

What Does a Deep Learning Architect at NVIDIA Do? (Video Interview)
source link: https://hackernoon.com/what-is-a-deep-learning-architect-at-nvidia-video-interview
Go to the source link to view the article. You can view the picture content, updated content and better typesetting reading experience. If the link is broken, please click the button below to view the snapshot at that time.
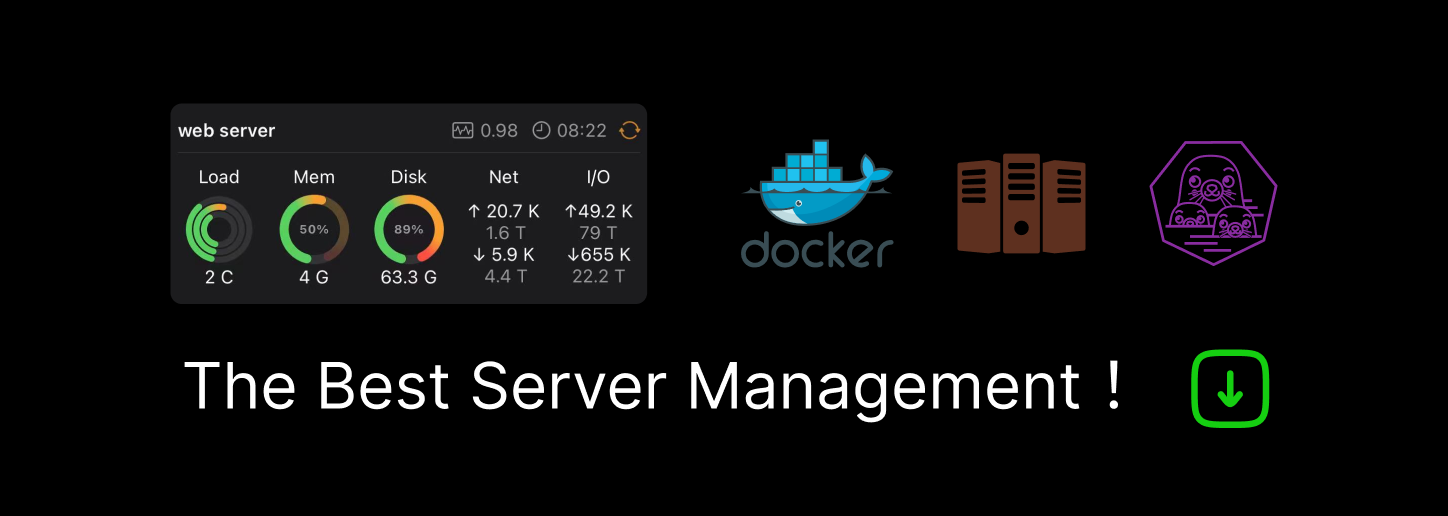
What Does a Deep Learning Architect at NVIDIA Do? (Video Interview)
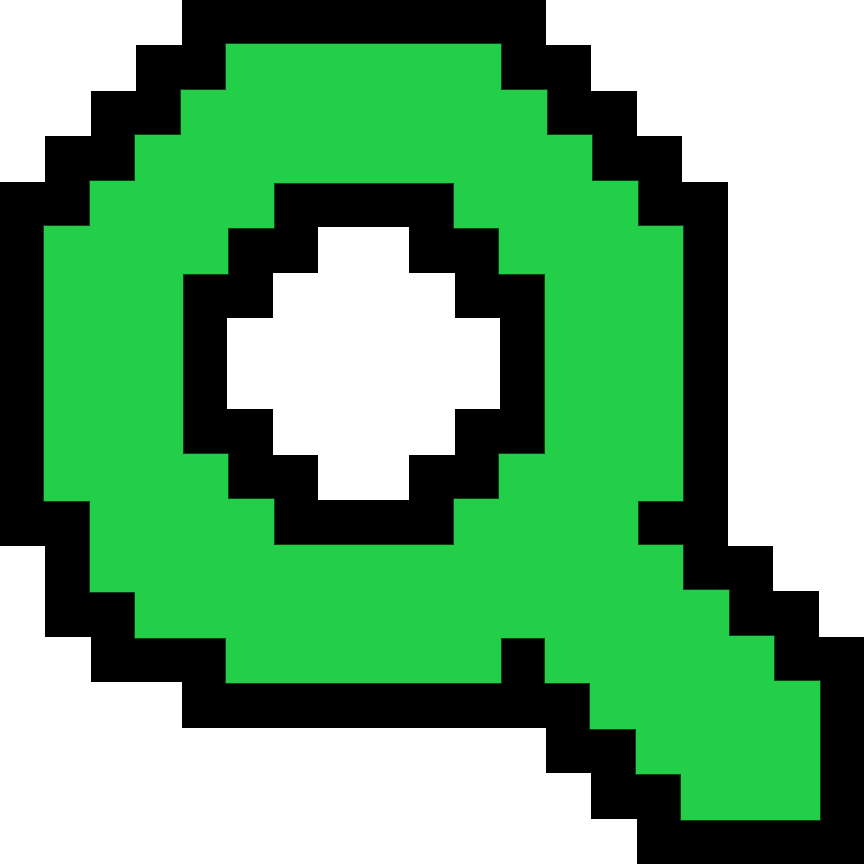
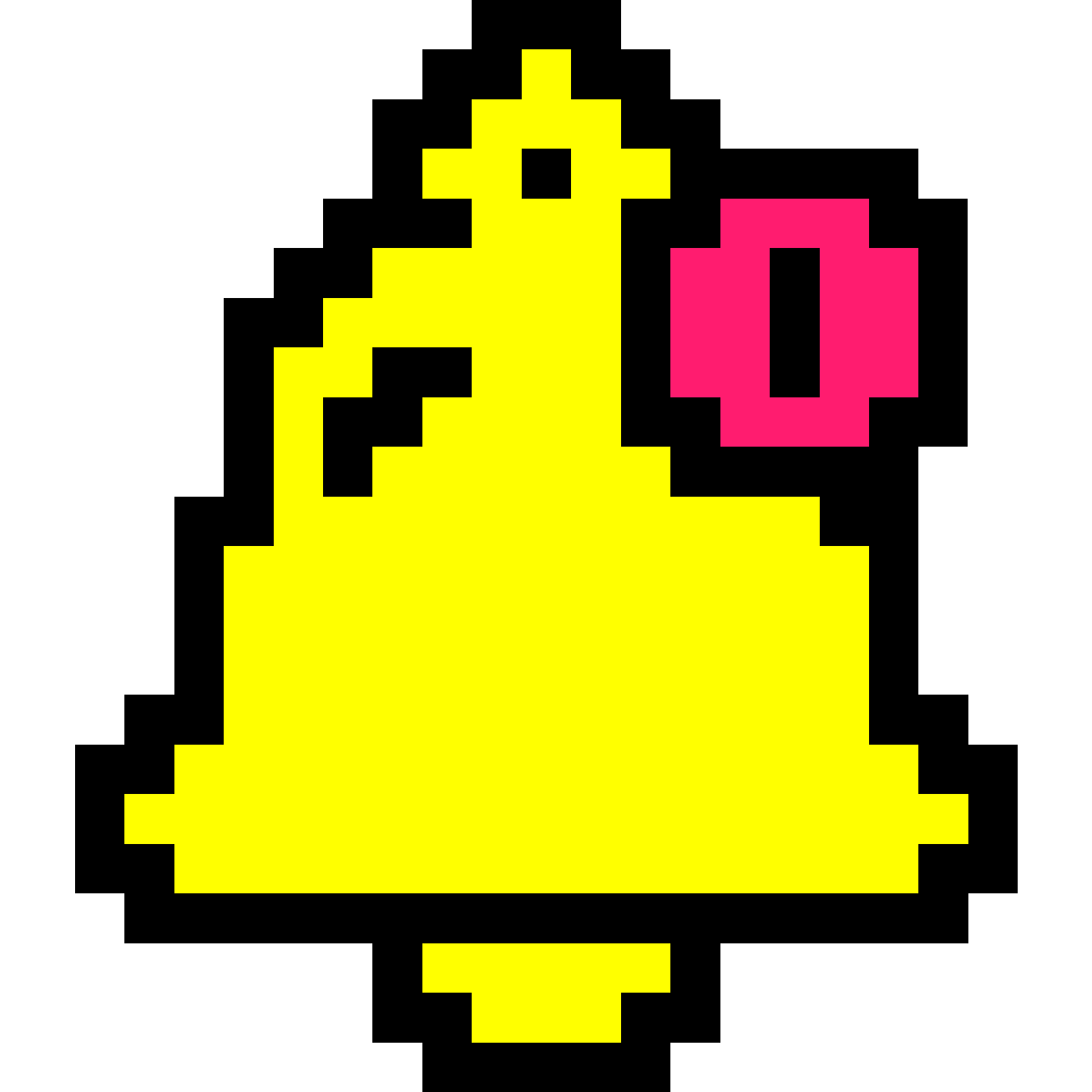
What Does a Deep Learning Architect at NVIDIA Do? (Video Interview)
1 min
by @whatsai
Louis Bouchard
@whatsai
I explain Artificial Intelligence terms and news to non-experts.
