

Python 代码性能分析
source link: https://xujinzh.github.io/2023/04/19/python-profile/
Go to the source link to view the article. You can view the picture content, updated content and better typesetting reading experience. If the link is broken, please click the button below to view the snapshot at that time.
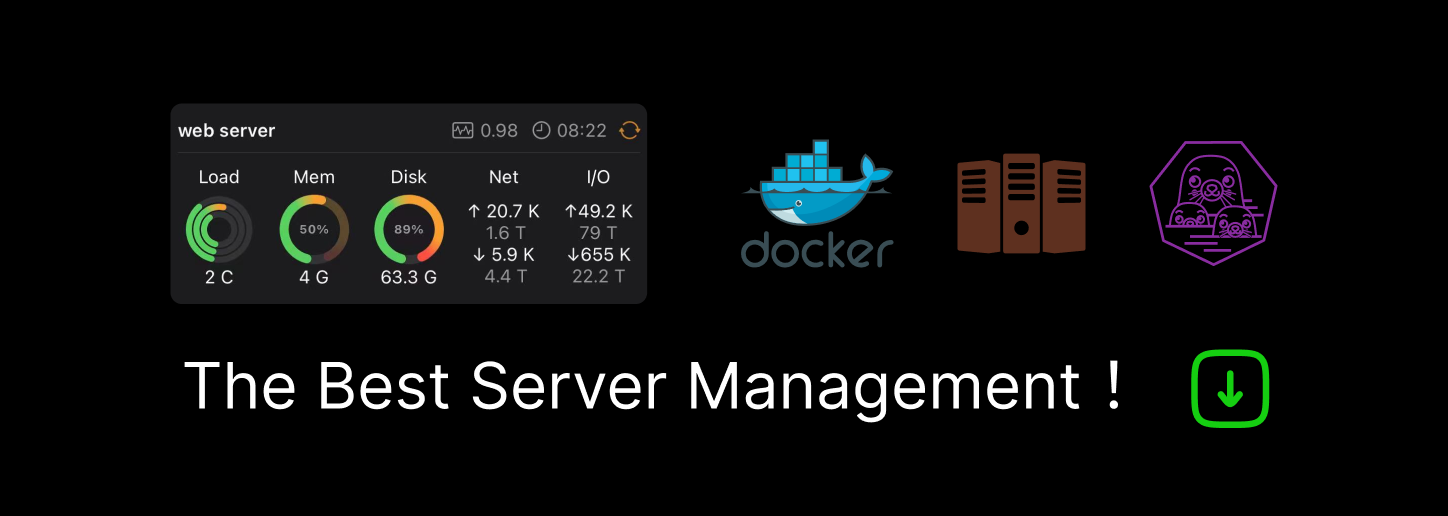
Python 代码性能分析
Python 是一门非常优秀的面向对象的解释性语言,代码编写快且易读(但从代码字面意义上)。如果非要挑出一个缺点,那就是代码的执行速度相对于 C、Java、Golang 等语言较慢,不过在 3.11 版的 Python 运行速度已经进行大幅提高。但是,对于 Python 代码的性能分析仍然不可或缺,这有助于找出耗时的代码部分,通过优化能够加速程序运行。本篇介绍一些 Python 代码性能分析的方法。
代码的性能分析跟代码执行时间密切相关,只不过它关注的是耗时的位置。默认的 Python 性能分析工具是 cProfile 模块,它在执行一个程序或代码块时,会记录各函数所耗费的时间。但它不是转为 Python 设计的。
cProfile 一般是在命令行上使用的,它将执行整个程序然后输出各函数的执行时间。我们首先编写一个 Python 模块 py_performance.py
:
import numpy as np
from numpy.linalg import eigvals
np.random.seed(33)
def run_experiment(niter=100):
K = 100
results = []
for _ in range(niter):
mat = np.random.randn(K, K)
max_eigenvalue = np.abs(eigvals(mat)).max()
results.append(max_eigenvalue)
return results
if __name__ == "__main__":
some_results = run_experiment()
print(f"最大特征值: {np.max(some_results)}")
执行性能分析:
python -m cProfile py_performance.py
结果大致是下面:
Largest one we saw: 11.375894329102476
80055 function calls (77991 primitive calls) in 1.101 seconds
Ordered by: standard name
ncalls tottime percall cumtime percall filename:lineno(function)
100 0.000 0.000 0.002 0.000 <__array_function__ internals>:177(all)
1 0.000 0.000 0.000 0.000 <__array_function__ internals>:177(amax)
1 0.000 0.000 0.000 0.000 <__array_function__ internals>:177(concatenate)
1 0.000 0.000 0.000 0.000 <__array_function__ internals>:177(copyto)
100 0.000 0.000 0.592 0.006 <__array_function__ internals>:177(eigvals)
149/1 0.001 0.000 0.454 0.454 <frozen importlib._bootstrap>:1002(_find_and_load)
180/16 0.001 0.000 0.435 0.027 <frozen importlib._bootstrap>:1033(_handle_fromlist)
