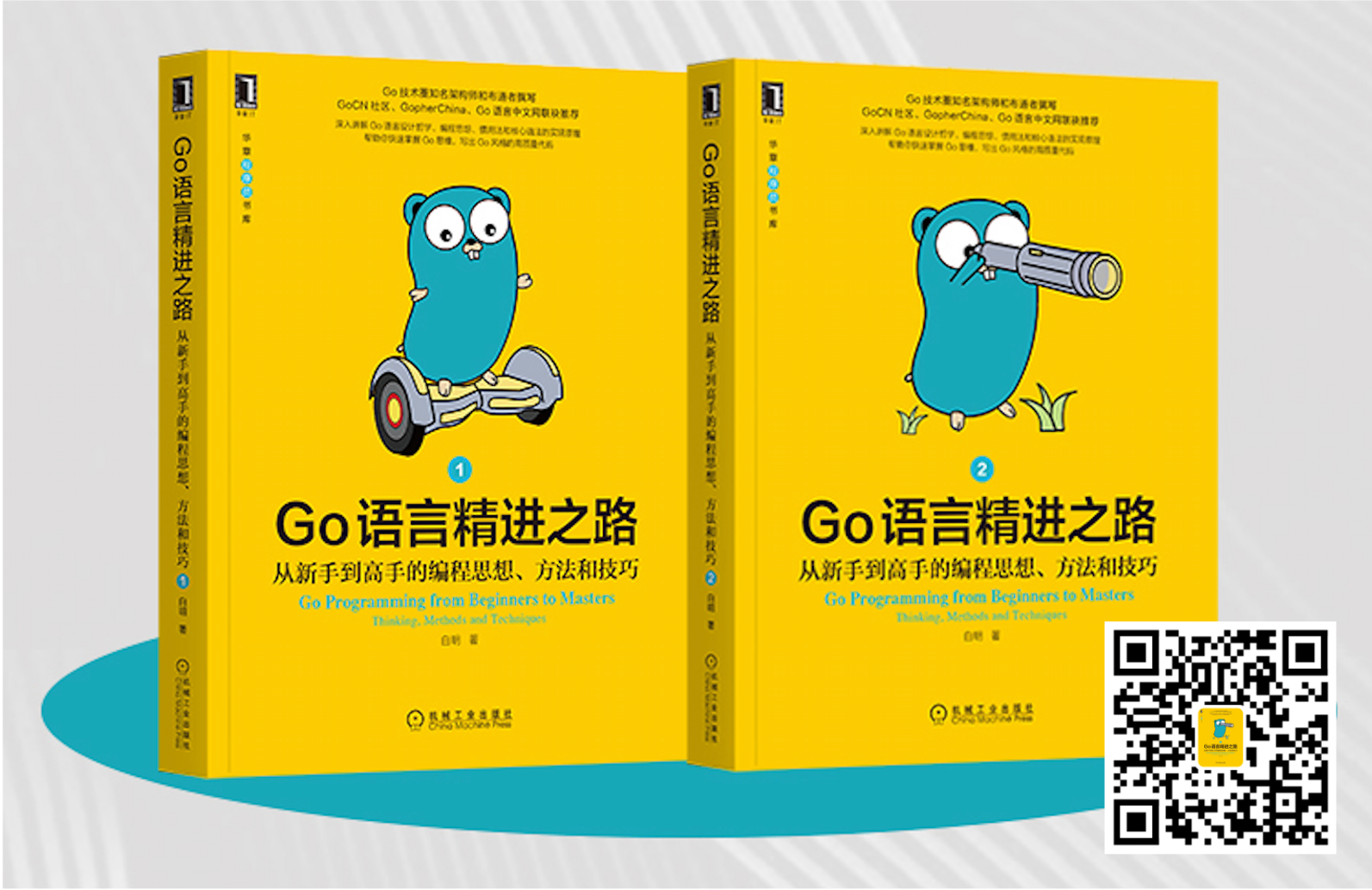
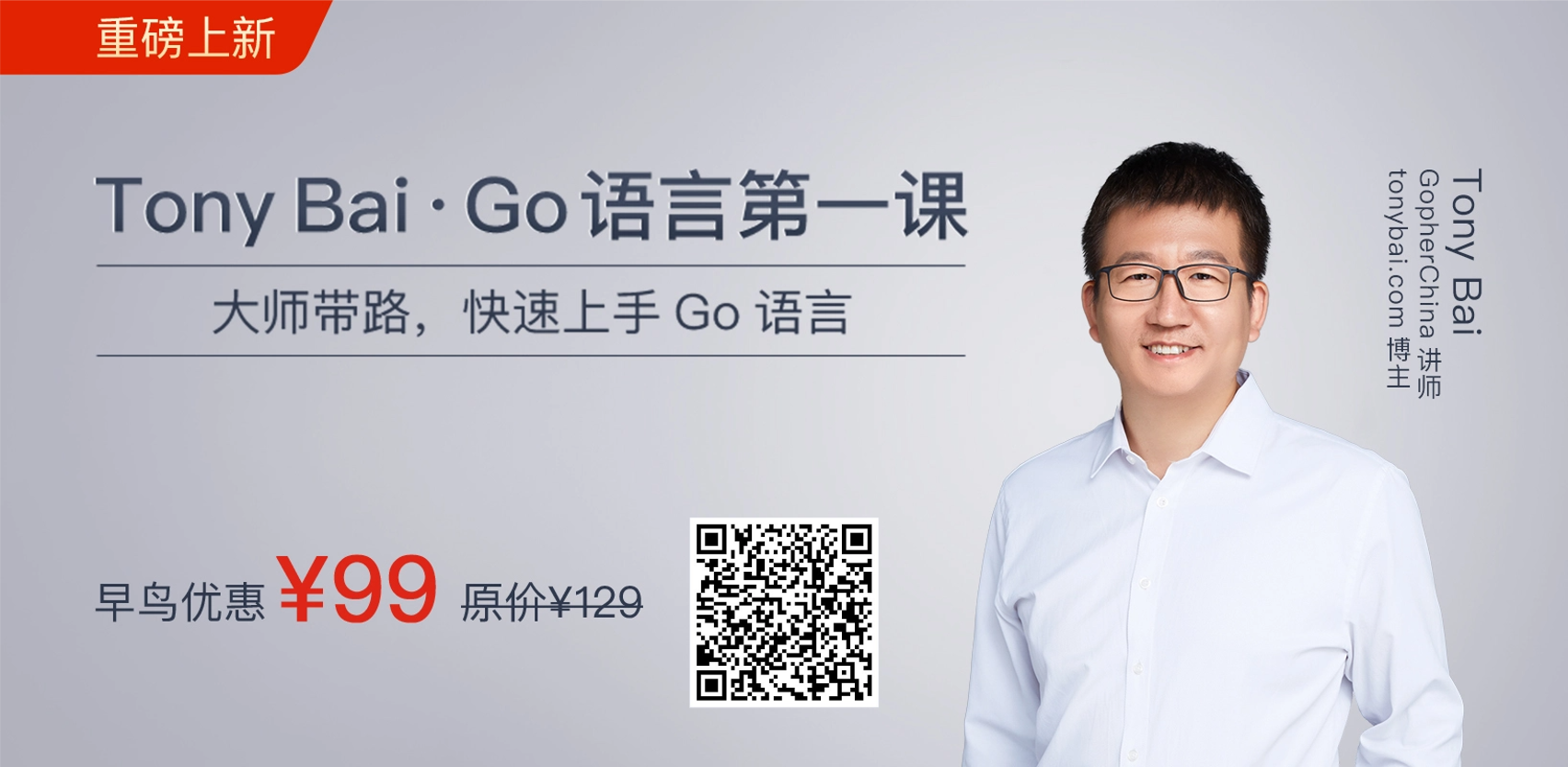
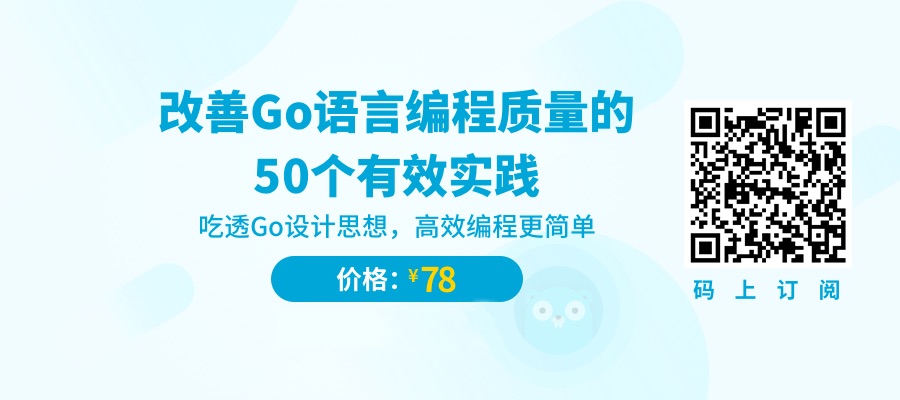
著名云主机服务厂商DigitalOcean发布最新的主机计划,入门级Droplet配置升级为:1 core CPU、1G内存、25G高速SSD,价格5$/月。