Too Long; Didn't Read
Adam Grzywaczewski, a senior deep learning architect at NVIDIA, is interviewed in this podcast.
He obtained a Ph.D. in information retrieval systems in 2013, before AI was trendy. With over 6 years of experience at NVIDIA, Adam has helped several companies scale their online models and is well-versed in NLP.
The interview covers topics such as Adam's Ph.D. research, the deep learning architect role, working at NVIDIA, his favorite tools, the challenges with the scaling model, and more. Gain insights from an NLP and scaling model expert.
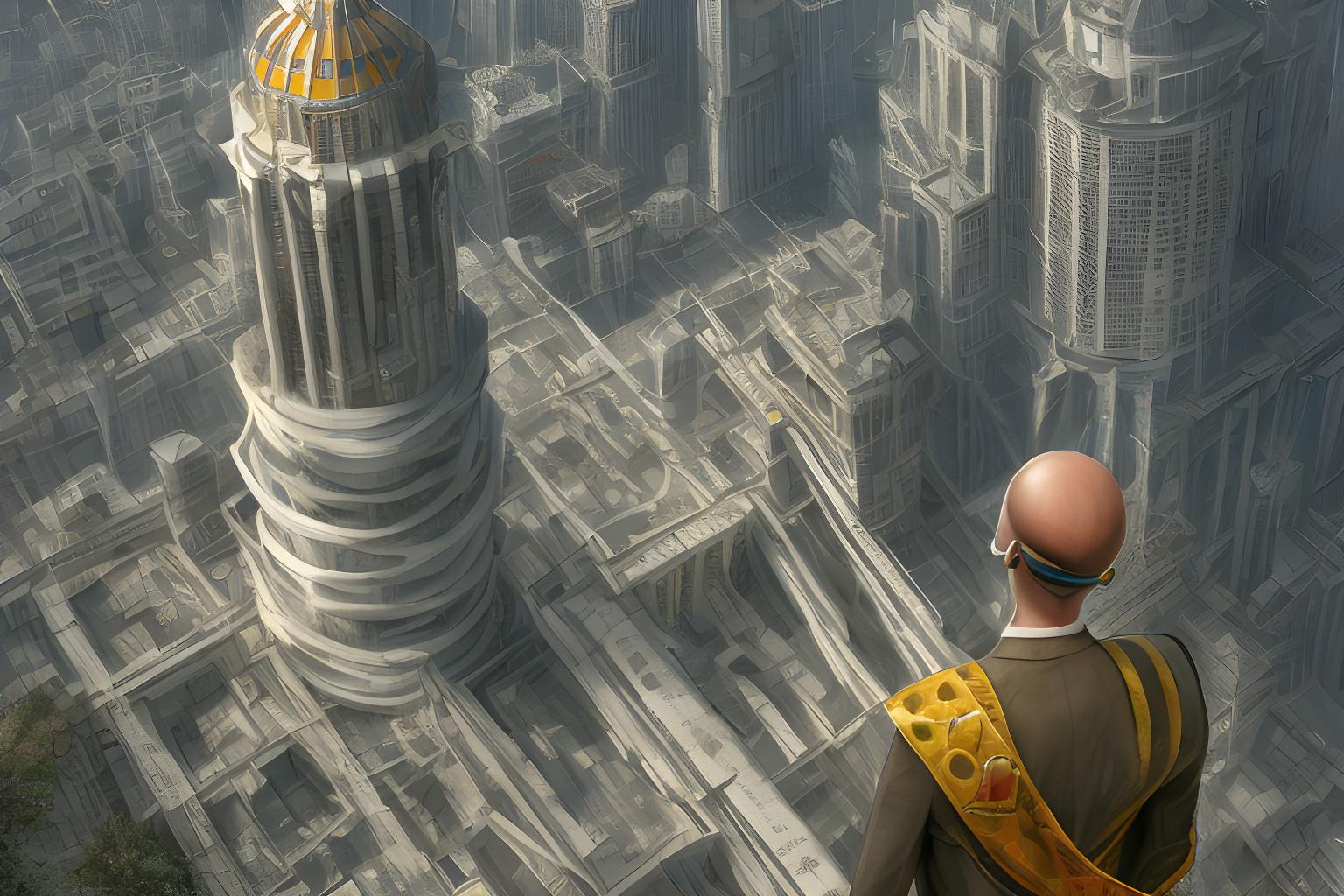
audio
element.@whatsai
Louis BouchardI explain Artificial Intelligence terms and news to non-e...
