

Drilling into Einstein GPT - is generative AI trustworthy enough for enterprise...
source link: https://diginomica.com/einstein-gpt-generative-ai-trustworthy-enterprise-use
Go to the source link to view the article. You can view the picture content, updated content and better typesetting reading experience. If the link is broken, please click the button below to view the snapshot at that time.
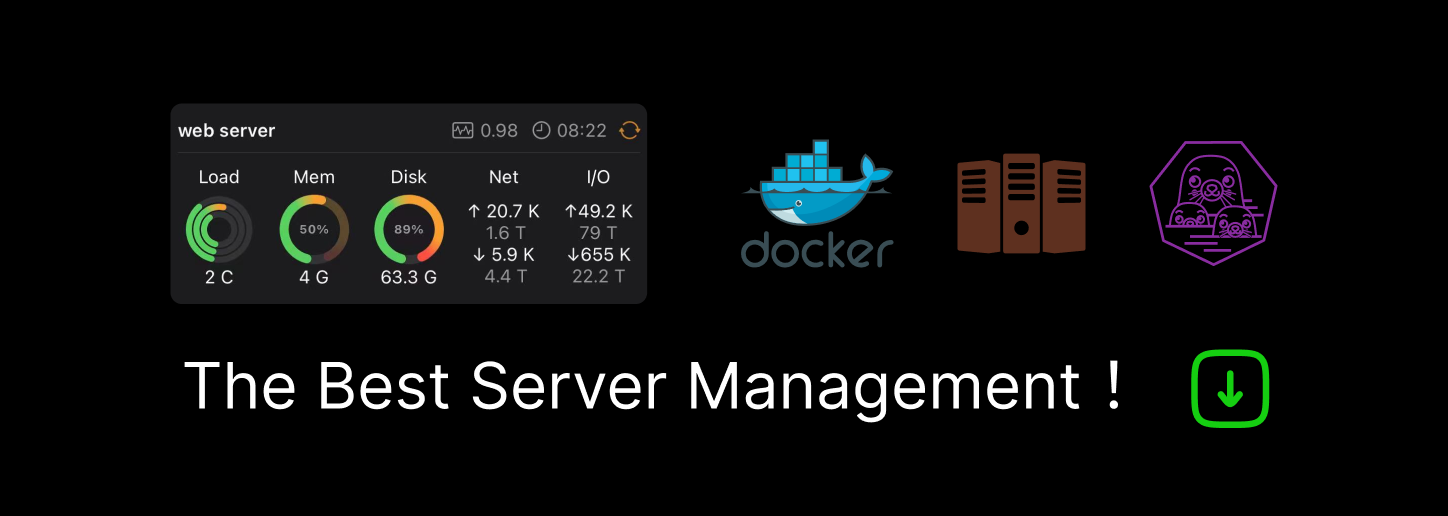
Drilling into Einstein GPT - is generative AI trustworthy enough for enterprise use cases?

Salesforce is making a big deal this week of building OpenAI's GPT3 technology — which powers ChatGPT — into a broad swathe of its products, describing its Einstein GPT offering as “the world’s first generative AI CRM technology.” But as I explored in an interview published yesterday with Emergence Capital's Jake Saper, there are big risks in using these Large Language Models (LLMs) in a business context. I spent the day investigating whether Salesforce is cognizant of those risks, and what steps it is taking to ensure its customers don't fall foul of them when implementing solutions based on Einstein GPT.
On the face of it, generative AI looks like it can bring a massive boost to business productivity, by making it easier to summarize information from unstructured data stored in documents, knowledgebases and message streams, preparing ready-made drafts for messages, emails and web content used in sales, service and marketing, or generating chunks of code and test routines for developers. But in more than twenty-five years of writing about and reporting on technology, I've seen enough to know that it's always sensible to look behind the hype and the enthusiastic demos to figure out what are the hidden downsides — where could it all go wrong?
In the case of generative AI, that skepticism is highly justified. As I sat through yesterday's keynote, I became more and more concerned. We saw Einstein GPT populate a Sales Cloud record with background information about a prospect, including information from external sources about a recent expansion into a new geography, which it then used to draft a message from the salesperson to the prospect. On the face of it, this seems like a huge timesaver. But how do we know that external source has all the facts right? What if the LLM has injected some made-up facts of its own? Sending that message without rigorous checking could do more harm than good.
