

GPT结合业务探索的思考二
source link: https://dreambo8563.github.io/2023/04/16/GPT%E7%BB%93%E5%90%88%E4%B8%9A%E5%8A%A1%E6%8E%A2%E7%B4%A2%E7%9A%84%E6%80%9D%E8%80%83%E4%BA%8C/
Go to the source link to view the article. You can view the picture content, updated content and better typesetting reading experience. If the link is broken, please click the button below to view the snapshot at that time.
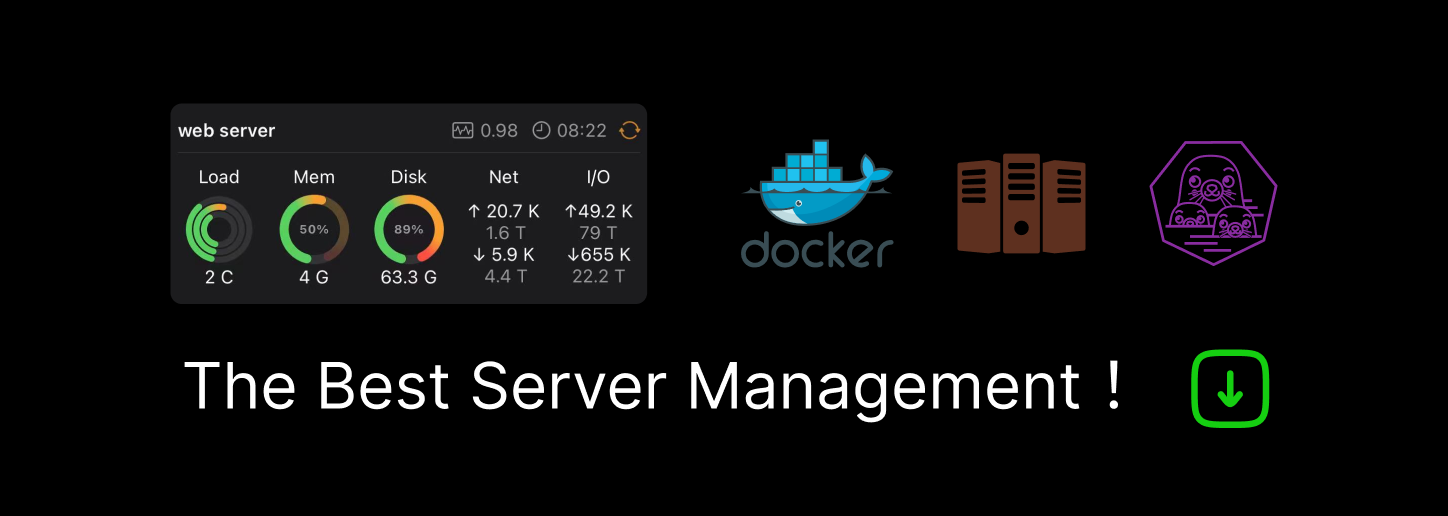
GPT结合业务探索的思考二
2023-04-16
| AI
上一篇主要是应用了 数据抽取 这个 openAI 的能力
这篇将主要记录 内容搜索 的能力的探索过程
https://beebom.com/how-train-ai-chatbot-custom-knowledge-base-chatgpt-api/
langchain
我们将借用这个探索的机会学习额外的东西
- python
- langchain
这个流程很多文章都说的很清楚, 这里没很么太多疑义
- 把自有知识库分拆成多个 chunk, 每个 chunk 可以向量化存到数据库
- 将输入(带 prompt) 也向量化
- 用输入从数据库中搜索相近的多个结果
- 将结果和原始问题 拼接, 结果作为参考资料, 让 gpt 回答
创建基础的能力
- 获取用户的输入
- 加入 prompt 并想 openAI 获取结果
这是基本流程的建立, 直接看 langchain 官网即可
https://python.langchain.com/en/latest/
开始我想试下 js 版本,但遇到了点困难, 和 openAI 打不通, 感觉是源码里打的对应的 api 地址不对造成的. 也可能我哪里没配置对. 后来索性就想顺便学点 python 也好.
毕竟在 AI 这个生态里, python 可以说是让不过去的门槛.
读取自有的知识库,并存储到向量数据库
https://python.langchain.com/en/latest/modules/indexes.html
这里的两个步骤是连续的, 因为都和存储的组件相关
- 读取文件存到数据库
- 根据输入从数据库里搜索到相似的内容
选择安装存储组件
这里我花费的时间有点多(大概是半天).