Adam Grzywaczewski, a senior deep learning architect at NVIDIA, is interviewed in this podcast.
He obtained a Ph.D. in information retrieval systems in 2013, before AI was trendy. With over 6 years of experience at NVIDIA, Adam has helped several companies scale their online models and is well-versed in NLP.
The interview covers topics such as Adam's Ph.D. research, the deep learning architect role, working at NVIDIA, his favorite tools, the challenges with the scaling model, and more. Gain insights from an NLP and scaling model expert.
Watch the Video
As a final installment in the NVIDIA partnership interview series, this podcast also includes a giveaway for an RTX 4080 GPU.
foreign
[Music]
ski deep learning architect at Nvidia
for six years before Nvidia Adam did a
PhD in information retrieval systems
which ended in 2013 and then worked as a
research engineer at Jaguar Land Rover
this interview is the third and last
interview in partnership with Nvidia and
the GTC event here's your last chance to
win an RTX 4080 you just have to attend
the free GTC event take a screenshot and
send it to me you will see that there
are a lot of incredibly interesting
talks including the ones that we'll
discuss in this interview I hope you
enjoy it
if you could go over your back
background I've seen that you've done a
PhD and worked as a resource scientist
and now at Nvidia so I would love a few
good like go back a few years and into
especially the academics background and
then how you transition upward into
Nvidia sure so you know life is a
journey in a sense and many things they
happen by accident so in on University
when I still in Poland we have been
toying with this idea with my colleague
to to to to start a business focusing on
finding tenders I used to live in this
like large metropolitan area and and and
with a lot of different cities everyone
with a different tender process so this
is how I started looking at the problem
of information retrieval that bit didn't
work out but on the back of it at some
point of time I've submitted an
application for funding of a PhD focused
on information she was a process of
finding information not necessarily in
the internet but and and and and
funnily enough despite the fact that
statistically I didn't have very high
chances someone liked it
with that particular application was
rejected but one of the reviewers
suggested that I submitted to a
different funding body and that was
accepted and funnily enough this is how
I started doing High PhD focused on you
know Finding information I specialized
in the process of supporting software
engineers in software development
process so many of you
most likely heard about Visual Studio
the the programming interface
from from Microsoft and right now
Microsoft has this thing called codex so
effectively my my PhD was focused on
building something like that obviously
not with neural networks but that was in
the the time for neural networks but
much more traditional approaches but
effectively we've been doing that we've
been trying to you know crawl various
online code repositories and inline
provide code recommendation that would
go beyond what was then intelli sense
and when was this
all right when was it I think I started
my PhD in what was it 2011.
yes so definitely a time before no
neural networks were understood but at
that point of time no one perceived them
as something that
can work particularly well and and and
like computation didn't exist to to
train anything meaningful so during my
PhD I was you know dealing with Much
More Much More conventional algorithm
related allocation and also spent a lot
of time looking at human behavior of
search so opportunistic programming so
how people actually formulate the Search
terms so that's that and funnily enough
when I was finishing my PhD even before
I finished it turned out because I used
to do it in in the Midlands that nearby
Jaguar Land Rover was just kicking off a
a part of the research Department
focusing on telematics so connected car
and they needed people that understand
machine learning and like you know that
I I I had a conversation that sounded
interesting
so after even yeah right after
submitting my reviews to PhD even before
the defense of the the physics itself I
think I moved to jaguarantro for
research and then then we've been doing
a lot of different things for those that
are interested just go to YouTube and
type self learning car jagran Rover that
was on the project that I helped to to
shape I wasn't working on it myself it
was a very large group but that was one
of the bigger things that I was focusing
on and roll out of telematics you know
and from there it was just a fairly
organic Journey part of those projects
involved neural networks coincidentally
it wasn't yeah okay super aware life
decision at that point of time it wasn't
obvious that neural networks will
actually work as well as they will but
we've looked at Andrew engs in in
baidu's work on automatic speech
recognition this is something that was
needed automatic speech recognition in
the car is it's complicated you have a
lot of background noise
so we've looked at that we've started to
look at reproducing some of that work
and this is how I CL Beyond just
traditional machine learning background
created a bit of background in
in automotive and it it so happened that
uh Nvidia recruiter was searching for
people he noticed the profile on like
then reached out and after I don't know
how many conversations and I think the
process like took nine months because it
wasn't that I've applied and went for
more interview processes immediately
like there was a bit of a back and forth
I joined Nvidia in 2017 and and started
to help with at that time predominantly
also Automotive so working a lot with
oems so car manufacturers and tier was
tier one suppliers
predominantly focusing on perception for
the self-driving curve and trying to
help them
Define the process of training
perception algorithms
which was at that point of time super
difficult like you have to appreciate
that in 2017 a lot of things that we
take for granted right now didn't work
yes so a human kind didn't know how to
scale neural networks we just didn't we
and and that that works in many
different ways we didn't know how to
make them deeper because they would
explode and not converge so for those of
you that don't believe me just
look at some older papers so for example
um what was the name of the like first
I think in Inception it it you'll notice
that it has those like multiple losses
this one at the top but then also
someone decides that's kind of so some
tricks to stabilize the training process
and we definitely didn't know how to
chain well in data parallel way with
large batch sizes not to mention that
the tools didn't exist this is like
horovot it was that wasn't something
that was available and so so there was
quite a lot of work around that also
understanding of Hardware just started
to form formulate and we had to discover
a lot of things that are obvious today
and and that that's the journey so on
the back of that I've I've learned how
to scale neural networks so when natural
language processing
like this new Revolution started with
GPT and birth it became obvious that
unsupervised training has a chance of
working but for that we'll need very
large models very large data sets focus
on that this is how I am right now where
I am the journey basically you were
already trying to scale the models that
existed in the days it's just that the
hardware didn't allow you know like no
one knew how to do it yeah so so that
knowledge didn't exist I very
distinctively remember in Europe's 2017
in December I've attended that and there
was a workshop on a AI at HPC scale no
one knew you know there were people from
Google Facebook no one knew how to scale
those models it wasn't obvious what to
do when batch size exceeds a certain
threshold very bad things happen to
optimization if you do that
is is is I think one said that France
don't allow friends to train with large
bar sizes that it's good for their
mental health and and that was true at
that point of time now obviously
if you train with extremely logical
sizes but
so it was definitely a good like you
started at the right time since you you