有使用DigitalOcean需求的朋友,可以打开这个链接地址:https://m.do.co/c/bff6eed92687 开启你的DO主机之路。
Gopher Daily(Gopher每日新闻)归档仓库 – https://github.com/bigwhite/gopherdaily
我的联系方式:
- 微博(暂不可用):https://weibo.com/bigwhite20xx
- 微博2:https://weibo.com/u/6484441286
- 博客:tonybai.com
- github: https://github.com/bigwhite
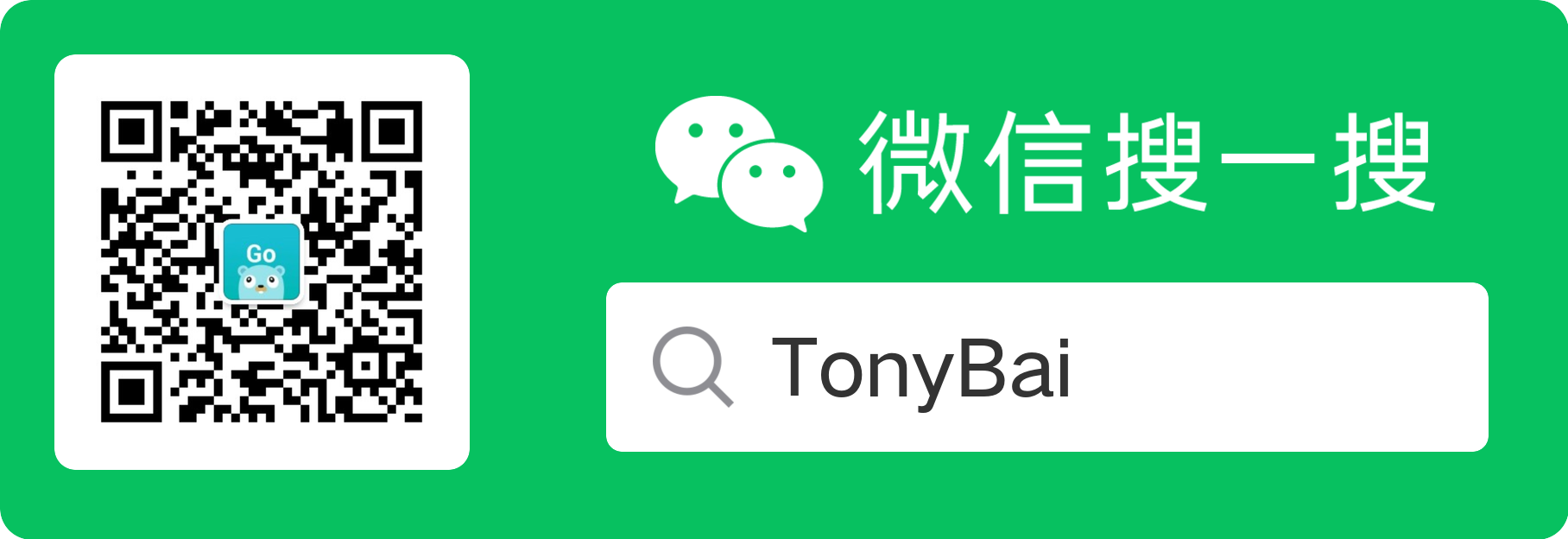
商务合作方式:撰稿、出书、培训、在线课程、合伙创业、咨询、广告合作。
© 2023, bigwhite. 版权所有.
Related posts:
Recommend
About Joyk
Aggregate valuable and interesting links.
Joyk means Joy of geeK