

How We Built and Deployed 3 ML Models in 3 Months
source link: https://hackernoon.com/how-we-built-and-deployed-3-ml-models-in-3-months
Go to the source link to view the article. You can view the picture content, updated content and better typesetting reading experience. If the link is broken, please click the button below to view the snapshot at that time.
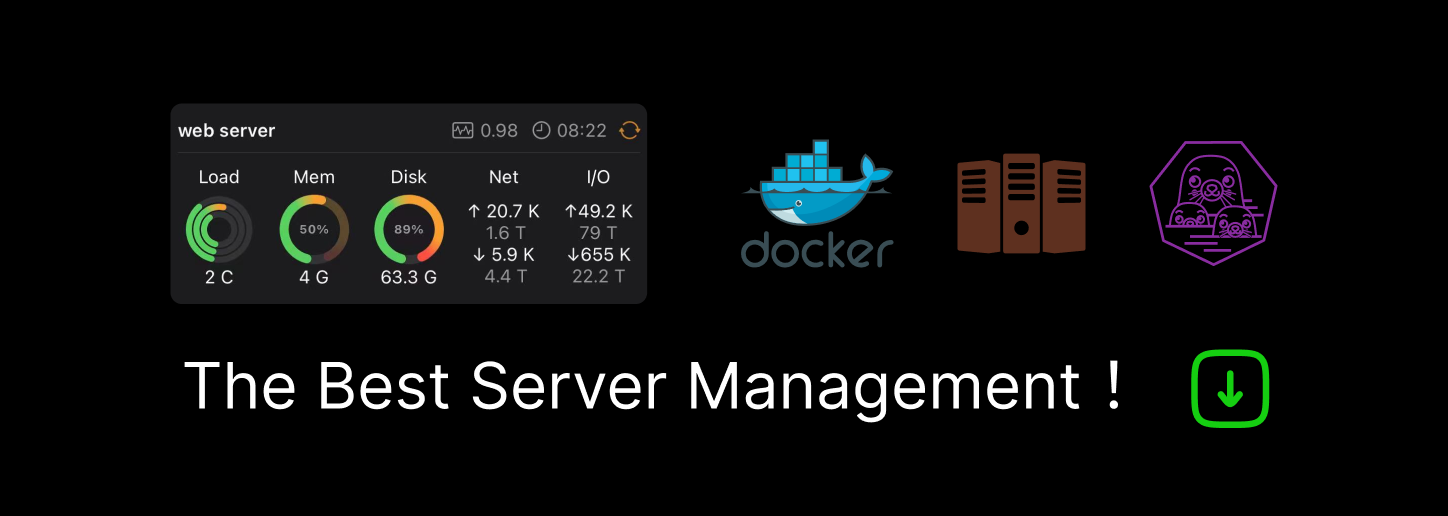
How We Built and Deployed 3 ML Models in 3 Months
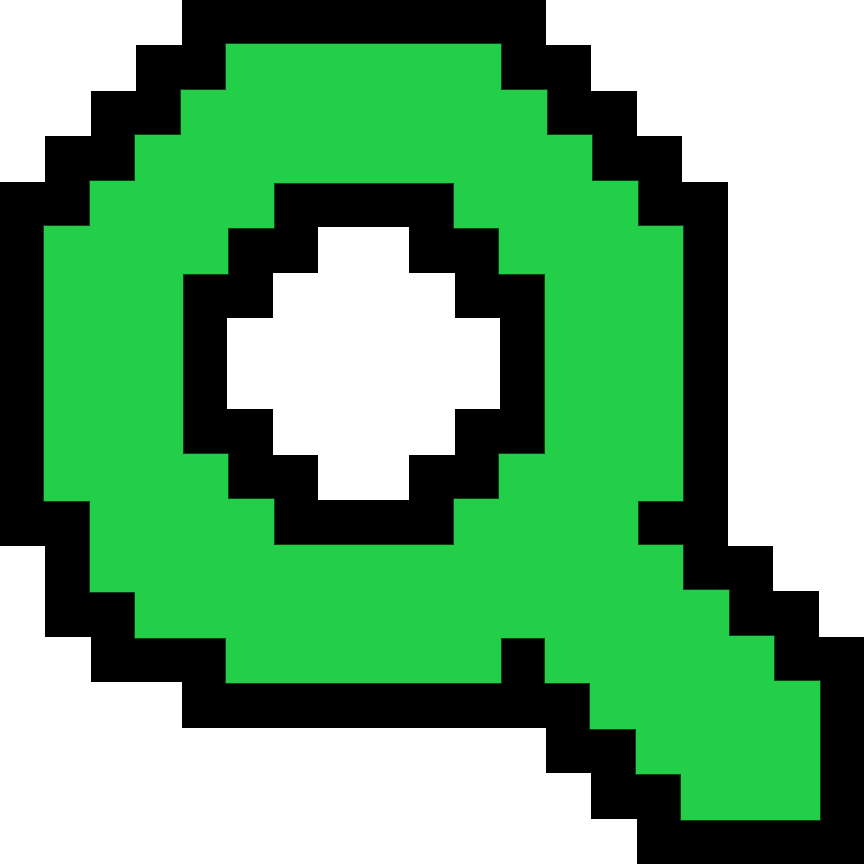
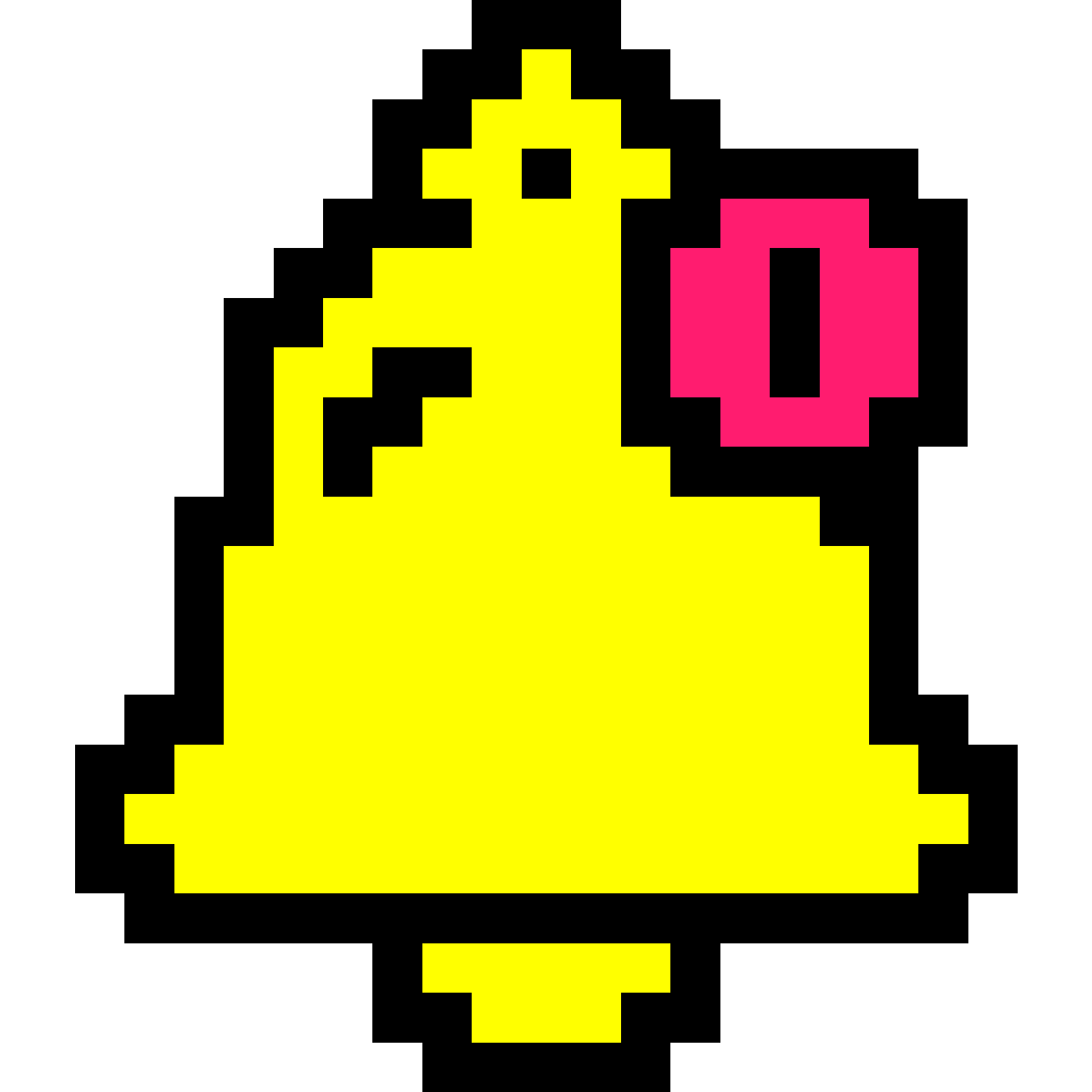
How We Built and Deployed 3 ML Models in 3 Months
2min
by @huwfulcher
Huw Fulcher
@huwfulcher
Software/MLOps Engineer, ex-Data Science Lead. Writing on mlopsnow.com to help...