already had quite a lot of experience in
the field before it it got so so much
hype like in the past since 2018 let's
see let's see you know it's you say luck
but it's so luck is a big part of life
yes but you always look at the decisions
ahead of you you look at them critically
and you try to make a good decision at
that point of time and you know those
those kind of changes that I've made
seem logical
they could have ended up being a mistake
but fortunately they weren't but and for
did you really want to do a PhD or was
this just to to work on the the project
you had in mind how many people do you
know that I just went straight out of
University and know what what does it
even mean like what does it mean to do a
PhD and then have an academic career
that's a that's a super abstract thing
maybe if you have parents and that are
that are you know publishing for a
living but you know my father is a was a
minor my human story in a railway so I
clearly didn't have that understanding
you know it sounded like a good idea at
the time and the fact that I managed to
win in you know a pot of money to
so so pay for it and sustain me for a
period of time helped a lot with the
decision
but did you already have an idea in mind
of like right now for example in
artificial intelligence a lot of people
think that a PhD might be required to
get a good job and so like they are just
aiming for a title
so think about it like you're an
employer yes you need to hire someone
you get 500 CDs you need to choose
somehow who you're going to interview
you cannot interview everyone it's just
not physically possible you cannot even
interview a large group of people
because every person that you're going
to interview that's at least I would say
two three hours of work if you want to
do it well prepare now let's think about
it so you really have to be you know
quite selective at the get-go and you
need to use some heuristics and you get
a CV so what can you look what can you
look at
evidence of you know in evidence that
would suggest that that person can do
their job PhD can be one of them and if
you don't have a PhD and you're a young
person how else would you prove yourself
if you know achieved something published
a paper contributed substantially to an
to an open source project and you know
PhD is not strictly required but you
need to start somehow and PhD is a in a
sense an easy way because it's super
prescribed you go to the university you
follow a program and typically if you
don't you know make some mistakes of
have a lot of bad luck at the end of it
you'll have a PhD you would have
contributed in a bunch of projects you
have published a bunch of papers it
achieves something that you can then
through which you can describe your
your skills if you have some other way
of doing it and there are people that do
that amazing but gaining the same level
of experience
without going through like four-year PHD
programs is sometimes challenging but we
did hire a group of amazing people into
into my brother Team without phds
because they kind of proved that they
can can do the work they need to be
doing
and when you see you hired were you the
one of the people making decisions
looking at the CD or just looking at the
the profile and deciding so so I had
either personally into my team and prior
to having a team that I have supported
hiring so I've interviewed and I made
notes it's a critical assessment of
their capabilities
in that case may I ask how are you
assessing their capabilities first
before the interview but also during the
interview for example when you say that
there are different ways of of proving
that you can do the work the PHD is is
one way but what would be the other ways
that you you've seen or that you are
looking for like the the most obvious
thing but you'd be surprised how few
people do it is to actually read the job
description
I'm not kidding
me a lot of people will send a genetic
CV without any consideration to what is
it that the employer is looking for So
reading the job description helps and
then taking the next step and after
reading the job description tailoring
your CV so that you're answering the
questions via lcv that needs to be
answered so okay the the employer said
that they want to see evidence of me
knowing certain technology how about I
include that information and not talk
about something irrelevant so so that is
the secret sauce really now just reading
the the job description and you know
showing a bit of empathy and and trying
to to spend time and in helping the
person that might know nothing about the
technology to read and through the city
in our case we can spend a lot of
technical time
going through them but in many
organizations you will have either a HR
person or a I don't know broker that has
nothing that knows nothing about the
technology and they are just matching
keywords so if you don't do yourself a
favor and actually read
the job description and then include
appropriate evidence then you have no
chance of going through that Festival
but then if when you get through the
this this first round there's the second
round of uh for example selecting like
the the best ten percent or something so
if for example you have a lot of resume
and CV that includes the skills you are
looking for is there any projects or or
academics level that are more
interesting to others like for example
is it fine if his experience is all into
kaggle different kaggle competitions or
are you looking for someone that built a
startup or sure or push something online
so so I actually and I think we'll talk
about it later because we've talked
about it earlier but like and I at some
point and and really we should spend
some time about it like building AI in
AI systems
is not
it's not a trivial task and they vary we
I think I refer to it as almost like
building cars
and and you there are no people people
that know how to build cars don't exist
that's just not possible a car is a
super complicated end-to-end system
composed of countless different
components and you typically need a
fairly large collection of Specialists
so I I don't think I have ever hired a
person that knows machine learning AI
I'm typically looking much narrower than
that and so a person that understands
inference
a person that understands automatic
speech recognition models a person that
understands to an extent natural
language processing
a person that shows evidence of
experience in deploying computer vision
pipelines into production a person that
understands embedded systems
cargo is maybe an Evidence of a person
knowing to an extent traditional machine
learning because in kaggle there are
very rarely neural network based
competitions most people really want to
basically learn to do everything so it's
not possible so yeah so be more
generalist but you you you would advise
to rather focus on something which will
maybe help you build a stronger or
stronger portfolio for a very specific
job is that possible to know everything
it's just not like
there are there exists this small subset
of people that seem to have superhuman
capability and consumer information much
faster than and everyone else and and
they those people can know a bit more
yes but it's just not possible I'm very
I'm trying to focus right now on two
things namely scaling of natural
language processing
pipelines and and inference so
optimizing models for production and and
I I don't claim to be
keeping up to up to date with the
literature because between reading I
have to do also other things like help
customers resolve issues and and so it's
just physically not possible yeah
obviously some high level of general
knowledge helps what is it like for for
example if someone gets through the
first steps and starts the actual
interviews what what does it look like
how many interviews or what the not
really the questions but how what is the
shape of the interview and and the
format of the interviews
sure so every organization and more
frequently
even a group in the organization will
have their own way depending on their
own needs and capabilities and and
bandwidth so I cannot comment for NVIDIA
I cannot even comment for my broader
team I can tell you how I do it yeah yes
so I tend to be quite empathic and I