Coming to the Service Cloud demo, we saw Einstein GPT generate an answer to a customer query based on a search of previous answers and knowledgebase entries. Again, this seems far better than the agent spending time tabbing through multiple different screens to manually compose that answer. But what if that answer is based on an out-of-date record or a previous conversation where the agent mistakenly gave bad advice? It's all very well to say that there's a human in the loop who msut check the suggested answer before sending it out, but where agents are rewarded for closing calls as quickly as possible, who's going to stop and check a ready-made answer that looks highly plausible? The checks and balances need to be built into the system, not left until you start to see your customer sat metrics sinking because they're being given poor advice.
The good news is that Salesforce is well aware of these pitfalls — it published its Guidelines for Trusted Generative AI just a month ago — and plans to build the necessary guiderails into its products. The bad news is that this may take a while and therefore you'll be waiting longer than you expect before Einstein GPT becomes generally available for use in production. And when it finally arrives, you may still have to spend more resource than you currently realize to make sure those guiderails are working effectively for your own use case.
So let's drill down into the various ways in which LLMs can go off the rails and what Salesforce is doing to mitigate the risks of Einstein GPT going rogue.
Risk #1 — the AI's source material is wrong
It's always been the case with computing that 'garbage in, garbage out,' and the only difference with generative AI is that it excels at making the garbage highly plausible. There's always a trade-off with generative AI models between creativity and safety, says Clara Shih, CEO of Sales Cloud. In consumer use cases, the emphasis typically skews towards creativity based on a wide cross-section of source material, whereas in business, it's crucial to constrain the source material to drastically reduce the risk of including false data or information. She adds:
Your customer service agent in crafting her email response to the customer's email doesn't have to be the most creative person in the entire world. You really want her to be very focused on the approved knowledge articles and product documentation and safety documentation ...
It's all about grounding [and] training the generative AI models in the knowledge articles, product documentation, which has the truth about how the products are supposed to work, using that to generate the outputs.
Salesforce is talking up its Data Cloud as the main repository of a customer's information that Einstein GPT will draw on, ensuring that it's relying on a validated source. But that means it's incumbent on customers to ensure that the data housed there is clean. Jayesh Govindarajan, SVP of Engineering, Einstein and Bots, says:
With AI, the quality of data is really important to have the right level of output. That comes in the way we ground it, based on what we have done with Data Cloud ... It's up to the customer to ensure that the data is clean, harmonized [and] has good semantic meaning.
The model should also be able to learn from how users are responding to its output, but here again the onus falls back on the customer to validate what Einstein GPT is producing. Shih warns:
There's a human in the loop. The marketing manager doesn't get off the hook from being accountable for what's put in the marketing campaign.
Risk #2 — the AI makes stuff up
For reasons that are not fully understood at the moment, LLMs like ChatGPT are prone to invent facts to fit the narrative they're building, and can even become aggressively assertive at defending their inventions when challenged — an AI phenomenon known as hallucination. So as well as grounding their training sets in validated data sources, it's also important to build in mitigations against this anomalous behavior. Kathy Baxter, Principal Architect at Salesforce, says:
We know that generative AI can give inaccurate answers, it can hallucinate and be really confident about it. [We can mitigate this] by saying, focus on these 100 knowledge articles, and if you can't find the answer to the customer's question in these 100 knowledge articles, come back with the answer 'I don't know.' Don't go and make make something up ... There's going to be a number of different things that we'll be putting into place to help our customers or the end users, can they trust this response? Is this something that perhaps I should seek other sources before using this answer?
Other potential mitigations that Salesforce is looking at are including the source of the information in the answer, requiring the model to validate certain types of information from more than one source, or adding a confidence level that shows how sure the model is that it has found the right answer. Building these features and ensuring they work effectively is one of the reasons why Salesforce isn't yet putting a timeline onto when Einstein GPT will go into production for the main Sales, Service and Marketing clouds. It's going to take time to get them working well, but it's essential work. Baxter adds:
A lot of these things are things that we are prioritizing for our models, because we know how important that is. If you don't bake it in from the beginning, you're always playing catchup after that.
Risk #3 — the AI still occasionally gets things wrong
All of the work that Salesforce is doing to make Einstein GPT robust brings up another risk well-known to builders of AI systems — how to make sure that humans still catch the occasional errors that still come through? Think of autonomous driving, where most of the time it works fine, but the the driver's attention drifts off and then they're caught unawares when something happens that the AI can't handle. Baxter comments:
If the AI is right the majority of the time and only every so often it gets the answer wrong, this becomes a surveillance task. And humans are lousy about extended surveillance ... Humans, they get used to AI being so magical, it gets it right so often that you become complacent.
It's going to be a really interesting design challenge, as we add in these different, what we call mindful friction moments, to get humans to slow down, check the answers every single time, so that what they are getting really is the right answer, really is the best answer.
