

Build your own ChatGPT starter kit
source link: https://dev.to/bdougieyo/build-your-own-chatgpt-starter-kit-41gm
Go to the source link to view the article. You can view the picture content, updated content and better typesetting reading experience. If the link is broken, please click the button below to view the snapshot at that time.
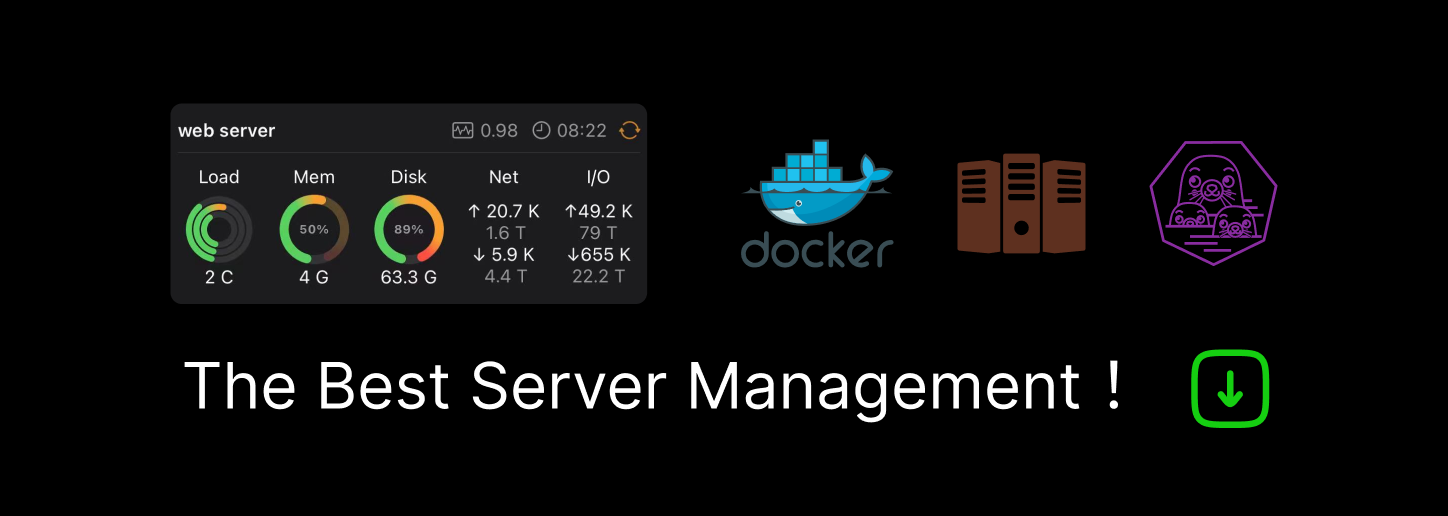
ChatGPT is an excellent general-purpose example of how we can use AI to answer casual questions, but it could do better when the questions require domain-specific knowledge. Thanks to this ChatGPT starter kit, you can train the model on websites you define.
header image was generated using midjourney
gannonh
/
gpt3.5-turbo-pgvector
ChatGTP (gpt3.5-turbo) starter app
What is gannonh/gpt3.5-turbo-pgvector?
This starter app was put together by @gannonh and makes great use of the Supabase pgvectors and OpenAI Embedding feature. The app leverages Next.js to stand up a simple prompt interface.
Live demo: https://astro-labs.app/docs
How does it work?
This starter app uses embeddings to generate a vector representation of a document and then uses vector search to find the most similar documents to the query. The results of the vector search are then used to construct a prompt for GPT-3, which is then used to generate a response. The response is then streamed to the user.
Web pages are scraped, stripped to plain text, and split into 1000-character documents.
// Stripe text from HTML
// pages/api/generate-embeddings.ts
async function getDocuments(urls: string[]) {
const documents = [];
for (const url of urls) {
const response = await fetch(URL);
const html = await response.text();
const $ = cheerio.load(html);
// tag based e.g. <main>
const articleText = $("body").text();
// class based e.g. <div class="docs-content">
// const articleText = $(".docs-content").text();
let start = 0;
while (start < articleText.length) {
const end = start + docSize;
const chunk = articleText.slice(start, end);
documents.push({ url, body: chunk });
start = end;
}
}
return documents;
}
Once the URLs are stripped down to the text, they are sent to the Supabase after some embedding creation using the text-embedding-ada-002
model.
The OpenAI docs recommend using text-embedding-ada-002 for nearly all use cases. Fun fact, this is the same embedding Notion's AI tool uses under the hood. It's better, cheaper, and simpler to use.
text-embedding-ada-002 announcement
// Create embeddings from URLs
// pages/api/generate-embeddings.ts
const documents = await getDocuments(urls);
for (const {
url,
body
}
of documents) {
const input = body.replace(/\n/g, " ");
console.log("\nDocument length: \n", body.length);
console.log("\nURL: \n", url);
const embeddingResponse = await openAi.createEmbedding({
model: "text-embedding-ada-002",
input
});
console.log("\nembeddingResponse: \n", embeddingResponse);
const [{
embedding
}] = embeddingResponse.data.data;
// In production we should handle possible errors
await supabaseClient.from("documents").insert({
content: input,
embedding,
URL
});
}
gpt3.5-turbo-pgvector is an excellent starter for folks looking to try out OpenAI on their own data or sites. I see this being extremely useful in the documentation and now understand why OpenAI doesn't need to search in their docs (this is a joke, they should add search). Search in docs could be replaced by projects setting up their own embeddings.
Share in the comments if you have a use case for this.
Also, if you have a project leveraging OpenAI or similar, leave a link in the comments. I'd love to take a look and include it in my 30 days of OpenAI series.
Find more AI projects using OpenSauced
Stay saucy.
Recommend
About Joyk
Aggregate valuable and interesting links.
Joyk means Joy of geeK