start by reading the CV yes
and in the same way as I would like them
to read the job description I read the
CV and I typically just ask them about
the things that they have written in the
and make sure that they actually
understand them because you know what's
the point of asking them
about something that is not listed that
I would hope that CV has enough you know
knowledge there already for so that I I
believe that that person can do the job
and not sure and I'll just focus
typically on that and if there are
certain gaps between the CV and what I
need them to do
I'll also be focusing on that so if they
would have read the job description and
prepared to the interview by looking at
all of the things listed there they
should be able to have a very meaningful
conversation with me
so an ideal preparation for such an
interview would be to as you said look
description description yes read the
description and understand it but also
look further into the bits of the
description that you are not sure you
are skilled about
you know so let's say I'm looking for
someone that that understands I don't
inference process and I'm writing that I
need someone that will be among many
things that will be supporting customers
in a quantization our training
it's a huge chance that someone will ask
me about quantization over training it
might be a simple thing and I might
spend five minutes reading about it and
understand it very well and in fact it
is but if I don't even devote two
minutes to to even on High level
understand it it shows that you know
like it's also to some extent that I
don't care
um so I've seen that you wear a deep
learning architect at Nvidia that's
correct could you explain a bit what is
a deep learning architect and especially
what it what is it in your case because
I assume as with Charles title it may
vary depending on the companion
that's correct so so so at Nvidia I
think the the that means actually
something quite different to what it
means in many other companies so
solution Architects are part of
pre-sales organizations so their goal is
to support customers and Adoption of our
technology but Nvidia tends to focus on
things that are difficult
that's that's almost like one of the key
principles that drive the selection of
what technologies Nvidia is and it's not
developing as a consequence solution
Architects tends to be you know very
specialized in in
quite narrow in a narrow field so but
but the role is on high level quite
straightforward at this to explain we
effectively have
in contrast to research scientists that
understand you know one topic one very
narrow topic very well and our role is
to understand substantially broader set
of topics also relatively well but
obviously nowhere near as well as as
individual researchers and and help to
bring those all of those things together
because as I mentioned building of AI
applications is almost like designing
and Manufacturing cars a lot of pieces
that need to come together and an
architect is a person that can grasp
those pieces maybe not understand each
and every components to its finest
detail but grasp those pieces together
and bring them into a holistic
end-to-end working solution
and what pieces are you working on you
mentioned that you wear mainly working
on scaling but are there any other
pieces so that's my core area of
competence so you know a lot of people
throughout Europe if they need to to to
know something about that piece they
would reach out to me and I have other
colleagues that are specializing in in
other things as well that are my go-to
you know support people for example a
colleague of my Miriam she specializes
in Riva which is our platform for
conversational AI I I have quite a bit
of respect for dye for his systems
knowledge and and so on and so forth so
so I specialize in that but I support
broad range of of activities of
customers because especially when
customers are just starting the journey
a lot of things I can cover with my
knowledge so you know stuff associated
with data preparation like enabling
establishing first pipelines the
development of first metrics and
kicking offers jobs monitoring their
performance doing error analysis
measuring efficiency with which they
execute first deployment into what will
become a production system and so so the
end-to-end process we try to support
customers some of them know all of that
already and then they have very specific
questions
and then we just work with engineering
to fix bugs that might be in the
software some of them don't and they
require more holistic support
so you mentioned customers I assume you
are part of a team at Nvidia that helps
with other companies asking for social
medias help using their corrector our
mission is to support the broader
community in adoption of AI Technologies
maybe the word customer is a bit
misleading because and and bbsl through
partners and you can buy our gpus from
AWS so micro
but yes our mission is to make sure that
the broad Community
can and adopts our Technologies and yes
that includes gpus but like like we are
more of a software
engineering company than a hardware
manufacturer uh the entire deep learning
stack is
is filled with Nvidia software from kudi
Anan from countless Fighters libraries
we're a major contributor to python
tensorflow
Triton infinite server tensor RT and
many many others
could you go over a bit more details
into one specific recent project that
you've had or had to help with
we have an upcoming conference called
the GTC it will be the 20th and there
will be two projects that I've been
supporting recently I will not be able
to go beyond what is already published
on the website I want to leave the
surprise
to the people that will actually be with
me presenting on the on the event but
like we have two talks one with juggle
and Rover and another one with Deutsche
Bank and and in both cases I was
supporting them in development of
natural language processing capability
for you know different use cases totally
different use cases totally different
sectors but the same technology stack
and same problems you know I have a
problem to solve
that problem is important to me you know
how do I how do I you know create a
training data set that is sufficiently
uh big to allow us to achieve our goals
you know how do I scale the training
process once I've succeeded and I've
reached my metrics how do I now provide
this user to a large group of people and
so you know some fairly standard yet not
trivial to solve problems like you know
GP utilization making sure that it's
high reaching latency targets when
executing those models scaling those
models so what if they you know the
demand from the users is Bumpy how do I
you know deploy dynamically a couple
additional gpus and then scale down all
of those problems are obviously solvable
but they require a lot of tools and a
lot of knowledge so we help and point
them into the right direction
and what's the for example you said that
the kind of the same technology were
applied very differently and the two
other two projects so what's the what
are the similar challenges on on both
projects but on all your projects is
there a recurrent challenge or something
right now you you know how to do but
it's like complicated for other people
to do and thus need your help
so most of the problems when you look at
them from again miles away they they
seem simple but it's really difficult is
the fact that life does not work like
that you don't get one thing instead you
get every single day
a medium scale problem to solve there's
just a lot of them and you know we we
want to label data how much data do we
need okay we need to establish that and
how exactly do we label let's define
that you know okay that's that's a lot
of data to label I cannot do it in one
evening so who exactly will label the
data okay I'm not labeling the data I
need to explain how exactly I want to
label to all of those people that I just
hired okay they've labeled some data how
do I know that they've labeled it in the
way that that is that that that is
appropriate yes okay I cannot do all of
that quality analysis I need to you know
maybe get a dedicated person to oversee
that process and you go through the
pipeline and all of those problems in