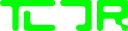
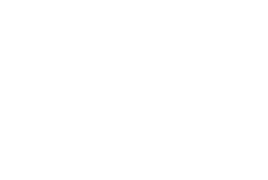



Too Long; Didn't Read
In this article, we’ll explore how my team went from having zero models and no infrastructure to deploying three production-ready models in just three months using MLOps principles. We’ll discuss the strategies we employed, the tools we used, and the lessons we learned along the way.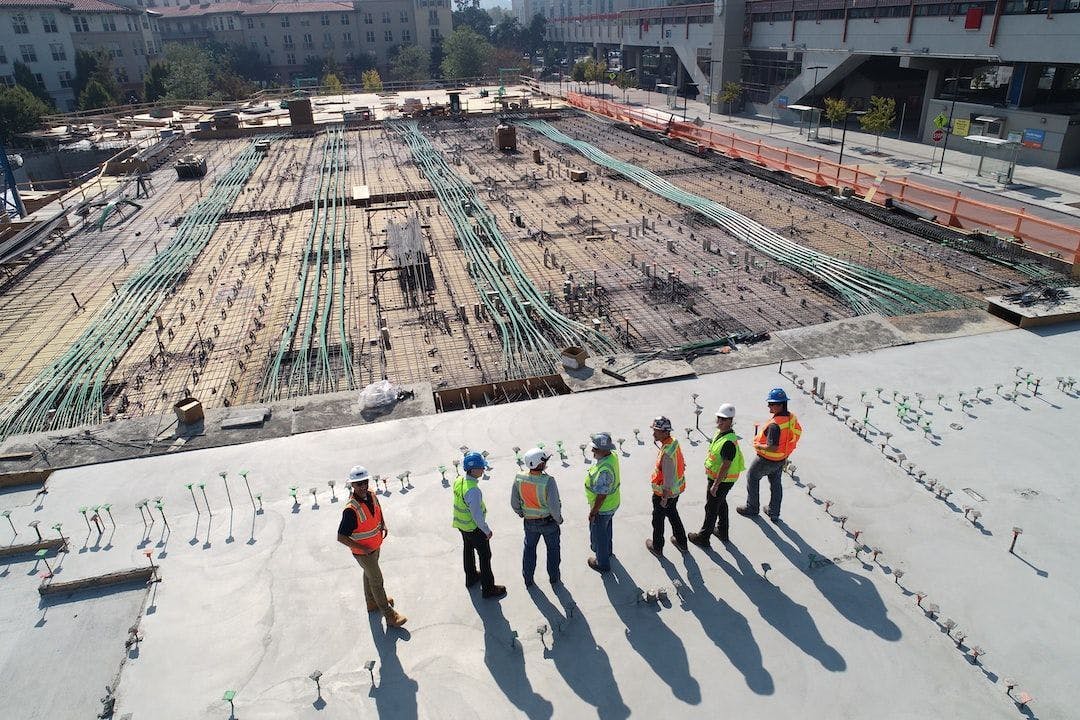
audio
element.@huwfulcher
Huw FulcherSoftware/MLOps Engineer, ex-Data Science Lead. Writing on...
Receive Stories from @huwfulcher
Machine learning operations (MLOps) is a critical component of modern data science. It streamlines the process of building, deploying, and maintaining machine learning models, making it easier for businesses to extract value from their data.
In this article, we’ll explore how my team went from having zero models and no infrastructure to deploying three production-ready models in just three months using MLOps principles. We’ll discuss the strategies we employed, the tools we used, and the lessons we learned along the way.
1. Leveraging Outside Help
As a Data Science Lead at the time, MLOps was a concept I was familiar with but had not yet put into practice. To kickstart our MLOps journey, we enlisted the help of a machine learning consultancy. This partnership allowed us to work alongside experienced professionals who guided us through the process of building a robust ML infrastructure. This safety net of knowledge was instrumental in accelerating our progress and ensuring that we built a solid foundation for our MLOps efforts.
2. Unlocking Team Productivity
One key factor that contributed to our rapid progress was the focused approach we took with our data science team. We narrowed the team’s scope to three specific models, with no deviations. All efforts, from experimentation to deployment, were directed towards these three models, which we knew would have the most significant impact on the business. By maintaining a clear focus and parking ideas for future models in a backlog, we were able to optimize our team’s productivity and drive results quickly.
3. Leveraging Existing MLOps Environments
Instead of building a custom MLOps platform from scratch, we opted to leverage existing tools and environments. We used Amazon Web Services (AWS) SageMaker, a fully managed machine learning service, along with some custom code. This decision allowed us to accelerate our time to market significantly, as we didn’t have to worry about building the critical infrastructure components ourselves. Moreover, using a well-established platform like SageMaker gave us greater confidence in the stability and reliability of our infrastructure.
4. Gaining Buy-in from the Business
Machine learning is not just for data departments – it provides value to the entire organization. To ensure a smooth transition to a model-driven approach, we communicated our plans and progress with teams across the company. By involving stakeholders from various departments and integrating their feedback into our development process, we ensured that everyone was prepared for the deployment of our models.
Conclusion
Our success in deploying three models in just three months demonstrates the power of MLOps in accelerating the development and deployment of machine learning solutions. By leveraging outside help, focusing our team’s efforts, using existing tools and environments, and obtaining buy-in from the business, we were able to rapidly create value from our data.
Featured Photo by Scott Blake on Unsplash
Also published here.
Comments
loading...Recommend
About Joyk
Aggregate valuable and interesting links.
Joyk means Joy of geeK