452 0.001 0.000 0.002 0.000 <frozen importlib._bootstrap>:112(release)
149 0.000 0.000 0.000 0.000 <frozen importlib._bootstrap>:152(__init__)
149 0.000 0.000 0.003 0.000 <frozen importlib._bootstrap>:156(__enter__)
149 0.000 0.000 0.001 0.000 <frozen importlib._bootstrap>:160(__exit__)
452 0.002 0.000 0.003 0.000 <frozen importlib._bootstrap>:166(_get_module_lock)
单从上面的日志很难发现最耗时的地方在哪里,常用 -s 标志对某一列进行排序,如 cumtime:
Largest one we saw: 11.375894329102476
80055 function calls (77991 primitive calls) in 0.743 seconds
Ordered by: cumulative time
ncalls tottime percall cumtime percall filename:lineno(function)
110/1 0.000 0.000 0.743 0.743 {built-in method builtins.exec}
1 0.000 0.000 0.743 0.743 py_performance.py:1(<module>)
1 0.001 0.001 0.621 0.621 py_performance.py:5(run_experiment)
100 0.000 0.000 0.567 0.006 <__array_function__ internals>:177(eigvals)
203/103 0.000 0.000 0.567 0.006 {built-in method numpy.core._multiarray_umath.implement_array_function}
100 0.562 0.006 0.567 0.006 linalg.py:976(eigvals)
13 0.001 0.000 0.259 0.020 __init__.py:1(<module>)
149/1 0.001 0.000 0.122 0.122 <frozen importlib._bootstrap>:1002(_find_and_load)
149/1 0.001 0.000 0.122 0.122 <frozen importlib._bootstrap>:967(_find_and_load_unlocked)
138/1 0.001 0.000 0.122 0.122 <frozen importlib._bootstrap>:659(_load_unlocked)
109/1 0.000 0.000 0.122 0.122 <frozen importlib._bootstrap_external>:844(exec_module)
216/1 0.000 0.000 0.122 0.122 <frozen importlib._bootstrap>:220(_call_with_frames_removed)
180/16 0.001 0.000 0.118 0.007 <frozen importlib._bootstrap>:1033(_handle_fromlist)
362/8 0.001 0.000 0.117 0.015 {built-in method builtins.__import__}
100 0.053 0.001 0.053 0.001 {method 'randn' of 'numpy.random.mtrand.RandomState' objects}
注意:如果一个函数调用了别的函数,计时器是不会停下来重新计时的。
除了上面命令行的方式外,cProfile 还提供了编程式的分析代码块的性能方法,IPython 提供了方便的接口,如 %prun
和 %run -p
.