itself are not like rocket science but
all of them
needs to be solved and some of them
actually actually are surprisingly
tricky
and since you mentioned the working with
NLP mainly and NLP you are also working
with very large models and and various
data set as well so may I ask how the
different
not customers but the different people
that are working with you are dealing
with with these very large either model
and data sets so like a lot of of
compute as well I have a lot of space
required so what's your typical solution
for that or just like so how do you
press it process that so two years ago
like this would be a very difficult
conversation but yeah today tools just
exist
like you can go to to to to your
favorite search engine and and and
search for say Nemo Megatron and that's
a tool with which you can largely change
the language code
and I don't know you want to build a
model for Polish you change the language
code it will download a pile data set
you then click and if you have compute
that will train you a fairly decent
language model of almost any size you
want I think we've published hyper
parameters for models up to 175 billion
parameters and it will scale perfectly
as long as you have the right Hardware
so lead by perfectly I mean linearly so
so so that that's not a problem we have
published reference designs for how to
build systems that will skill linearly
we have this thing called superpot
reference architectures and many many
many people are reusing those also for
their own
system design so so we know exactly how
to how to train and and prompt tune or
adapter fine tune or
those models
the literature exists today this is not
as big of a challenge as you might think
and you know for an individual
the amount of hardware needed can be
scary if you think about oh how much my
car costs but but then you know a
typical large company
sometimes in a canteen will will spend
the same amount of money on sandwiches
in a year and what it takes to chain
those models and electricity so so those
not as as challenging problems as you
might think and there are many many
startups that have set up either
in-house or via some partners and a
large training systems that they use for
training for those jobs
so they're compared to the past the
current challenge is mainly to find
which tool to use and how to to
use them in a way that they would be
like cost effective rather than
developing something yourself
there are a lot of organizations that
are developing through themselves but if
you don't want to develop tools for
training large language models you don't
have to you just go to
Nemo websites you download the code
assets and you download the container
Docker container that packages
everything that you need including the
software you configure a single yaml
file to point it to
to where the data is located and choose
the size you you want and actually
preparing the data is always difficult
it's not as cheesy yes you can download
like file and train on that but you know
that model will not have all necessary
all the properties you want the systems
Hardware exists for training of those
models it's not that difficult to be one
of the big challenge is the people
they there aren't that many people that
actually know that those tools exist let
alone know how to use them or
have any hands-on experience using them
that's just a function of all of this
being very new very mind I think birth
paper and doesn't a large language model
that was published in what 2018 no end
of 2019 where we've shoved curves
demonstrating that larger NLP
architectures trained with larger models
on larger data sets
improving sample efficiency
so all of that is super new
so what would you well I assume this is
one of the things that you will talk in
the two events at GTC coming so people
can learn more about how to to scale NLP
models and and just to deploy them yeah
and we'll have dedicated talks devoted
to that as well so I think those talks
uh the two talks that I've mentioned are
predominantly focused on what
specifically Deutsche Bank and throw
that has right but we will have
dedicated stocks also focusing on
um on on large language models from
various different perspectives some
Hardware to software and we have this
thing called LM service which is like
hosted large language model so it would
be all sorts yeah perfect and so if if
for for the audience if you are
interested in learning more about what
we've just discussed there's the the
toes will be in the description below so
you and it's as as Adam said it's
completely free and it's during yeah
event coming so just to to go a bit more
into the your your Nvidia work I would
just like to ask a very basic question
that is what what is your day-to-day
life at Nvidia what are you you're doing
on a regular basis
our job is is quite flexible yes and and
really changes with uh with not only the
technology landscape but also with where
our customers are so when I've joined in
2017 my job was dramatically different
to what it is right now yes so in 2017
they weren't there wasn't that much of
an adoption of deep neural networks we
had some you know selected customers
that we've been supporting on a
day-to-day basis we've been supporting a
lot of academic and business events as
preparing talks and doing evangelization
and and and now it's it's dramatically
different right now a lot of those early
customers matured we have like very
large inference deployments so I
personally have couple customers that
will have multiple thousands of of
instances of Triton infinite server we
have regular engineering calls during
which they ask questions ask for
features and erase bugs and I have to
work with product management to
prioritize and resolve them and we we
definitely have now less less of a
presence on those like business focused
AI events because there is less need to
do that and I don't think I have a a a
super strict daily routine yes I have a
schedule of engineering calls with a
fairly large number of organizations
it's like every week maybe every two
weeks maybe every month depending on the
pace of that progress I'll dial in we'll
have a conversation about progress they
made issues challenges maybe bugs I
would then try to resolve some of myself
I would then pass some on to engineering
and I support development of proposals
just day-to-day conversations with
customers and answering the deeply
technical questions around
details so yeah things such as sizing
scaling how many gpus do we need to
train a model of this size on this data
set or how many do we need to to to to
be able to work with a team of six to to
do I don't know prompt learning or
adapter tuning on working towards this
problem
um and then it changes throughout the
year as well so closer to events like
GTC we we focus slightly more on the
content and sometimes prior to GTC we we
support development of demos
and and we have some customers that we
work very closely with Lighthouse
account customers that we support more
intensity so for example I just
published a paper with a company called
instadeep on nucleotide Transformers I
don't I know very little about biology
yeah admittedly but I do know how to
force neural networks to scale well so I
was you know Hands-On helping them to
make sure that they're very large
language model works very well on
proteins
so um so so
there isn't a single recipe that I
follow on a day-to-day basis yeah super
interesting it seems like for all the
interviews that I had recently with
people working at Nvidia you have a very
broad range of of projects that you can
participate in and learn from so it
seems really cool
yeah Nvidia is trying through their
culture to be very agile and and quickly
adapt to this admittedly insane
technological landscape
and would you say that is that that this
technology is more insane now than it
was like in 2018 when you first joined
and dramatically dramatically more so I
have like almost every day or every year
I say the same thing I've never seen
such a fast rate of progress yeah it's
unbelievable like if you would take I
don't know what was published right now
on those instruction tuned models
and tickets to an academic conference
two or three years ago
they would refer you to a mental
institution
