

OpenAI's New Tool Attempts To Explain Language Models' Behaviors - Slashdot
source link: https://slashdot.org/story/23/05/09/2133220/openais-new-tool-attempts-to-explain-language-models-behaviors
Go to the source link to view the article. You can view the picture content, updated content and better typesetting reading experience. If the link is broken, please click the button below to view the snapshot at that time.
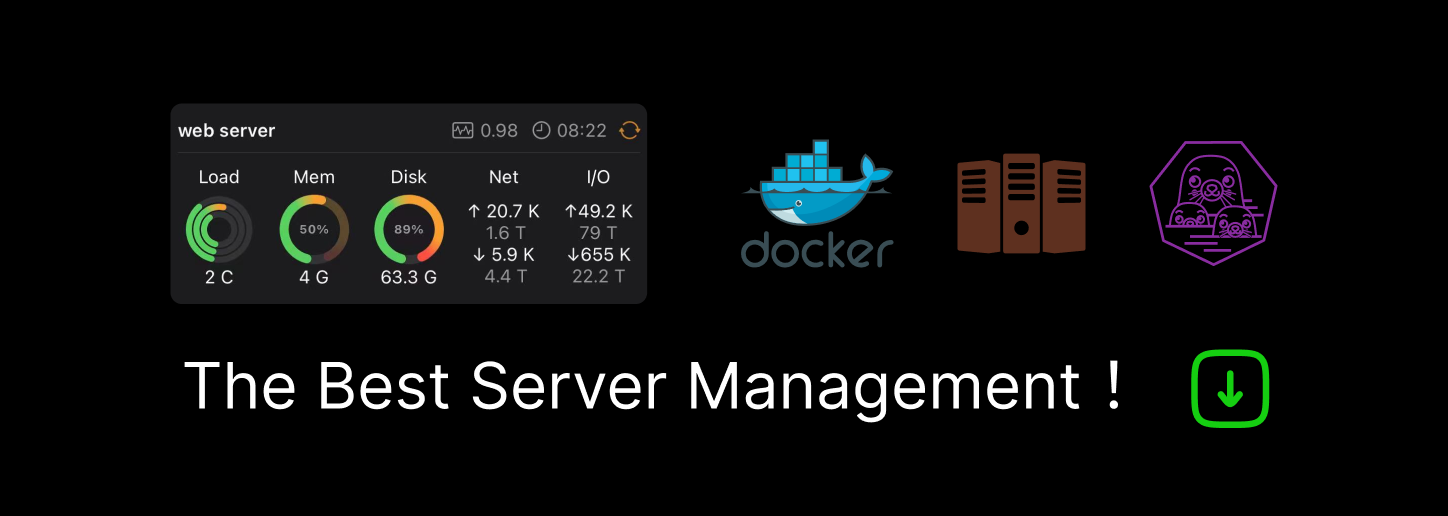
OpenAI's New Tool Attempts To Explain Language Models' Behaviors
Slashdot is powered by your submissions, so send in your scoop
binspamdupenotthebestofftopicslownewsdaystalestupid freshfunnyinsightfulinterestingmaybe offtopicflamebaittrollredundantoverrated insightfulinterestinginformativefunnyunderrated descriptive typodupeerror
Sign up for the Slashdot newsletter! or check out the new Slashdot job board to browse remote jobs or jobs in your area
To that end, OpenAI's tool uses a language model (ironically) to figure out the functions of the components of other, architecturally simpler LLMs -- specifically OpenAI's own GPT-2. How? First, a quick explainer on LLMs for background. Like the brain, they're made up of "neurons," which observe some specific pattern in text to influence what the overall model "says" next. For example, given a prompt about superheros (e.g. "Which superheros have the most useful superpowers?"), a "Marvel superhero neuron" might boost the probability the model names specific superheroes from Marvel movies. OpenAI's tool exploits this setup to break models down into their individual pieces. First, the tool runs text sequences through the model being evaluated and waits for cases where a particular neuron "activates" frequently. Next, it "shows" GPT-4, OpenAI's latest text-generating AI model, these highly active neurons and has GPT-4 generate an explanation. To determine how accurate the explanation is, the tool provides GPT-4 with text sequences and has it predict, or simulate, how the neuron would behave. In then compares the behavior of the simulated neuron with the behavior of the actual neuron.
"Using this methodology, we can basically, for every single neuron, come up with some kind of preliminary natural language explanation for what it's doing and also have a score for how how well that explanation matches the actual behavior," Jeff Wu, who leads the scalable alignment team at OpenAI, said. "We're using GPT-4 as part of the process to produce explanations of what a neuron is looking for and then score how well those explanations match the reality of what it's doing." The researchers were able to generate explanations for all 307,200 neurons in GPT-2, which they compiled in a dataset that's been released alongside the tool code. "Most of the explanations score quite poorly or don't explain that much of the behavior of the actual neuron," Wu said. "A lot of the neurons, for example, are active in a way where it's very hard to tell what's going on -- like they activate on five or six different things, but there's no discernible pattern. Sometimes there is a discernible pattern, but GPT-4 is unable to find it."
"We hope that this will open up a promising avenue to address interpretability in an automated way that others can build on and contribute to," Wu said. "The hope is that we really actually have good explanations of not just what neurons are responding to but overall, the behavior of these models -- what kinds of circuits they're computing and how certain neurons affect other neurons."
Recommend
About Joyk
Aggregate valuable and interesting links.
Joyk means Joy of geeK