基本性能分析 %prun 和 %run -p
该魔法函数可以直接在 IPython Jupyter notebook 中使用,
from pyscripts.py_performance import run_experiment
# 这里为了节省空间,只打印7行
%prun -l 7 -s cumulative run_experiment()
结果如下:
4004 function calls (3904 primitive calls) in 0.603 seconds
Ordered by: cumulative time
List reduced from 35 to 7 due to restriction <7>
ncalls tottime percall cumtime percall filename:lineno(function)
1 0.000 0.000 0.603 0.603 {built-in method builtins.exec}
1 0.000 0.000 0.603 0.603 <string>:1(<module>)
1 0.001 0.001 0.603 0.603 py_performance.py:5(run_experiment)
100 0.000 0.000 0.552 0.006 <__array_function__ internals>:177(eigvals)
200/100 0.000 0.000 0.552 0.006 {built-in method numpy.core._multiarray_umath.implement_array_function}
100 0.547 0.005 0.552 0.006 linalg.py:976(eigvals)
100 0.049 0.000 0.049 0.000 {method 'randn' of 'numpy.random.mtrand.RandomState' objects}
%run -p -l 7 -s cumulative pyscripts/py_performance.py
结果如下:
Largest one we saw: 11.375894329102476
4163 function calls (4062 primitive calls) in 0.584 seconds
Ordered by: cumulative time
List reduced from 106 to 7 due to restriction <7>
ncalls tottime percall cumtime percall filename:lineno(function)
2/1 0.000 0.000 0.584 0.584 {built-in method builtins.exec}
1 0.000 0.000 0.584 0.584 <string>:1(<module>)
1 0.000 0.000 0.584 0.584 interactiveshell.py:2774(safe_execfile)
1 0.000 0.000 0.583 0.583 py3compat.py:51(execfile)
1 0.000 0.000 0.582 0.582 py_performance.py:1(<module>)
1 0.001 0.001 0.581 0.581 py_performance.py:5(run_experiment)
100 0.000 0.000 0.532 0.005 <__array_function__ internals>:177(eigvals)
逐行分析函数性能 %lprun
逐行分析函数性能需要第三方模块 line_profiler
,安装方法如下:
pip install line_profiler
安装后,可以编辑 ~/.ipython/profile_default/ipython_config.py
,增加如下内容:
c.TerminalIPythonApp.extensions = [
'line_profiler',
]
此时,可以在 IPython 中使用:
In [1]: from pyscripts.py_performance import run_experiment
In [2]: %lprun -f run_experiment run_experiment()
Timer unit: 1e-09 s
Total time: 0.997021 s
File: /home/jinzhongxu/MEGA/py/nb/bs/pyscripts/py_performance.py
Function: run_experiment at line 5
Line # Hits Time Per Hit % Time Line Contents
==============================================================
5 def run_experiment(niter=100):
6 1 1530.0 1530.0 0.0 K = 100
7 1 421.0 421.0 0.0 results = []
8 100 57943.0 579.4 0.0 for _ in range(niter):
9 100 66120221.0 661202.2 6.6 mat = np.random.randn(K, K)
10 100 930721489.0 9307214.9 93.4 max_eigenvalue = np.abs(eigvals(mat)).max()
11 100 118745.0 1187.5 0.0 results.append(max_eigenvalue)
12 1 321.0 321.0 0.0 return results
%lprun
的通用语法为:
%lprun -f func1 -f func2 func(x, y)
在 Jupyter notebook 中使用:
%load_ext line_profiler
from pyscripts.py_performance import run_experiment
%lprun -f run_experiment run_experiment()
结果如下:
Timer unit: 1e-09 s
Total time: 0.58282 s
File: /home/jinzhongxu/MEGA/py/nb/bs/pyscripts/py_performance.py
Function: run_experiment at line 5
Line # Hits Time Per Hit % Time Line Contents
==============================================================
5 def run_experiment(niter=100):
6 1 4160.0 4160.0 0.0 K = 100
7 1 952.0 952.0 0.0 results = []
8 100 43829.0 438.3 0.0 for _ in range(niter):
9 100 46188517.0 461885.2 7.9 mat = np.random.randn(K, K)
10 100 536499670.0 5364996.7 92.1 max_eigenvalue = np.abs(eigvals(mat)).max()
11 100 82394.0 823.9 0.0 results.append(max_eigenvalue)
12 1 192.0 192.0 0.0 return results
Recommend
About Joyk
Aggregate valuable and interesting links.
Joyk means Joy of geeK