Risk #4 — users aren't empowered to train the AI
Companies who see Einstein GPT as a great opportunity to lower their costs by replacing humans with AI equivalents need to take into account the continuing need for human supervision to keep these systems honest. This goes back to the point made by Emergence Capital's Jake Saper in the interview published yesterday:
Deploying them on their own without a feedback loop, without contextually specific data, and without a human to help oversee and ensure accuracy, is likely to result in some some scary outcomes ... [I]f you use them without guardrails, bad things will happen.
A key part of Einstein GPT's advantage over generic LLMs is that its own users can provide feedback on the model's output, which then goes back into its reinforcement learning cycle. Govindarajan explains:
If the content is edited [by a human user] in some form, it's being edited on our platform, that's a signal. If the content is outright being rejected, and a completely new response is being written by a human in the loop, that's also feedback information. Or if content has been just accepted as is, that's the Holy Grail, you're starting to get more and more accurate.
But that doesn't happen unless the users are empowered and incentivized to take the time to give that feedback. Companies that simply use these technologies to cut back on staff and report faster case resolution times will inadvertently poison their own training sets by rewarding and reinforcing the AI's false answers.
Risk #5 — customers misuse the AI
Salesforce, along with other vendors adopting generative AI technologies, have a big customer education job ahead of them, to ensure that customers are aware of all of the pitfall mentioned above, and also to make sure that customers don't inadvertently — or even deliberately — use these systems in unacceptable ways. Paula Goldman, Chief Ethical and Humane Use Officer at Salesforce, says:
We not only need think carefully about what we're going to build, and then we build it very carefully. But then we have a set of policy guardrails for which there are consequences if you violate them.
The company runs 'consequence scanning' workshops to figure out the various ways in which use cases may go off the rails and what mitigations to put in place. She explains:
We try to imagine as much as possible, what are all of the ways that this technology can be used with malice, naivety or just orthogonal use cases ... So we really try to do a lot of consequence scanning and then mitigations for it, but then also working with pilot customers and others to get feedback.
My take
It looks like it's going to be a long road to put in place the various mitigations that will be needed to safely fulfil all the promise shown off in yesterday's keynote. As Govindarajan told me, while it's relatively easy to include citations, it may take a year or more to develop meaningful confidence scores. Pilots and beta testing with customers will be an important part of getting this right. He says:
We want to do this with care. We want to understand the bounds of the system. But we also know that the only way to learn is to get it out there.
But nevertheless he believes the overall impact on productivity will be worthwhile, even if users need vigilance in checking the output from the LLM. He says:
If you break your work down into going from an idea to a first draft, and then verifying the process of going from a draft to the final thing, if the verification usage is small, and the creation time is large, I think those tasks will be phenomenal fixes.
Overall I think that's true, but I suspect the impact will vary depending on the use case. Einstein GPT's ability to create snippets of code and test routines is a very strong use case, largely because programming languages are very clearly constrained sets of data so there's less scope for LLMs to introduce errors, while the users already have a lot of expertise in the language so can spot errors much more easily than in other use cases. Those parameters suggest that the productivity gains will be significant, freeing up developer time to focus on other work. But it's still going to be important for customers to build in processes to ensure that overlooked errors don't slip through into production code.
Slack users who opt into the beta of the ChatGPT app for Slack will get an early chance to experience some of the risks outlined above, as unlike yesterday's Einstein GPT announcements, this simply applies OpenAI's ChatGPT engine to a company's Slack messages (but not the contents of its Huddle chats, video Clips and any images, since Slack doesn't make transcripts of these and ChatGPT is text-only). Slack users at OpenAI who have already been using the integration say that it's useful for summarizing threads, searching for specific information across multiple threads, and composing drafts of messages. But the user base at OpenAI is clearly well versed in the limitations of the technology — others who adopt this integration should exercise due caution.
As for Einstein GPT when applied to the core Sales, Service and Marketing clouds, success will depend on the testing and guiderails that Salesforce can put in place over the coming months, along with the change management guidance it develops in collaboration with customers, based on their experience of making use of the technology. The upside potential is improved productivity and a higher quality customer experience. But if it goes wrong, the downside cost of a poor customer experience can be huge. Last night I asked Rick Nucci, CEO of Guru, which uses AI in the customer service realm, for his thoughts on yesterday's announcements, and his emailed response sums up the crucial balance that customers must weigh up:
The long-term success of products using GPT technology will ultimately come down to the level of the trust and accuracy of the content that’s produced. If a customer receives a response that’s inaccurate and low quality, they’ll leave that interaction unhappy — no matter how quickly the response came through.
Recommend
About Joyk
Aggregate valuable and interesting links.
Joyk means Joy of geeK