- Chroma 看到时直接放内存的,我估计会比较简单搭建,让程序跑起来, 最后发现我的 MBP 的 cpu 不合适, 安装不上而放弃
- 先看到有 redis 支持. 就拿现有的测试环境数据库尝试,发现不支持. 一直失败, 开始以为是 redis 版本不够. 后续发现应该是需要额外模块才行.
https://redis.io/docs/stack/search/ - 应该是要安装这个模块才行,还未尝试 - 又看到 ES 可以支持. 也是想直接用项目上现有的测试 ES.发现应该是版本不够. 我们是 7.9. 从提示看起码需要 8. 放弃
- 最后一个还算比较熟悉的就是 postgres 了
很多时间都花在更新 homebrew 了. homebrew 直接安装 postgres@14 这个过程还好,缺啥装啥就行了. 当然 mac 自带的 python 是 2.x. 需要先装 3.x 和对应 pip
然后需要安装 向量化插件 https://python.langchain.com/en/latest/modules/indexes/vectorstores/examples/pgvector.html
还要在 db 中执行插件命令 CREATE EXTENSION vector
基础设施基本有了, 才能把代码逻辑连上
下面解决导入的问题
这个比较顺利. 尝试了比较普遍的格式
这里没遇到明显的困难, 运行报错基本都是有些依赖包没安装
中间有些 prompt 的细节最后看代码注释就明白了, 我也尝试看了下向量化的结果,真的看不懂,非常多的维度坐标来表示一段话的核心含义
这些向量化数据库都具备搜索的 api,所以这步不用说了
导入执行成功后, 那段代码就需要注释掉, 否则每次执行都会导入一遍,数据库里有会重复信息
可以通过如下 sql 查看导入的向量化数据
SELECT * FROM information_schema.tables WHERE table_schema = 'public';
SELECT * from langchain_pg_embedding
最后看了开始的参考文章加了 gradio 这个 UI.
基本上我想探索的搜索能力都具备了
import gradio as gr
from langchain.prompts.chat import (
ChatPromptTemplate,
SystemMessagePromptTemplate,
HumanMessagePromptTemplate,
)
from langchain import LLMChain
from langchain.chat_models import ChatOpenAI
from langchain.docstore.document import Document
from langchain.vectorstores.pgvector import PGVector
from langchain.text_splitter import CharacterTextSplitter
from langchain.embeddings.openai import OpenAIEmbeddings
from typing import List, Tuple
import os
from dotenv import load_dotenv
load_dotenv()
def chatbot(input_text):
# load txt
# from langchain.document_loaders import TextLoader
# loader = TextLoader('./zhiyin.txt')
# documents = loader.load()
# load pdf
# from langchain.document_loaders import PDFMinerLoader
# loader = PDFMinerLoader("./1.pdf")
# documents = loader.load()
documents = []
text_splitter = CharacterTextSplitter(
chunk_size=500, separator="\n", chunk_overlap=0)
docs = text_splitter.split_documents(documents)
embeddings = OpenAIEmbeddings() # type: ignore
# PGVector needs the connection string to the database.
# We will load it from the environment variables.
CONNECTION_STRING = PGVector.connection_string_from_db_params(
driver=os.environ.get("PGVECTOR_DRIVER", "psycopg2"),
host=os.environ.get("PGVECTOR_HOST", "localhost"),
port=int(os.environ.get("PGVECTOR_PORT", "5432")),
database=os.environ.get("PGVECTOR_DATABASE", "postgres"),
user=os.environ.get("PGVECTOR_USER", "vincent"),
password=os.environ.get("PGVECTOR_PASSWORD", ""),
)
# The PGVector Module will try to create a table with the name of the collection. So, make sure that the collection name is unique and the user has the
# permission to create a table.
# load doc into gp and create embedding
db = PGVector.from_documents(
embedding=embeddings,
documents=docs,
collection_name="kaoqin",
connection_string=CONNECTION_STRING,
)
query = input_text
docs_with_score: List[Tuple[Document, float]] = db.similarity_search_with_score(query, 10)
dict = {}
resultStr: str = ""
print("*"*80)
for doc, score in docs_with_score:
if dict.get(score) == None:
# remove the same score content
dict[score] = doc.page_content
print("score",score)
print("page_content",doc.page_content)
resultStr += doc.page_content
# compose all text
print("*"*80)
system_message_prompt = SystemMessagePromptTemplate.from_template(
"你将作为一个问答知识库,根据提出的问题给出回答和相关参考信息.回答字数不超过 1000 字符")
human_template = """
参考信息:{reference}
问题是:{question}
"""
human_message_prompt = HumanMessagePromptTemplate.from_template(
human_template)
chat_prompt = ChatPromptTemplate.from_messages(
[system_message_prompt, human_message_prompt])
chat = ChatOpenAI(
temperature=0.5, model_name="gpt-3.5-turbo") # type: ignore
chain = LLMChain(llm=chat, prompt=chat_prompt)
# compose question and reference and send to gpt
print("question:", query)
# print("chatGPT:", chain.run(reference=resultStr[0:2000], question=query))
answer = chain.run(reference=resultStr[0:2000], question=query)
print("answer:" ,answer)
return answer
iface = gr.Interface(fn=chatbot,
inputs=gr.components.Textbox(
lines=14, label="Enter your text"),
outputs=gr.components.Textbox(
lines=14),
title="Custom-trained AI Chatbot")
iface.launch(share=False)
Recommend
About Joyk
Aggregate valuable and interesting links.
Joyk means Joy of geeK