I don't think anyone would have believed
that in such a short period of time
those model would exhibits those types
of behavior especially that in in most
cases we are not necessarily explicitly
training them to exhibit those behaviors
those are emerging features most of
those models are just trying to predict
the next token yeah it's indeed crazy
and how do you keep up with with this
rate of progress I don't I don't think I
do yeah so I have my
day-to-day job I do a bit of reading
based on we have an amazing research
team and and and we have in internal
email and other newsletters where people
just erase the most important stuff so
they do an amazing job
but I I don't I don't like it's not
possible so not even across entire AI
but even right now within natural
language processing it's just borderline
impossible to keep up with everything
and you have to learn to let go and
focus on what is it that you're doing
what are the problems that you have in
front of you solve them and and try to
add value like that
so as the field is maturing like this or
just getting crazier would you say that
you have to be more and more specific on
what you are doing right compared to the
to five or six years ago oh definitely
so we had that conversation with some of
my colleagues I don't remember exactly
one doesn't matter I guess so when I
joined Nvidia in 2017 it was somehow
possible yet already challenging for me
to grasp everything AI Nvidia right now
it's just not
not practical not not possible
that's too much would you say is that
it's more challenging now compared to
then when you you know for example it's
different it's definitely different
challenges back then you had to learn a
lot about
everything basically or like you
it was difficult to know what to learn
about just because you you have it's not
as broad so you can allow yourself to
learn about a bit everything comparative
versus now you you have to learn a lot
about a very specific thing and
that yeah as I said the challenges are
are very different would you say that
it's harder now or harder than with the
different challenges and different
things to to do I'm sure harder right
at that point of time it was also quite
hard because yeah a lot of things were
super non-obvious ly
and right now there is just a lot of
very good quality research and
Engineering coming out and so so I just
you have to learn to let go just it's
okay you cannot know everything I I at
some point I was trying to keep up with
both natural language processing and
computer vision research but I I think I
had to let go of computer vision I know
obviously that Transformer architectures
are very popular on occasional skin for
a paper to make sure that I more or less
know how to understand them but if you
were and I look at multimodal
architectures now a lot so that helps me
helps me be somehow up to speed and
unsupervised models but if you were to
ask me what is right now the best
architecture for I don't know object
detection on on Ms cook I I don't know
yeah you can ask strategypt so that's
yeah I can go to Google it doesn't
matter it's just I don't know from the
top of my head you have to let go
there's too much
yeah it's even
this thing is even stronger now when
Google first came up it's it's already a
different mindset of instead of trying
to gather knowledge in your own mind you
just need to understand how to find the
knowledge because Google has everything
accessible from from your fingertips but
now it's it's even more towards that
that way of just basically you need to
to know how to type to charge GPT or
whatever machine learning model that
will give you that the answer if it's
not hallucinating but that's another
thing but like it's yeah it's
what I think is that it's very dangerous
for our own memory just because we don't
have to know
as many things nearly as many things as
we did in the past and so maybe our
memory will just enter fire
or something but that's no it's still
useful to to memorize that it's just you
cannot just rely on once you know you
have expertise come from the fact that
you can bring together facts and combine
them together to like create new
insights
for your current project which is
something that I think a lot of people
are interested in too scaling and
natural language processing sure what
what is your well this question has
twofolds the first one is what is your
favorite tools to use and the second one
is what is your Tech stack
programming language and other like
internal tools you are using
so the tools that we're using are open
sources and I use almost exclusively
those so on a day-to-day basis when it
comes to NLP and I do other stuff as
well don't get me wrong
we use Nemo
uh I I use Nemo for both natural
language processing but I work with some
ad quite a lot also in like this broader
conversation AI so I do support quite a
few customers with automatic speech
recognition as well and less so in text
to speech and and Nemo provides
foundations for for those models as well
for users that don't want to be exposed
to kind of the the the low level
elements of implementations of Nema we
also have this thing called Tau which
stands for
terrain adapt optimize
which effectively helps people with less
of model knowledge to just fine-tune
models on their own data sets and I so I
use I use I use Nemo and historically
Megatron LM also for a lot of natural
language processing work prior to the
the generative models and where we've
been working with smaller bird-like
architectures those were typically just
Standalone models that you can still
find on our deep learning examples git
Repository and on inference front I
predominantly if not exclusively work
with Titan inference server which is an
open source infinite server used by many
used by Microsoft integrated in teams
office
and with by American Express and and
many other organizations
um and it has a back end called faster
Transformer through which which is still
quite early but it it already Works
quite well in my opinion in which
through which you can integrate even the
largest of language models for for
serving so even if you have a model that
doesn't fit into a GPU a faster
Transformer has a tensor and pipeline
parallel implementations you can slice
the model in half either a vertically or
horizontally and and and serve it like
that but if it doesn't if it's so huge
that it doesn't fit into any whatever
many however many gpus you have an s78
you it also supports like multi-node
serving and it has a lot of tricks up
its sleep around
just optimizing Auto regressive
inference because models like GPT are
Auto aggressive meaning that you
generate one token at a time and then
you do a lot of forward passes so so
there's a need to do a bit of trickery
so that you don't compute the same thing
so over and over again
so so that's kind of that we were I work
mostly with pytorch that's just because
the tools are available for pytharch and
we I also work with tensor RT quite a
bit tensority is a is a tool that we
also provide to the community that takes
a neural network
that was trained in say pytorch or that
was exported into Onyx and it optimizes
it for deployment to a specific GPU so
it does things such as I don't know
Fusion of layers of kernels it does
post-training quantization and and quite
a few other things
and and that list I work a lot with Riva
reverse our stack for
for conversational AI so provides
pipelines for automatic speech
recognition text to speech and now also
has an early version of chatbot maker
and all of the satellite utilities
around the technologies that I just
mentioned
are you surprised by the fact that open
source Technologies are so powerful
like compared to Preparatory
Technologies are you is it something
that is like to me this is something
like that is kind of mind-blowing that
everyone can access that and even right
now build companies and make profit off
of tools that are that were developed
openly for for everyone
I'm not sure they have a very strong
opinion or ever thought about this
problem in a lot of detail you know
definitely for technologies that have a
lot of a big community and this is a
blessing yes yeah but at the same time
there are countless open source projects
that are just maintained by one person
and they have no chance of
competing with a dedicated Enterprise
solution
yeah I was asked I was asking that
because right now we are in a world
where for example there could be cha GPT
that is completely issued and built by a
specific company and so you have to pay
and you cannot really do well you can do
a lot with it but you cannot really work
with its inner working and and it modify
it whereas for example there's the
stability AI with open source work and
like I feel like
just before stable diffusion the most
open source projects where kind of
not a pale copy but where under the
Preparatory Technologies most times and
I I'm feeling like there's a
turn around now where open source
Technologies is becoming
closer and closer to companies or even
more powerful now is that true like
there are plenty of Apache foundations
projects that are just at the foundation
of the internet you know that you know
that Linux is open so since yeah
so I you know they always played it all
but there is always also a role for
commercial products so so open source is
not necessarily cheap you know that no
of course let's say you have a small
company you take an open source project
and something doesn't work and your only
person that understands that project
cannot solve it
what do you do you ask politely to the
community and they maybe do or do not
help you right that's not that's not a
way to do business so there is obviously
value for many other companies to you
know provide Commercial Services around
US Open Source projects provide
Alternatives even Nvidia we have this
thing called Nvidia AI Enterprise
through which we provide support
for you know people that want it to all
of the open source tools like you know
if you find the bug in pytharch we'll
fix it yes for you and so so there is a
value in those and and and if you ever
would like I I participated in
deployment of production systems so
if stuff goes wrong you want to fix it
and I just wanted as my similar to my
other questions what what is the
the biggest challenge or the recurrent
challenge in just deploying models is
there is it something that is more
difficult than other stuff so you never
deploy models
you deploy pipelines yeah like I I don't
think I've ever participated in a
project where you deployed a model the
the challenges that you deploy really a
lot of different things that need to
work together and that all have
different properties so I don't know in
computer vision uh let's say you get a
video stream first thing you have to do
is to decode the Stream
and the coding in itself is super
non-trivial you have to think how you do
it if you just do it with some random
library on a CPU that would just be
maxing out all of the servers and you
will be not making much money but you
know if you choose the right codec
Nvidia gpus have Hardware acceleration
for video decoding and suddenly that
becomes free
but then next step you want to do some
pre-processing let's say you've used
Nvidia GPU for decoding yes so so you
want to use a library that does
something then I don't know trims the
trims the the image doesn't really
matter but you choose to use a CPU a
library because you your team knows it
that has implications you have to make a
memory copy from the GPU memory over PCI
Express to the host and you're putting
load so you you so in most day-to-day
life you don't deploy a model but a
quite complicated multi-stage pipeline
that's typically is composed of
substantially more than one neural
network yeah
and even in computer vision so you might
first of all do I don't know
identification of regions of interest
and classify initially those regions of
interest and then pass on those regions
to individual
inevitables that do different things I
don't know
in the shop setting and theft detection
vandalism detection or analytics you
want to have a shared decode because for
every one of those use cases you don't
want to decode the video stream over and
over again and then you do like common
detection of locations and maybe couple
other neural networks and then you have
another neural network that does
tracking and then you might know our
Network plus a classical tracking
algorithm classical tracking algorithm
because it's cheap
and neural network because if someone
goes to the toilet he wants to be able
to re-identify them so for
identification you'll have a second
neural network so suddenly what was just
oh I'll deploy whatever mask rcnn it
ended up in being this as quite a big
monstrosity of components and all of
them need to work all of them need to
scale
video analytics it's easy because the
workload is like clockwork 30 frames per
second 30 frames per second yes but in
many cases it's not so your customers
come in waves at Christmas
and then they don't come at all on New
New Year's Eve and and you don't want to
be paying for the harder it's such a
multi-dimensional problem
inference requires quite a lot of work
quite a lot of people with quite a lot
of different expertise
so the main challenges come with the the
complexity of the the solution as well
as the complexity of depression and
Randomness behind it it's just life like
life is never that easy like even things
that seem super trivial when you look at
them from from 100 miles away when you
start digging into the detail have a
level of complexity that level of
complexity might not be necessarily high
like neither of the things that I just
mentioned it's rocket science but it's
just a lot of it and you have to
systematically tackle every one of those
problems and that requires a bit of
patience a bit of
character sometimes and and you know
especially if you have a younger thing
without experience of doing it earlier
in quite a bit of
time that and this is kind of the the
the support we provide we we have a very
capable team that have has done those
things quite a few times and this is the
type of guy evidence we get them
so my my fourth question was about the
the two topics you we discussed that you
will be giving at and at GTC sure and so
is there anything else you wanted to
mention about those topics to or just at
least maybe summarize why should people
tune in to those two specific tasks that
you are giving at GTC so so
if you amplify emphasize with what I
just said yes around just day-to-day
complexity of solving problems those
talks will be great for you because
you'll hear two different groups that
are serving two dramatically different
problems talk about more or less the
same pain points pain points of you know
getting the first pipeline up and
running writing kpis
and you you'll get their point of view
of how to solve those problems and
hopefully it will help you plan your uh
your projects a bit better and and give
you some you know ideas on you know what
what things to put in place and in which
order because I think both of those
stocks are are organized chronologically
in a sense that they just go through the
Journey
from the very beginning to the very end
highlighting all of the key things that
caught them by surprise they also go
into motivation in quite a bit of detail
so why did those companies those
particular groups decided to embark on
that journey and that's also something
that might help you maybe if you're not
in an engineering role but moreover in a
management
to to to help you think more
systematically about what is it that
it's possible with natural language
processing
so very uh we should expect very
applicable tips from real world examples
basically yeah they will they will they
will they will people will
obviously have different experiences but
awesome well thank you very much for
your time and for all the very valuable
insights it was really interesting and I
learned a lot just in this past hour or
so so thank you very much for your time
again and I was glad to have you on on
this interview
thank you Candy and have a great day you
[Music]
foreign
Listen to the interview on your favorite streaming platforms like Spotify, Apple podcasts, or YouTube.
The lead image was generated using HackerNoon's Stable Diffusion AI Image Generator feature, via the prompt "a human architect standing and looking at a building".
Comments
loading...Recommend
About Joyk
Aggregate valuable and interesting links.
Joyk means Joy of geeK